Monthly streamflow prediction using hybrid extreme learning machine optimized by bat algorithm: a case study of Cheliff watershed, Algeria
HYDROLOGICAL SCIENCES JOURNAL(2023)
摘要
In the present paper, we propose a new approach for monthly streamflow prediction based on the extreme learning machine (ELM) and the metaheuristic bat algorithm (Bat-ELM). The performance of the Bat-ELM was compared to that of ELM, support vector regression (SVR), Gaussian process regression (GPR), multilayer perceptron neural network (MLPNN), and generalized regression neural network (GRNN). The proposed models were applied using data from three hydrometric stations located in the Cheliff Basin, Algeria. The results showed that the Bat-ELM was more satisfactory than the standalone models. The Bat-ELM achieved the highest numerical performance with correlation coefficient and Nash-Sutcliffe efficiency ranging from 0.927 to 0.973 and from 0.846 to 0.944, respectively, much higher than the respective values obtained using the MLPNN, GRNN, SVR, GPR and ELM approaches. The obtained results demonstrate that the Bat-ELM is an interesting alternative algorithm for predicting high and extreme streamflow.
更多查看译文
关键词
streamflow, prediction, ELM, bat, GPR, SVR, MLPNN
AI 理解论文
溯源树
样例
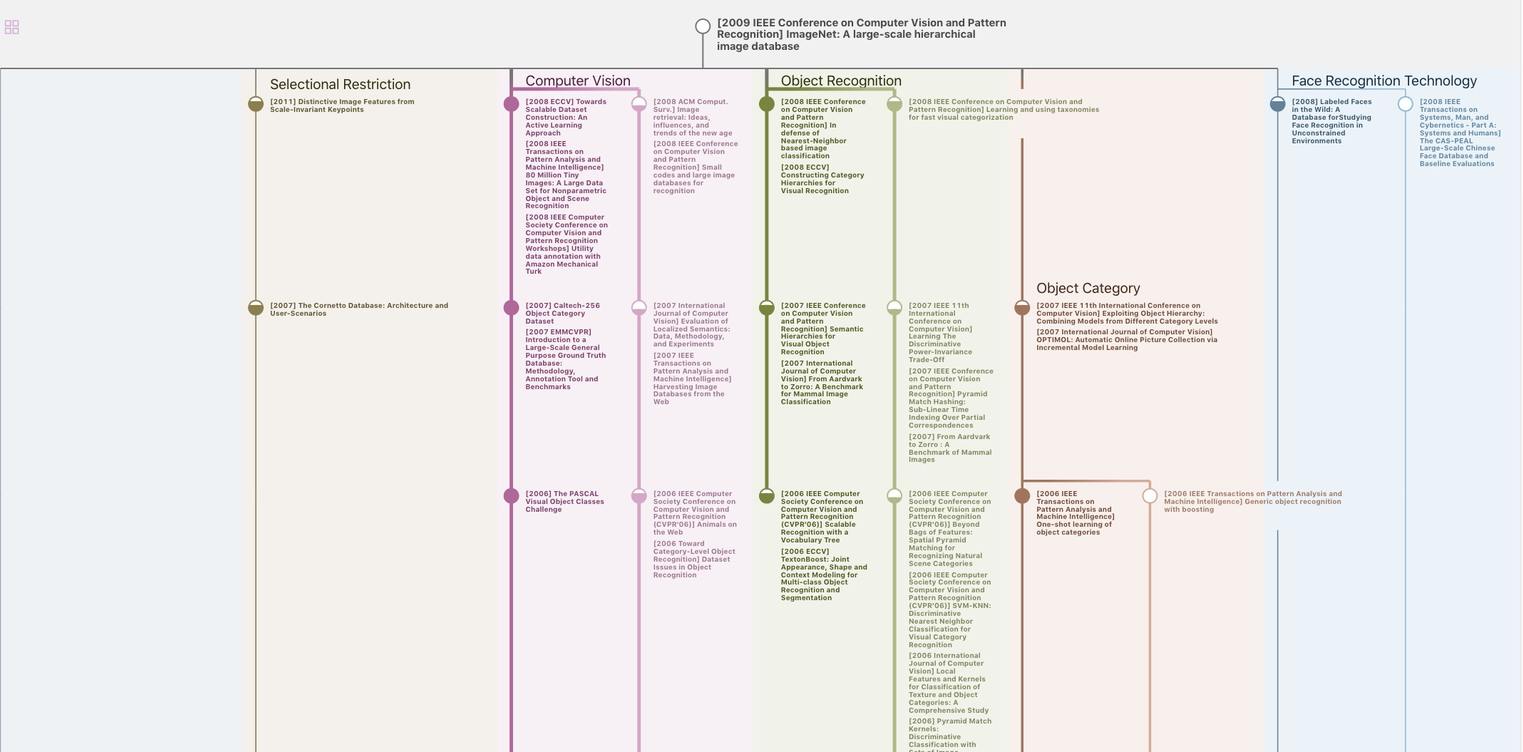
生成溯源树,研究论文发展脉络
Chat Paper
正在生成论文摘要