Topologically preserved registration of 3D CT images with deep networks.
Physics in medicine and biology(2023)
摘要
. Computed Tomography (CT) image registration makes fast and accurate imaging-based disease diagnosis possible. We aim to develop a framework which can perform accurate local registration of organs in 3D CT images while preserving the topology of transformation.. In this framework, the Faster R-CNN method is first used to detect local areas containing organs from fixed and moving images whose results are then registered with a weakly supervised deep neural network. In this network, a novel 3D channel coordinate attention (CA) module is introduced to reduce the loss of position information. The image edge loss and the organ labelling loss are used to weakly supervise the training process of our deep network, which enables the network learning to focus on registering organs and image structures. An intuitive inverse module is also used to reduce the folding of deformation field. More specifically, the folding is suppressed directly by simultaneously maximizing forward and backward registration accuracy in the image domain rather than indirectly by measuring the consistency of forward and inverse deformation fields as usual.. Our method achieves an average dice similarity coefficient (DSC) of 0.954 and an average Similarity (Sim) of 0.914 on publicly available liver datasets (LiTS for training and Sliver07 for testing) and achieves an average DSC of 0.914 and an average Sim of 0.947 on our home-built left ventricular myocardium (LVM) dataset.. Experimental results show that our proposed method can significantly improve the registration accuracy of organs such as the liver and LVM. Moreover, our inverse module can intuitively improve the inherent topological preservation of transformations.
更多查看译文
关键词
CT,attention,deep learning,medical image registration,uni-modal,weakly-supervised
AI 理解论文
溯源树
样例
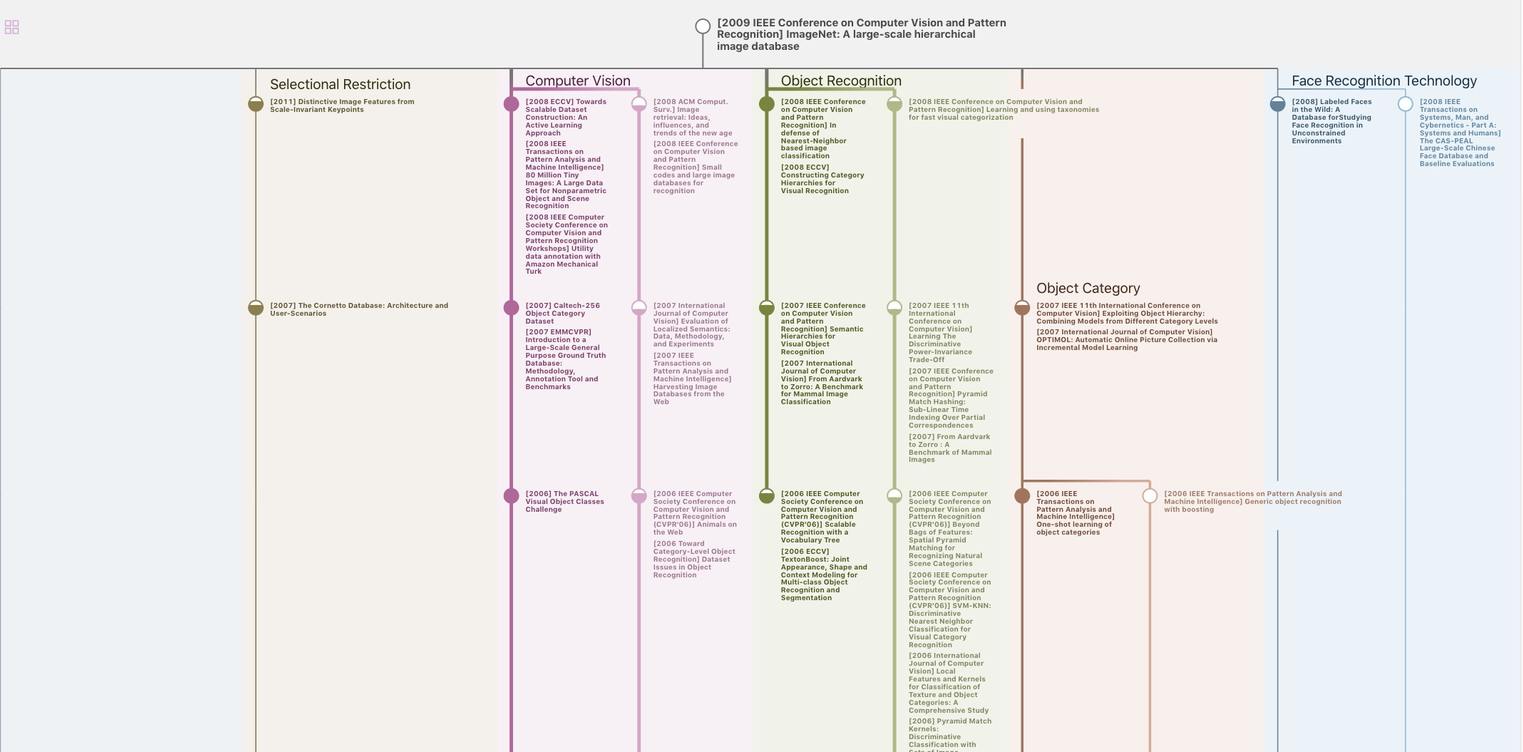
生成溯源树,研究论文发展脉络
Chat Paper
正在生成论文摘要