Hyperspectral 3D Point Cloud Segmentation Using RandLA-Net
Intelligent Autonomous Systems 17 Lecture Notes in Networks and Systems(2022)
摘要
Point clouds are commonly used in robotics to represent 3D maps. To gain further understanding of their content, it is useful to annotate such maps semantically. To segment 3D point clouds with RGB values, different solutions exist. In machine learning, pre-trained classifiers are used for this purpose. Since it is not always possible to differentiate between entities relying solely on RGB information, hyperspectral histograms can augment the 3D data. The aim of this work is to evaluate, if hyperspectral information can improve the segmentation results for ambiguous objects, e.g., streets, sidewalks, and cars using established deep learning methods. Given the reported performance on geometrical 3D data and the possibility to directly integrate point annotations, we extended the neural network RandLA-Net. In addition to the evaluation of RandLA-Net in this context, we also provide a reference dataset consisting of semantically annotated hyperspectral 3D point clouds.
更多查看译文
关键词
segmentation,3d,randla-net
AI 理解论文
溯源树
样例
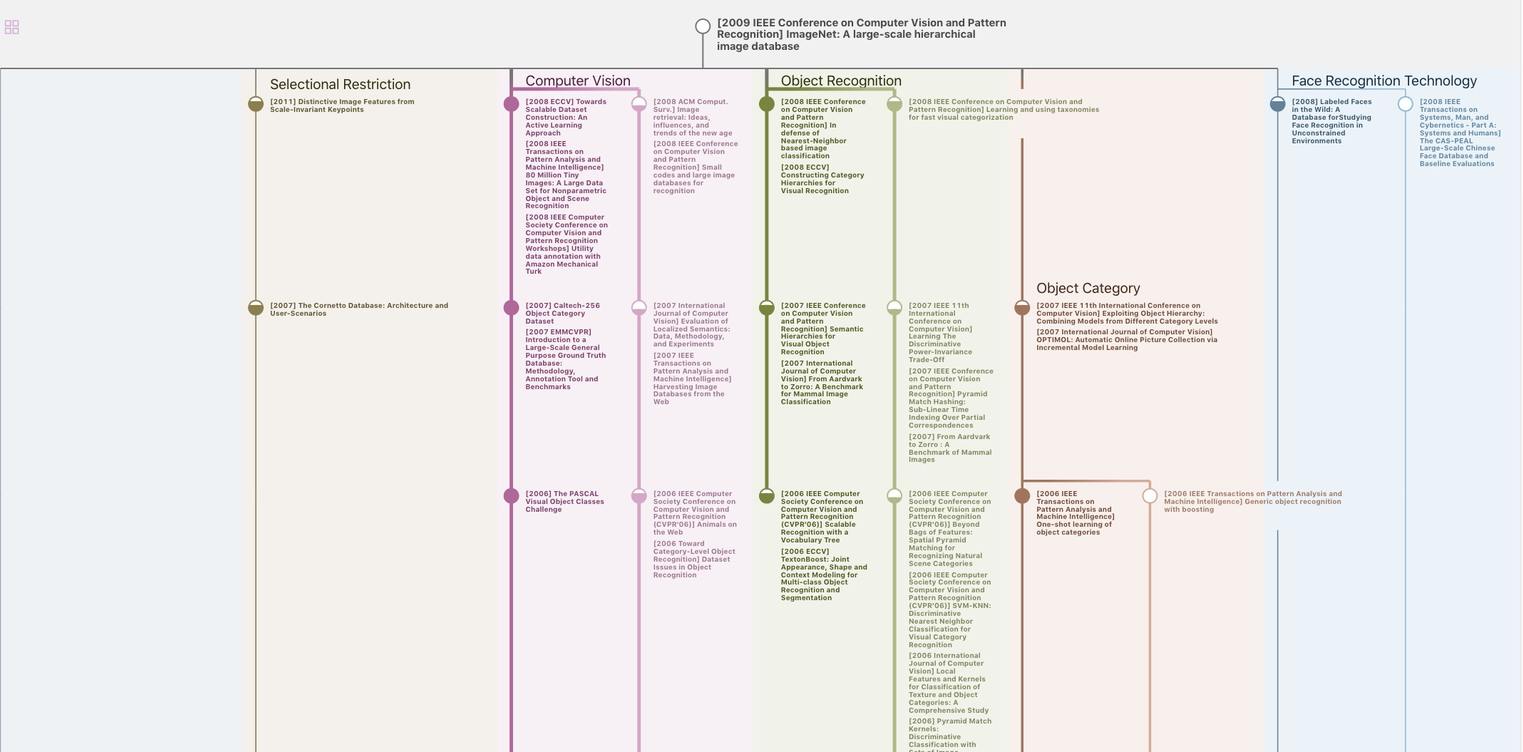
生成溯源树,研究论文发展脉络
Chat Paper
正在生成论文摘要