Sensitivity analysis of driving event classification using smartphone motion data: case of classifier type, sensor bundling, and data acquisition rate
JOURNAL OF INTELLIGENT TRANSPORTATION SYSTEMS(2023)
摘要
Classification of driving events is a crucial stage in driving behavior monitoring using smartphone sensory data. It has not been previously explored that to what extent classification performance depends on the classifier type and input data characteristics. To fill this gap, a real-world experiment is designed for supervised data collection. Then the effects of different machine learning (ML) classifiers, data sampling rates, and sensor combinations on the final classification accuracy are demonstrated. A considerable number of labeled events (4114) containing 11 types of driving maneuvers are collected using base sensors (accelerometer and gyroscope) and composite sensors (linear accelerometer and rotation vector) available in smartphones. Several models using 23 ML algorithms are trained. The sensitivity of these models is analyzed by changing the characteristics of the input data concerning the type of ML classifier, data sampling rate, and the bundle of mobile sensors. It is demonstrated that: (1) F1 scores vary from 70 to 96% for different ML classifiers, (2) F1 scores drop 30–40% depending on the classifier type when reducing the data sampling rate, and (3) using all four sensors as a bundle for classifying driving events is not reasonable since an approximate equal F1 score is achievable by a three-sensor bundle which includes an accelerometer and a linear accelerometer.
更多查看译文
关键词
driving behavior,machine learning,mobile sensors,sensitivity analysis
AI 理解论文
溯源树
样例
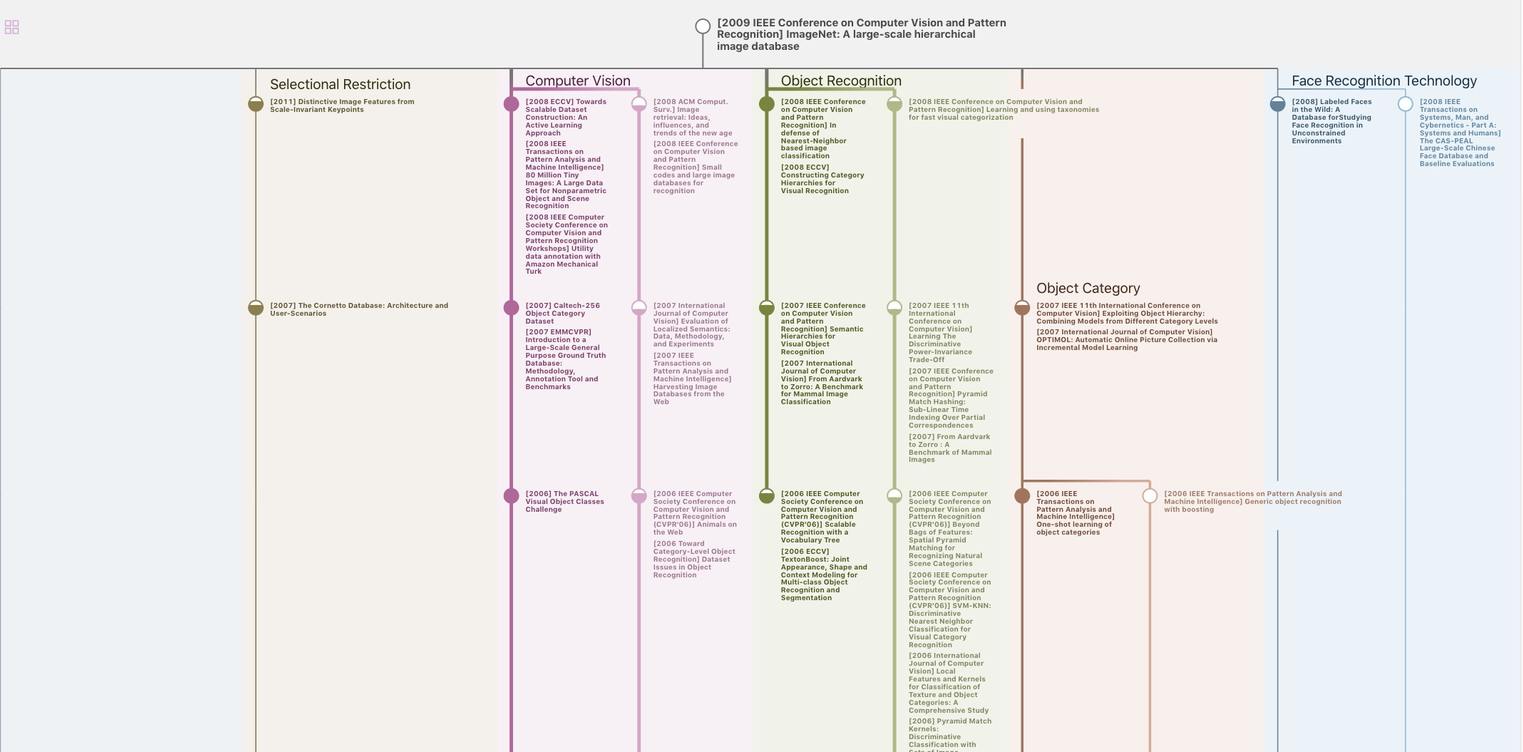
生成溯源树,研究论文发展脉络
Chat Paper
正在生成论文摘要