Parallel LSTM-CNN Network With Radar Multispectrogram for Human Activity Recognition
IEEE Sensors Journal(2023)
摘要
Owing to the ability of automatic feature extraction, deep learning is extensively applied in human activity recognition (HAR) based on radar. In this article, a recognition method based on a multispectrogram and deep-learning model is proposed. The radar echo data are transformed into spectrograms with different feature expressions by performing three time–frequency analyses, including short-time Fourier transform (STFT), reduced interference distribution with Hanning kernel (RIDHK), and smoothed pseudo-Wigner–Ville distribution (SPWVD), and then the spectrograms are sent to deep-learning model to realize HAR. The model employs three long short-term memory (LSTM) networks to learn the temporal features of the spectrograms and uses a 3-D convolutional neural network (3-DCNN) to extract the independent spatial features of a single spectrogram and the spatial correlation features between different kinds of spectrograms, named parallel LSTM-CNN network (PLCN). The PLCN is capable of effectively improving the degree of feature utilization in the spectrogram and the accuracy of human activity classification by fusing both temporal and spatial features. The final experimental results show that the average recognition accuracy of the method based on the PLCN for eight human activities reaches 94.75%, which is significantly higher than that of the three parallel LSTMs and the single 3-DCNN.
更多查看译文
关键词
Deep learning,human activity recognition (HAR),parallel LSTM-CNN network (PLCN),radar multispectrogram
AI 理解论文
溯源树
样例
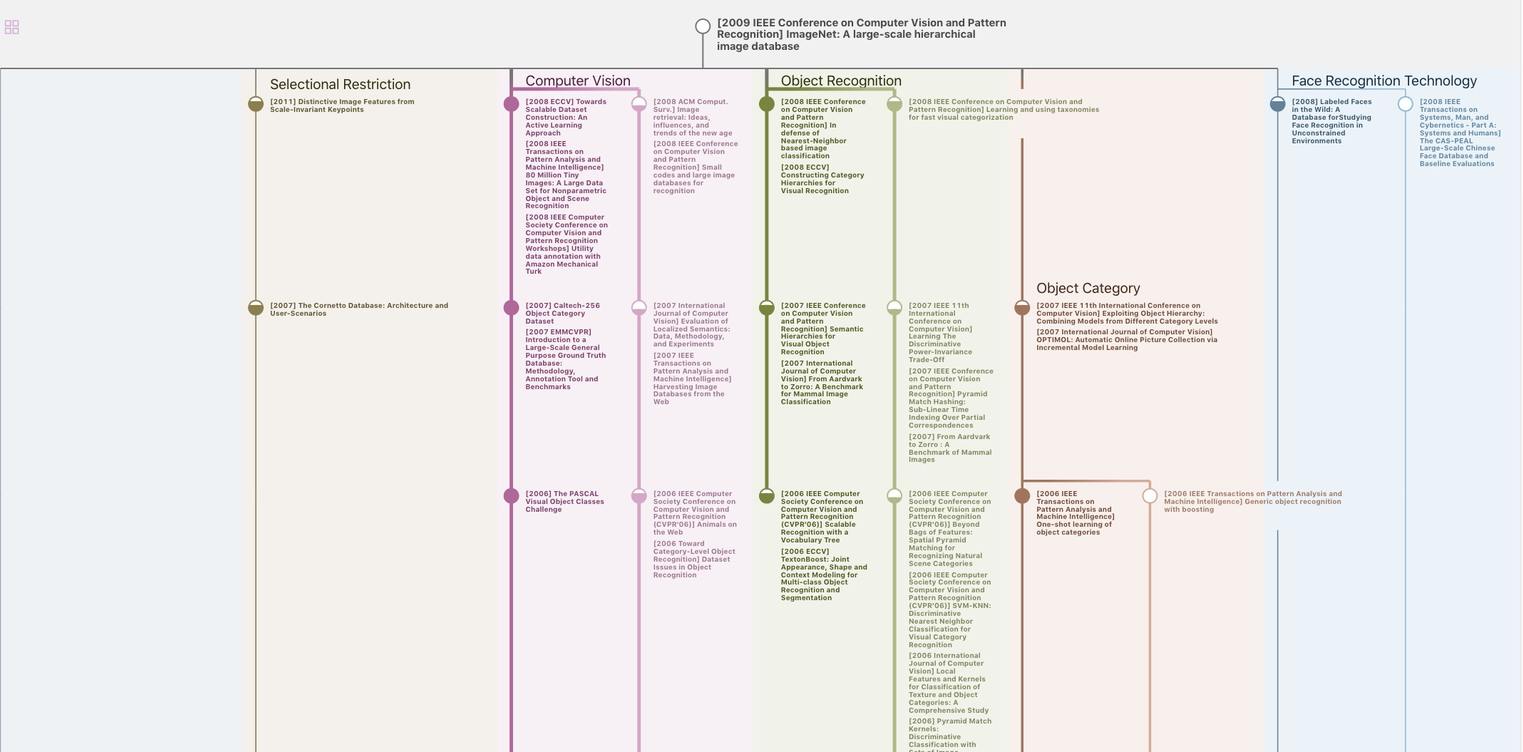
生成溯源树,研究论文发展脉络
Chat Paper
正在生成论文摘要