The population-attributable fraction for time-to-event data
INTERNATIONAL JOURNAL OF EPIDEMIOLOGY(2022)
摘要
Background Even though the population-attributable fraction (PAF) is a well-established metric, it is often incorrectly estimated or interpreted not only in clinical application, but also in statistical research articles. The risk of bias is especially high in more complex time-to-event data settings. Methods We explain how the PAF can be defined, identified and estimated in time-to-event settings with competing risks and time-dependent exposures. By using multi-state methodology and inverse probability weighting, we demonstrate how to reduce or completely avoid severe types of biases including competing risks bias, immortal time bias and confounding due to both baseline and time-varying patient characteristics. Results The method is exemplarily applied to a real data set. Moreover, we estimate the number of deaths that were attributable to ventilator-associated pneumonia in France in the year 2016. The example demonstrates how, under certain simplifying assumptions, PAF estimates can be extrapolated to a target population of interest. Conclusions Defining and estimating the PAF in advanced time-to-event settings within a framework that unifies causal and multi-state modelling enables to tackle common sources of bias and allows straightforward implementation with standard software packages.
更多查看译文
关键词
Attributable fraction,multi-state modelling,competing risks bias,immortal time bias,software implementation
AI 理解论文
溯源树
样例
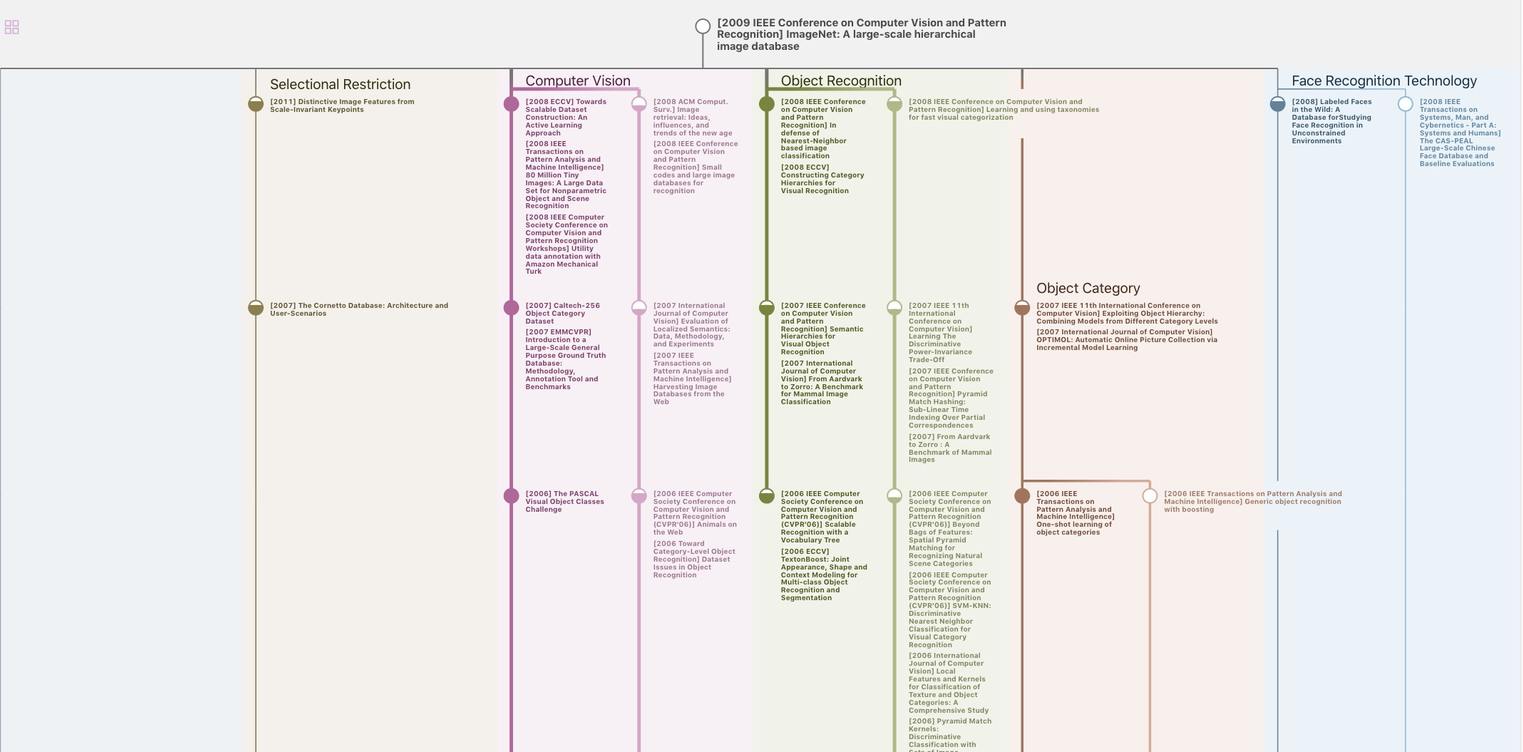
生成溯源树,研究论文发展脉络
Chat Paper
正在生成论文摘要