A Scalable Technique for Weak-Supervised Learning with Domain Constraints
arxiv(2023)
摘要
We propose a novel scalable end-to-end pipeline that uses symbolic domain knowledge as constraints for learning a neural network for classifying unlabeled data in a weak-supervised manner. Our approach is particularly well-suited for settings where the data consists of distinct groups (classes) that lends itself to clustering-friendly representation learning and the domain constraints can be reformulated for use of efficient mathematical optimization techniques by considering multiple training examples at once. We evaluate our approach on a variant of the MNIST image classification problem where a training example consists of image sequences and the sum of the numbers represented by the sequences, and show that our approach scales significantly better than previous approaches that rely on computing all constraint satisfying combinations for each training example.
更多查看译文
关键词
learning,scalable technique,constraints,weak-supervised
AI 理解论文
溯源树
样例
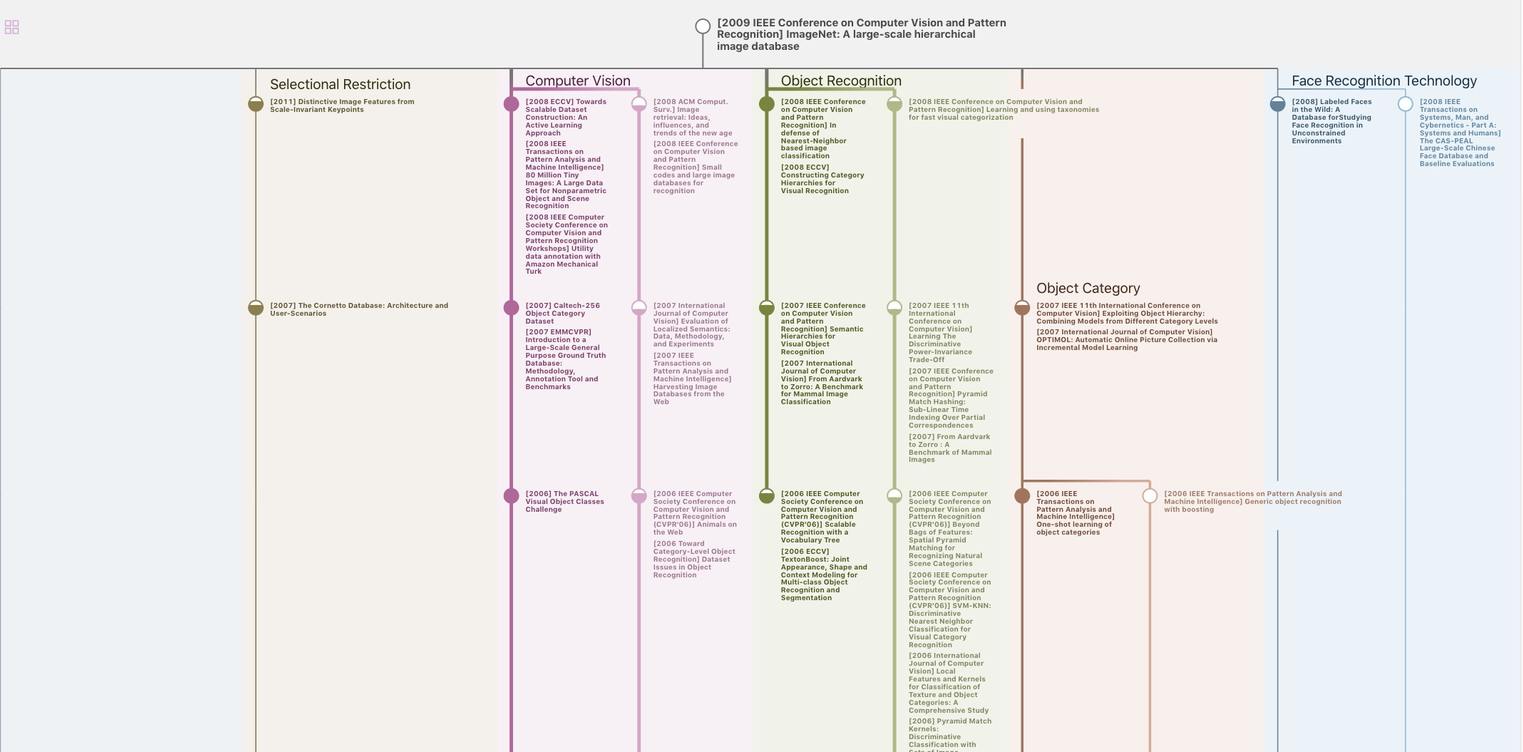
生成溯源树,研究论文发展脉络
Chat Paper
正在生成论文摘要