Bilateral‐Weighted Online Adaptive Isolation Forest for anomaly detection in streaming data
Statistical Analysis and Data Mining: The ASA Data Science Journal(2023)
摘要
We propose a method called Bilateral-Weighted Online Adaptive Isolation Forest (BWOAIF) for unsupervised anomaly detection based on Isolation Forest (IF), which is applicable to streaming data and able to cope with concept drift. Similar to IF, the proposed method has only few hyperparameters whose effect on the performance are easy to interpret by human intuition and therefore easy to tune. BWOAIF ingests data and classifies it as normal or anomalous, and simultaneously adapts its classifier by removing old trees as well as by creating new ones. We show that BWOAIF adapts gradually to slow concept drifts, and, at the same time, it is able to adapt fast to sudden changes of the data distribution. Numerical results show the efficacy of the proposed algorithm and its ability to learn different classes of concept drifts, such as slow/fast concept shift, concept split, concept appearance, and concept disappearance.
更多查看译文
关键词
anomaly detection,bilateral weighting,ensemble methods,isolation forest,online machine learning,outliers,stream processing
AI 理解论文
溯源树
样例
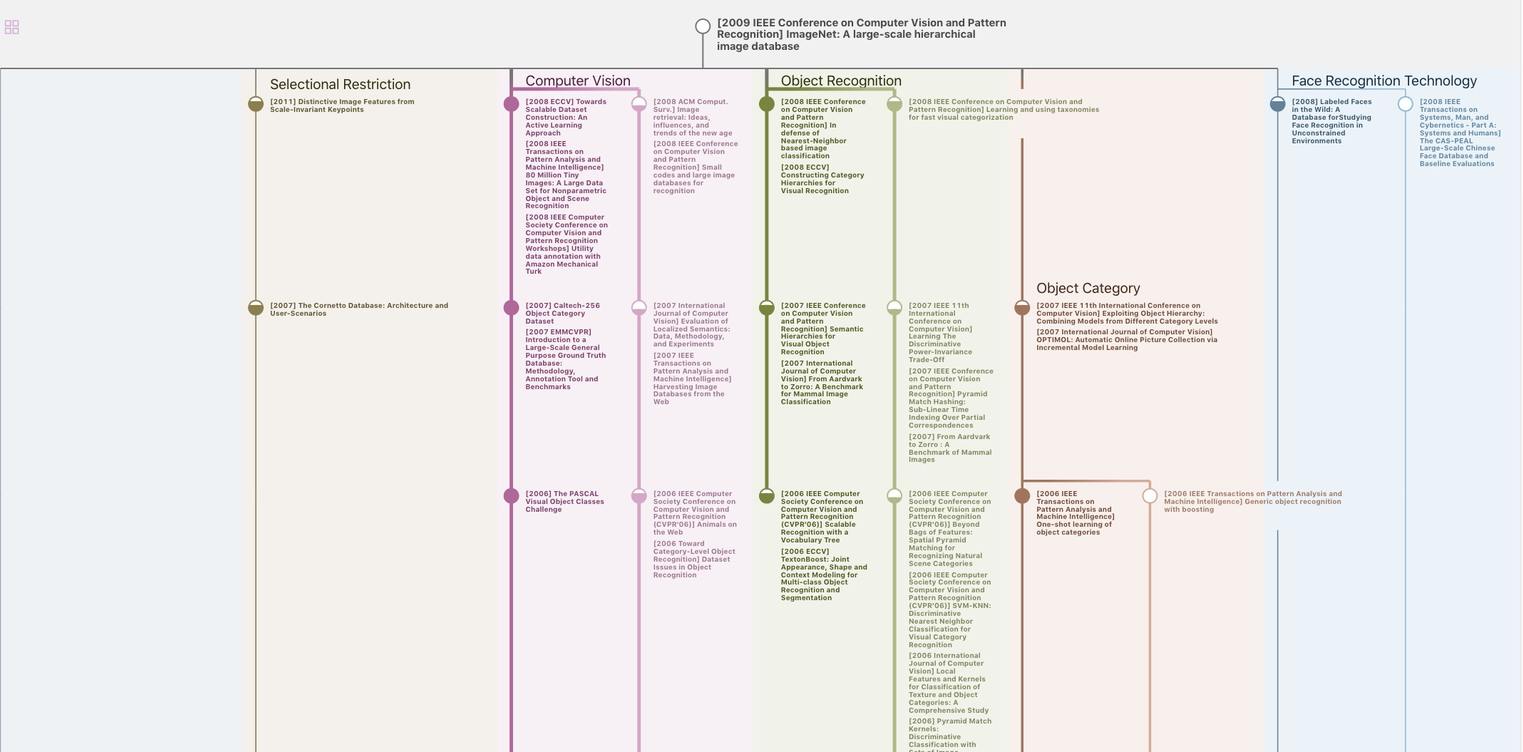
生成溯源树,研究论文发展脉络
Chat Paper
正在生成论文摘要