Algorithmic similarity depends continuously on the input distribution, not categorically on how inputs are generated.
Trends in cognitive sciences(2023)
摘要
I share Schyns and colleagues’ [ 1. Schyns P.G. et al. Degrees of algorithmic equivalence between the brain and its DNN models. Trends Cogn. Sci. 2022; 26: 1090-1102 Abstract Full Text Full Text PDF PubMed Scopus (6) Google Scholar ] interest in comparing algorithms between brains and machines, and I agree that generative models have an important place in interpreting algorithms. However, I think they err in claiming a categorical difference in comparing networks by their generative models versus by their input-output functions. Instead, these differences reflect a continuous spectrum of where the input-output functions’ similarities are evaluated. Crucially, misidentifying a spectrum of differences as a categorical difference loses sight of the fundamental challenge for intelligence: generalization to new things. Generalization itself is a graded concept, and algorithms can have different performances when tested on data that are independent and identically distributed from the same training data, weakly out of distribution (like for common corruption [ 2. Hendrycks D. Dietterich T. Benchmarking neural network robustness to common corruptions and perturbations. in: Proceedings of the International Conference on Learning Representations. 2019 Google Scholar ] or adversarial attack [ 3. Szegedy C. et al. Intriguing properties of neural networks. arXiv. 2013; (Published online December 21, 2013)https://doi.org/10.48550/arXiv.1312.6199 Google Scholar ]), or more strongly out of distribution (like for new poses, new objects, new attributes, or unnatural and supernormal stimuli).
更多查看译文
AI 理解论文
溯源树
样例
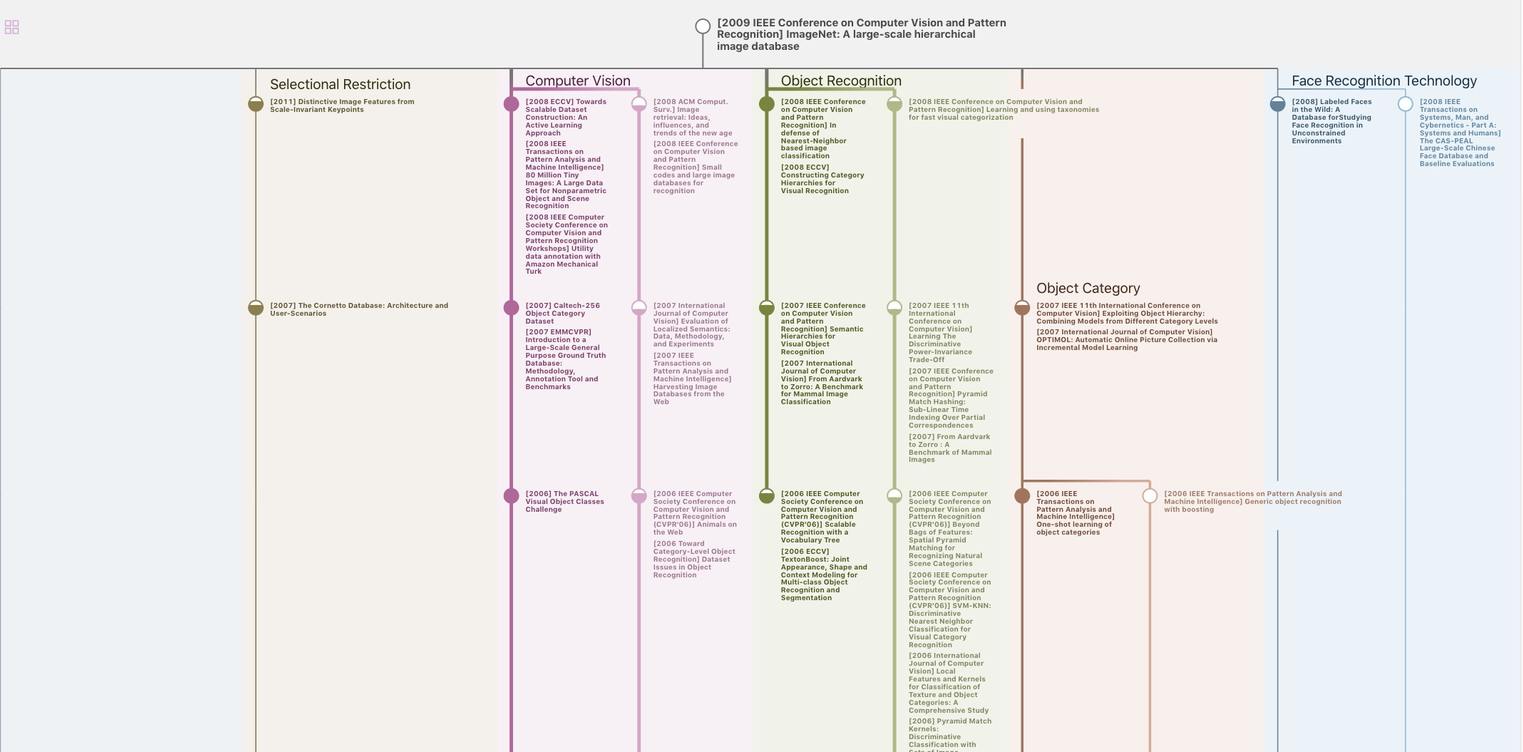
生成溯源树,研究论文发展脉络
Chat Paper
正在生成论文摘要