Fusing collaborative transformation with temporally aware target interaction networks for sequential recommendation
INTERNATIONAL JOURNAL OF MACHINE LEARNING AND CYBERNETICS(2022)
摘要
Sequential recommendation aims to simulate the changes in users’ interests according to their historical behavior data to predict which items they may interact with next. However, most existing sequential recommendation methods (such as attention and recurrent network-based models) utilize only the user’s own behavior sequence for user modeling, ignoring the dynamic transitions between items in the temporal pattern and between users’ complex transition structures arising from multilevel interdependencies. Additionally, traditional methods fuse a sequence representation into a fixed vector, but user interests are diverse, and a single vector does not reflect the diversity of user interests, and a fixed vector representation will limit the representation capability of the model.To address the above problems, this paper proposes a novel sequential recommendation method that fuses collaborative transformations and temporally aware target interaction networks. It can automatically learn the item transformation relationships within and between sequences. We first design a global feature extraction layer. This layer explicitly captures item transitions in different sequences in the form of higher-order connectivity by performing embedding propagation using global graph contexts. The global static representation extracted after collaborative transformation is used as the initial embedding of the sequential pattern, and the user-item interaction information is integrated into the embedded representation to enhance the sequential pattern. Then, different forms of temporal embeddings are fused to capture the dynamic interest changes of users over time. Finally, the candidate target items are used to activate users’ specific interests, and some items in the interaction sequence are enhanced by measuring the correlation between historical items and candidate items, so as to realize diverse user interest modeling and greatly improve the expression ability of the model. Extensive experiments on three real datasets show that our model can effectively improve the recommendation performance compared with existing methods. Code and data are open-sourced at https://github.com/carolmky/FCTT4Rec .
更多查看译文
关键词
Sequential recommendation,Collaborative filtering,Temporal embedding,Target interaction
AI 理解论文
溯源树
样例
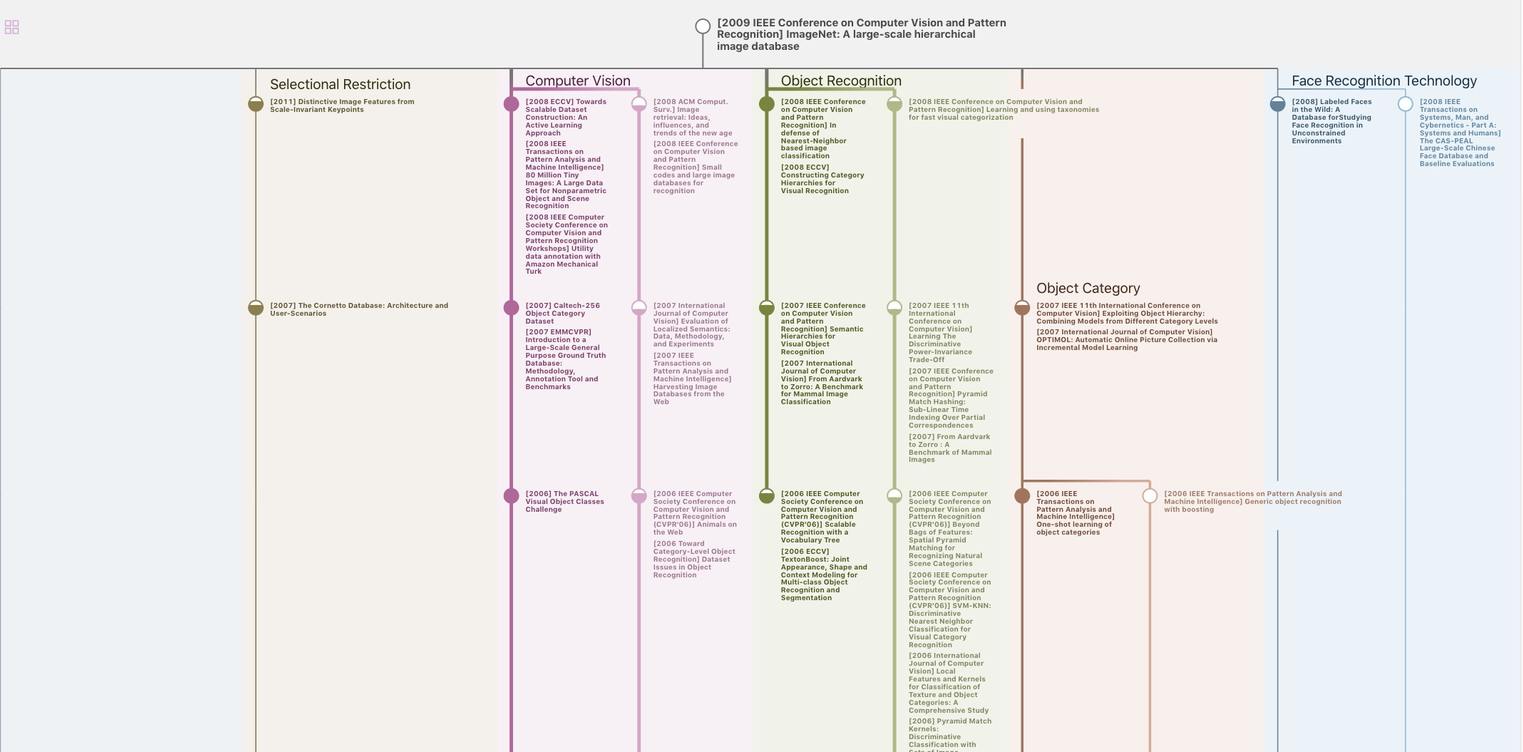
生成溯源树,研究论文发展脉络
Chat Paper
正在生成论文摘要