Predicting Structural Properties of Pure Silica Zeolites Using Deep Neural Network Potentials.
The journal of physical chemistry. C, Nanomaterials and interfaces(2023)
摘要
Machine learning potentials (MLPs) capable of accurately describing complex potential energy surfaces (PESs) have revolutionized the field of multiscale atomistic modeling. In this work, using an extensive density functional theory (DFT) data set (denoted as Si-ZEO22) consisting of 219 unique zeolite topologies (350,000 unique DFT calculations) found in the International Zeolite Association (IZA) database, we have trained a DeePMD-kit MLP to model the dynamics of silica frameworks. The performance of our model is evaluated by calculating various properties that probe the accuracy of the energy and force predictions. This MLP demonstrates impressive agreement with DFT for predicting zeolite structural properties, energy-volume trends, and phonon density of states. Furthermore, our model achieves reasonable predictions for stress-strain relationships without including DFT stress data during training. These results highlight the ability of MLPs to capture the flexibility of zeolite frameworks and motivate further MLP development for nanoporous materials with near- accuracy.
更多查看译文
关键词
pure silica zeolites,neural network,structural properties,potentials
AI 理解论文
溯源树
样例
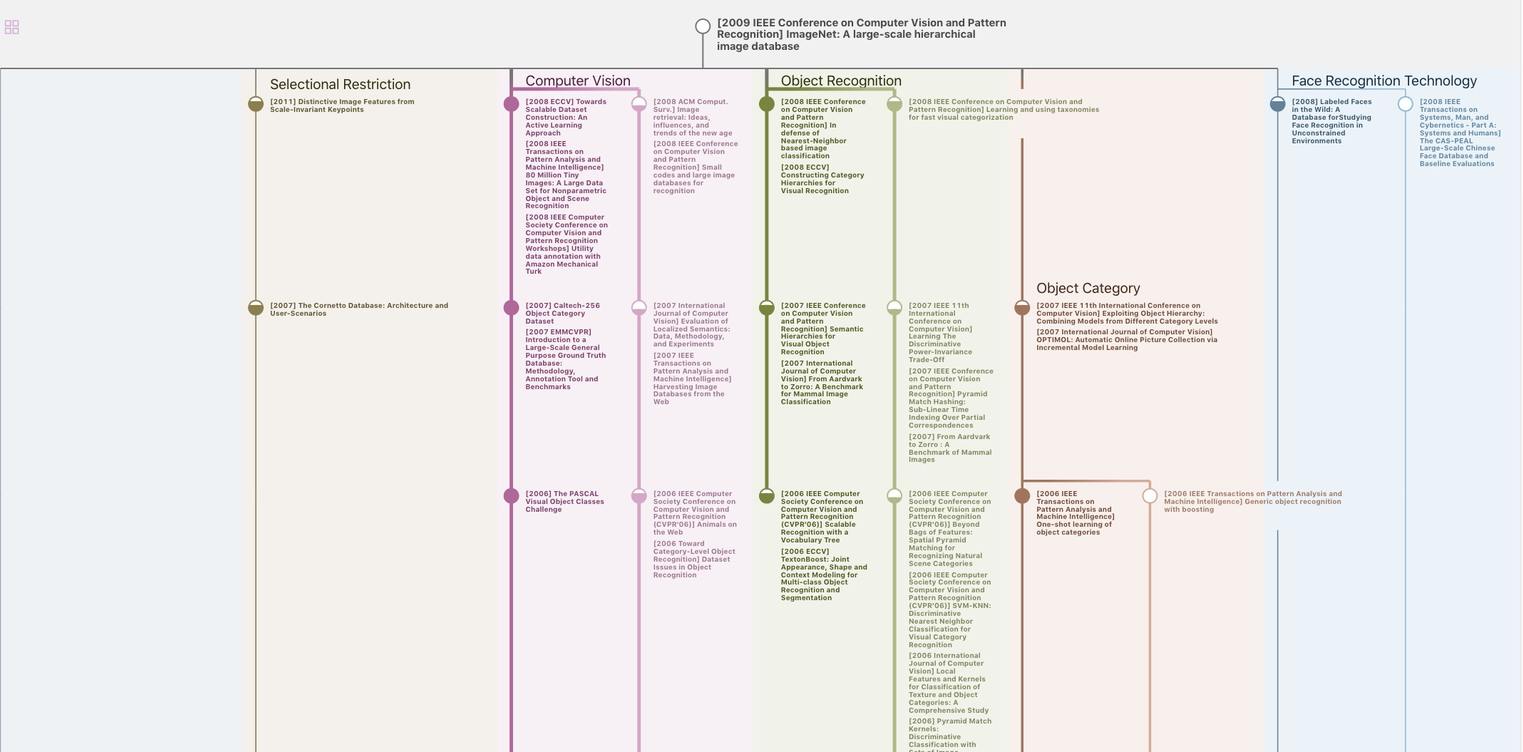
生成溯源树,研究论文发展脉络
Chat Paper
正在生成论文摘要