Adversarial Adaptation for French Named Entity Recognition
arxiv(2023)
摘要
Named Entity Recognition (NER) is the task of identifying and classifying named entities in large-scale texts into predefined classes. NER in French and other relatively limited-resource languages cannot always benefit from approaches proposed for languages like English due to a dearth of large, robust datasets. In this paper, we present our work that aims to mitigate the effects of this dearth of large, labeled datasets. We propose a Transformer-based NER approach for French, using adversarial adaptation to similar domain or general corpora to improve feature extraction and enable better generalization. Our approach allows learning better features using large-scale unlabeled corpora from the same domain or mixed domains to introduce more variations during training and reduce overfitting. Experimental results on three labeled datasets show that our adaptation framework outperforms the corresponding non-adaptive models for various combinations of Transformer models, source datasets, and target corpora. We also show that adversarial adaptation to large-scale unlabeled corpora can help mitigate the performance dip incurred on using Transformer models pre-trained on smaller corpora.
更多查看译文
关键词
adversarial adaptation,french,recognition
AI 理解论文
溯源树
样例
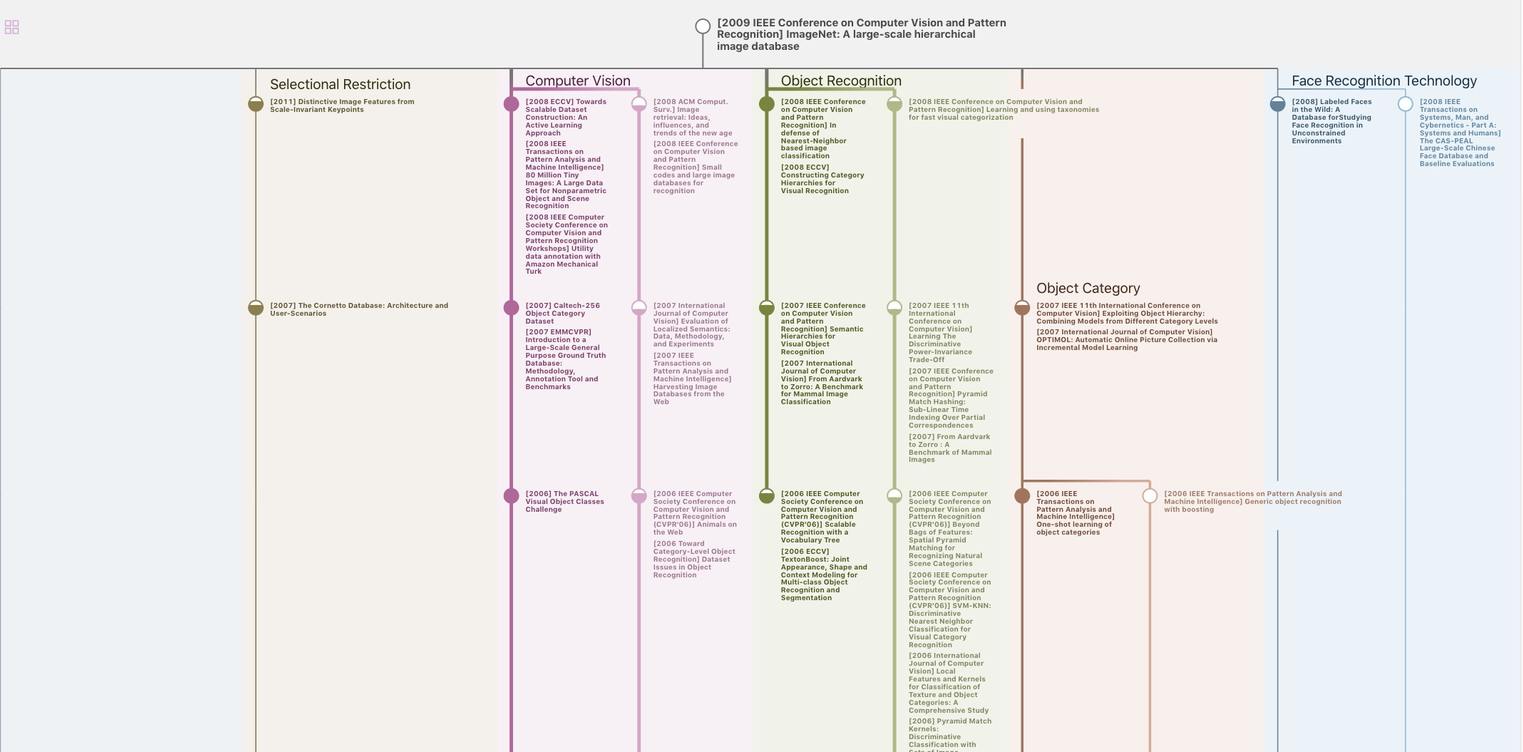
生成溯源树,研究论文发展脉络
Chat Paper
正在生成论文摘要