Feature-scML: An Open-source Python Package for the Feature Importance Visualization of Single-Cell Omics with Machine Learning
CURRENT BIOINFORMATICS(2022)
摘要
Background: Inferring feature importance is both a promise and challenge in bioinformatics and computational biology. While multiple biological computation methods exist to identify decisive factors of single cell subpopulation, there is a need for a comprehensive toolkit that presents an intuitive and custom view of the feature importance.Objective: We developed a Feature-scML, a scalable and friendly toolkit that allows the users to visualize and reveal decisive factors for single-cell omics analysis.Methods: Feature-scML incorporates the following three main functions: (i) There are seven feature selection algorithms to comprehensively score and rank every feature. (ii) Four machine learning approaches and increment feature selection (IFS) strategy jointly determine the number of selected features. (iii) The Feature-scML supports the visualized feature importance, model performance evaluation, and model interpretation. The source code is available at .Results: We systematically compared the performance of seven feature selection algorithms from Feature-scML on two single cell transcriptome datasets. It demonstrates the effectiveness and power of the Feature-scML.Conclusion: Feature-scML is effective for analyzing single-cell RNA omics datasets to automate the machine learning process and customize the visual analysis from the results.
更多查看译文
关键词
Feature ranking,bioinformatics,machine learning,python,feature selection,visualization
AI 理解论文
溯源树
样例
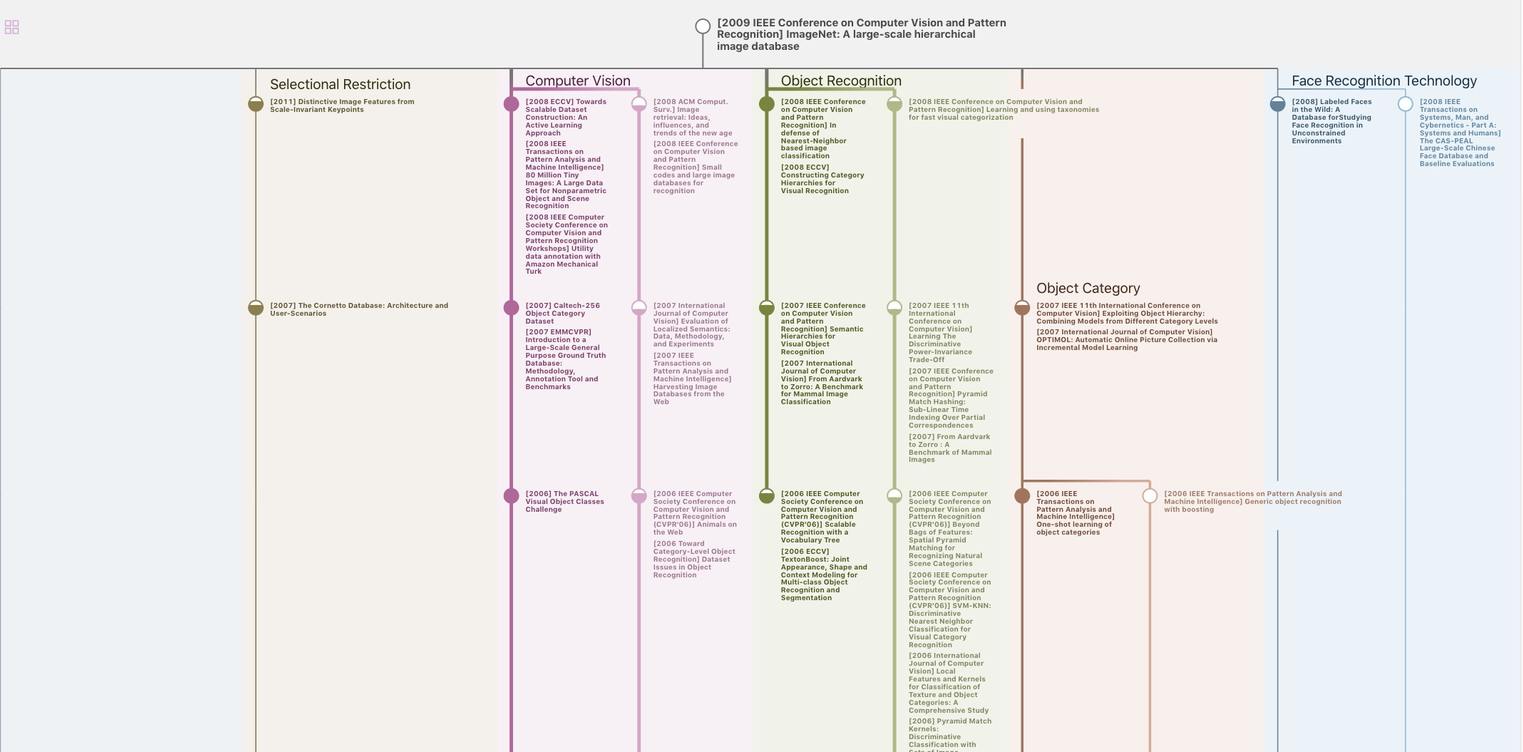
生成溯源树,研究论文发展脉络
Chat Paper
正在生成论文摘要