Detecting Nontechnical Losses in Smart Meters Using a MLP-GRU Deep Model and Augmenting Data via Theft Attacks
SUSTAINABILITY(2022)
摘要
The current study uses a data-driven method for Nontechnical Loss (NTL) detection using smart meter data. Data augmentation is performed using six distinct theft attacks on benign users' samples to balance the data from honest and theft samples. The theft attacks help to generate synthetic patterns that mimic real-world electricity theft patterns. Moreover, we propose a hybrid model including the Multi-Layer Perceptron and Gated Recurrent Unit (MLP-GRU) networks for detecting electricity theft. In the model, the MLP network examines the auxiliary data to analyze nonmalicious factors in daily consumption data, whereas the GRU network uses smart meter data acquired from the Pakistan Residential Electricity Consumption (PRECON) dataset as the input. Additionally, a random search algorithm is used for tuning the hyperparameters of the proposed deep learning model. In the simulations, the proposed model is compared with the MLP-Long Term Short Memory (LSTM) scheme and other traditional schemes. The results show that the proposed model has scores of 0.93 and 0.96 for the area under the precision-recall curve and the area under the receiver operating characteristic curve, respectively. The precision-recall curve and the area under the receiver operating characteristic curve scores for the MLP-LSTM are 0.93 and 0.89, respectively.
更多查看译文
关键词
deep learning, GRU, healthcare, MLP, non-technical losses, PRECON, smart cities, smart grids, smart meters
AI 理解论文
溯源树
样例
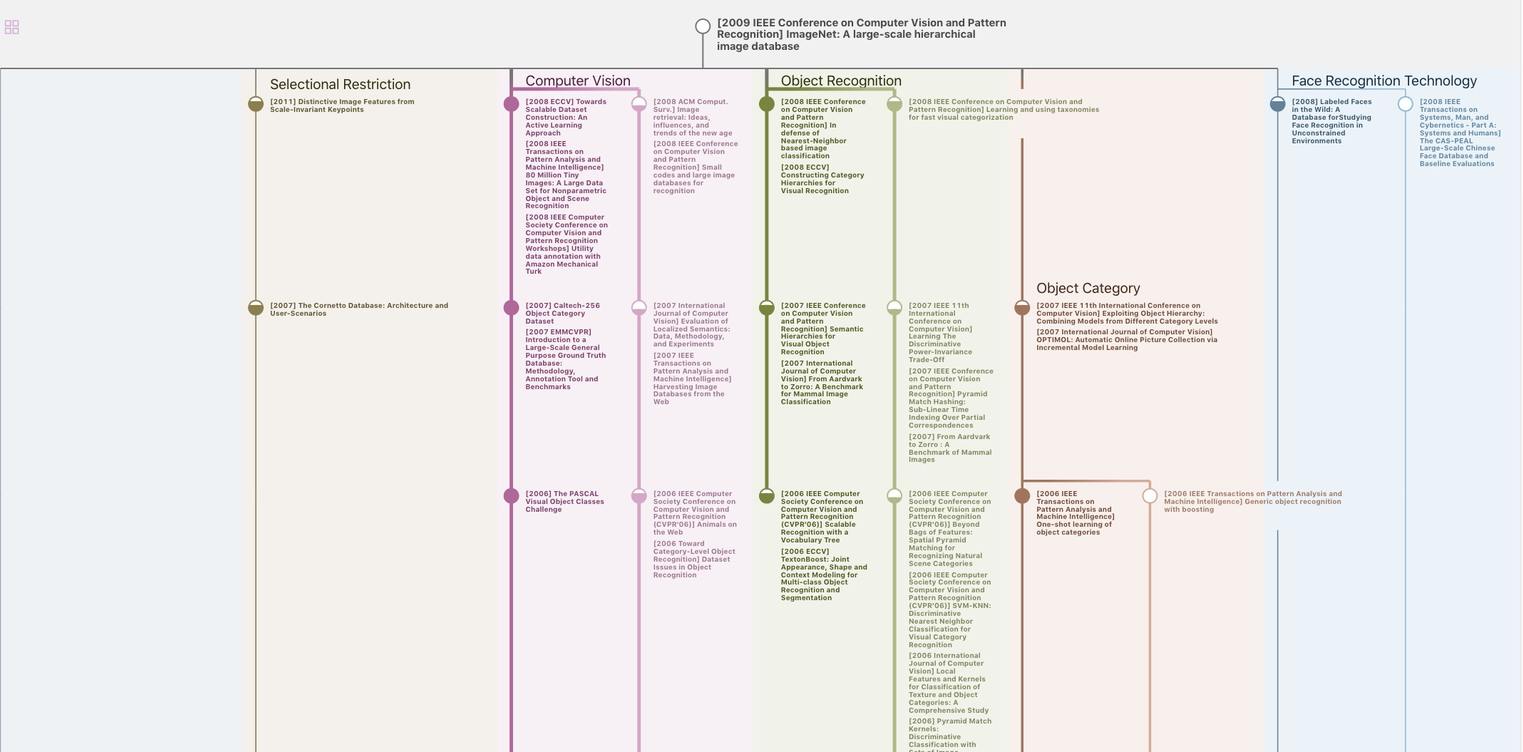
生成溯源树,研究论文发展脉络
Chat Paper
正在生成论文摘要