Fusion of Spectral and Textural Data of Hyperspectral Imaging for Glycine Content Prediction in Beef Using SFCN Algorithms
FOOD ANALYTICAL METHODS(2022)
摘要
Glycine, the simplest free amino acid. It is one of the important factors affecting the flavor of beef. In this study, a fast and non-destructive method combining near-infrared hyperspectral (900–1700 nm) and textural data was first proposed to determine the content and distribution of glycine in beef. On the basis of spectral information pre-processing, spectral features were extracted by the interval variable iterative space shrinkage approach, competitive adaptive reweighting algorithm, and uninformative variable elimination (UVE). The glycine content prediction models were established by partial least squares regression, least squares support vector machine, and the optimized shallow full convolutional neural network (SFCN). Among them, the UVE-SFCN model was found to show better results with prediction set determination coefficient ( R P 2 ) of 0.8725. Furthermore, textural features were extracted by the gray-level co-occurrence matrix and fused with the spectral information of the best feature band to obtain an optimized UVE-FSCN-fusion model ( R P 2 = 0.9005, root mean square error = 0.3075, residual predictive deviation = 0.2688). Compared with the full spectrum and characteristic wavelength spectrum models, R P 2 was improved by 6.41% and 3.10%. The best fusion model was visualized to represent the distribution of glycine in beef. The results showed that the prediction and visualization of glycine content in beef were feasible and effective, and provided a theoretical basis for the hyperspectral study of meat quality monitoring or the establishment of an online platform.
更多查看译文
关键词
Hyperspectral, Glycine, Fusion of spectral and textural data, SFCN algorithms, Visualization
AI 理解论文
溯源树
样例
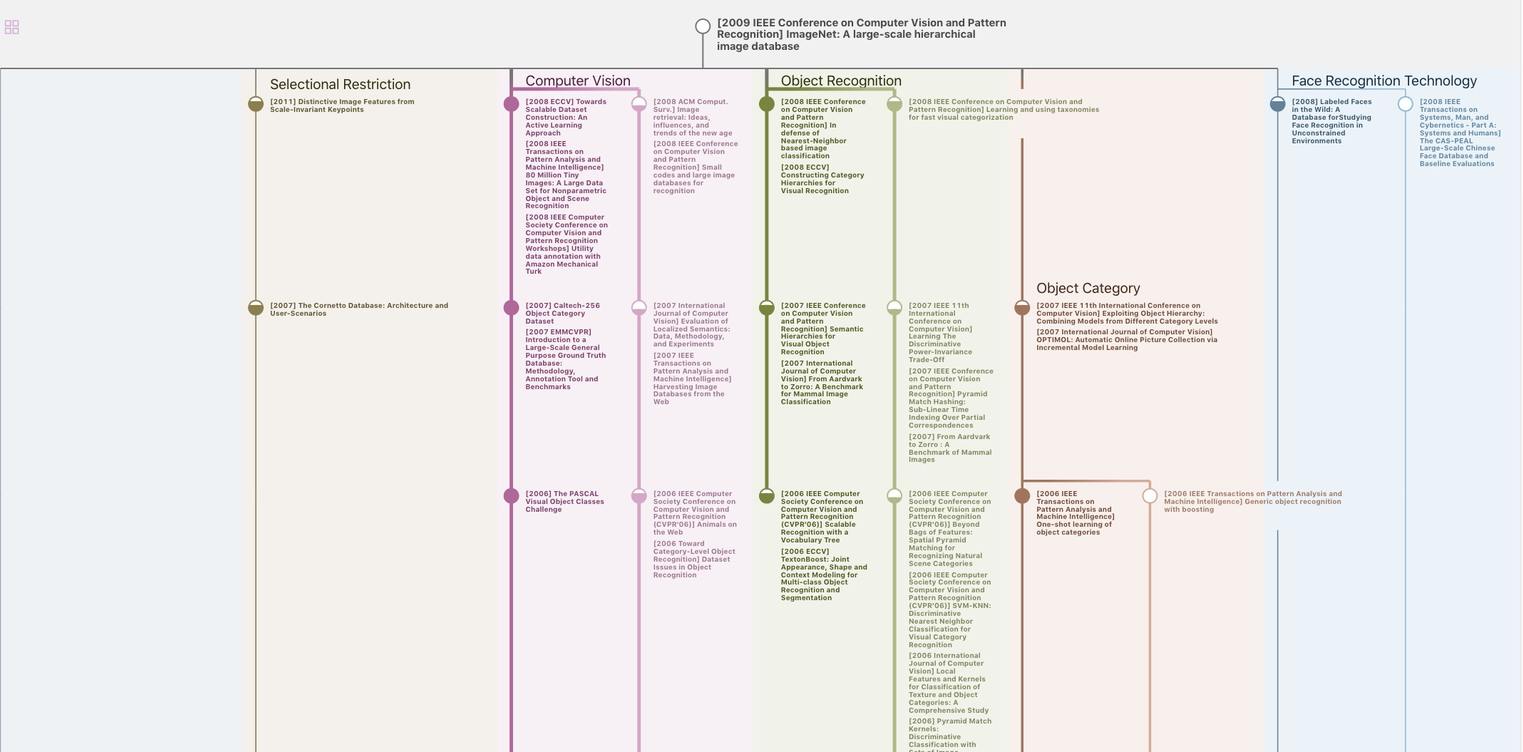
生成溯源树,研究论文发展脉络
Chat Paper
正在生成论文摘要