Modeling the evolution of temporal knowledge graphs with uncertainty
arxiv(2023)
摘要
Forecasting future events is a fundamental challenge for temporal knowledge graphs (tKG). As in real life predicting a mean function is most of the time not sufficient, but the question remains how confident can we be about our prediction? Thus, in this work, we will introduce a novel graph neural network architecture (WGP-NN) employing (weighted) Gaussian processes (GP) to jointly model the temporal evolution of the occurrence probability of events and their time-dependent uncertainty. Especially we employ Gaussian processes to model the uncertainty of future links by their ability to predict predictive variance. This is in contrast to existing works, which are only able to express uncertainties in the learned entity representations. Moreover, WGP-NN can model parameter-free complex temporal and structural dynamics of tKGs in continuous time. We further demonstrate the model's state-of-the-art performance on two real-world benchmark datasets.
更多查看译文
关键词
temporal knowledge graphs,uncertainty,modeling
AI 理解论文
溯源树
样例
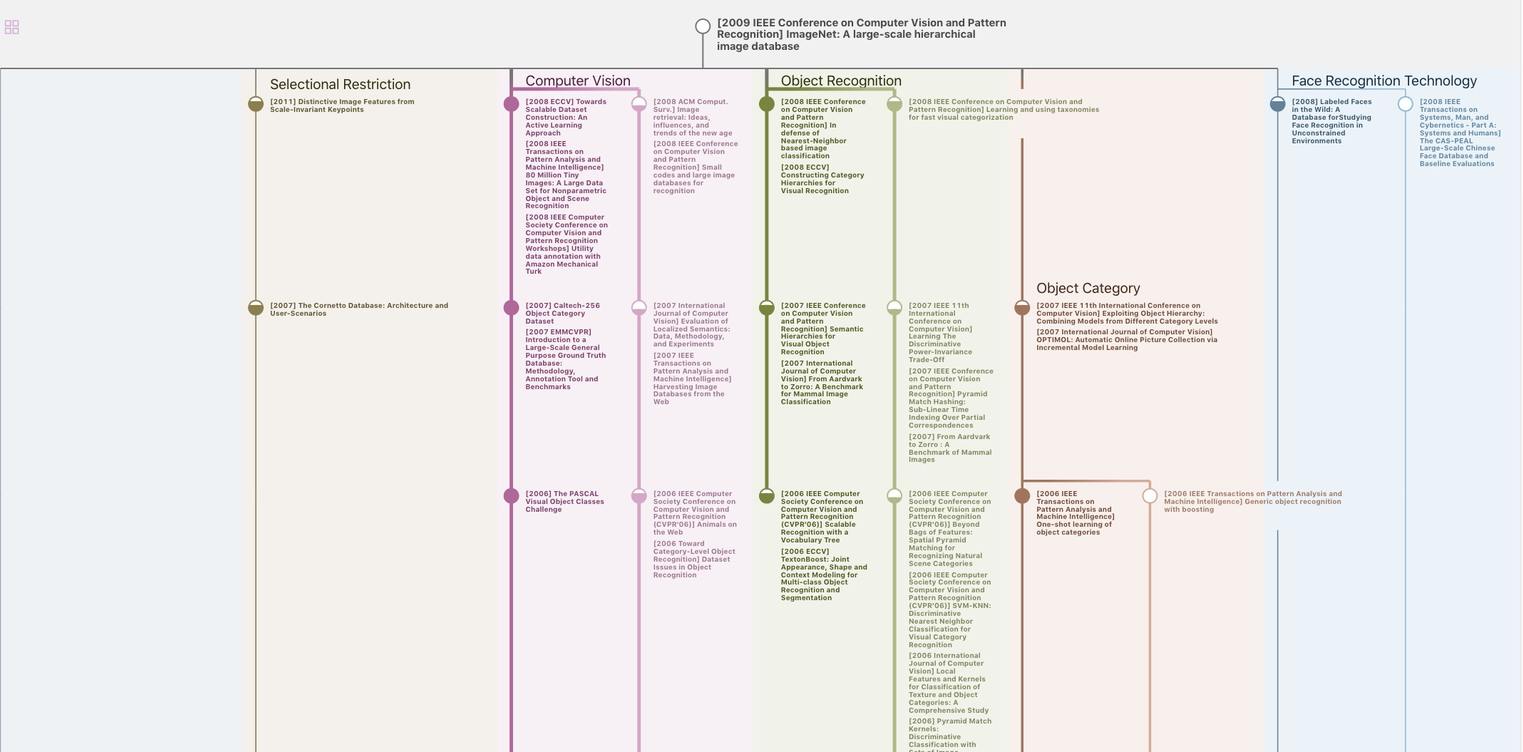
生成溯源树,研究论文发展脉络
Chat Paper
正在生成论文摘要