Machine-Learning-Driven High-Entropy Alloy Catalyst Discovery to Circumvent the Scaling Relation for CO2 Reduction Reaction
ACS CATALYSIS(2022)
摘要
To achieve an equitable energy transition toward net-zero 2050 goals, the electrochemical reduction of CO2 (CO2RR) to chemical feedstocks through utilizing both CO2 and renewable energy is particularly attractive. However, the catalytic activity of CO2RR is limited by the scaling relation of the adsorption energies of intermediates. Circumventing the scaling relation is a potential strategy to achieve a breakthrough in catalytic activity. Herein, based on density functional theory (DFT) calculations, we designed a high-entropy alloy (HEA) system of FeCoNiCuMo with high catalytic activity for CO2RR. Machine learning models were developed by considering 1280 adsorption sites to predict the adsorption energies of COOH*, CO*, and CHO*. The scaling relation between the adsorption energies of COOH*, CO*, and CHO* is circumvented by the rotation of COOH* and CHO* on the designed HEA surface, resulting in the outstanding catalytic activity of CO2RR with the limiting potential of 0.29-0.51 V. This work not only accelerates the development of HEA catalysts but also provides an effective strategy to circumvent the scaling relation.
更多查看译文
关键词
scaling relation, CO2 reduction reaction, high-entropy alloy catalysts, density functional theory, machine learning
AI 理解论文
溯源树
样例
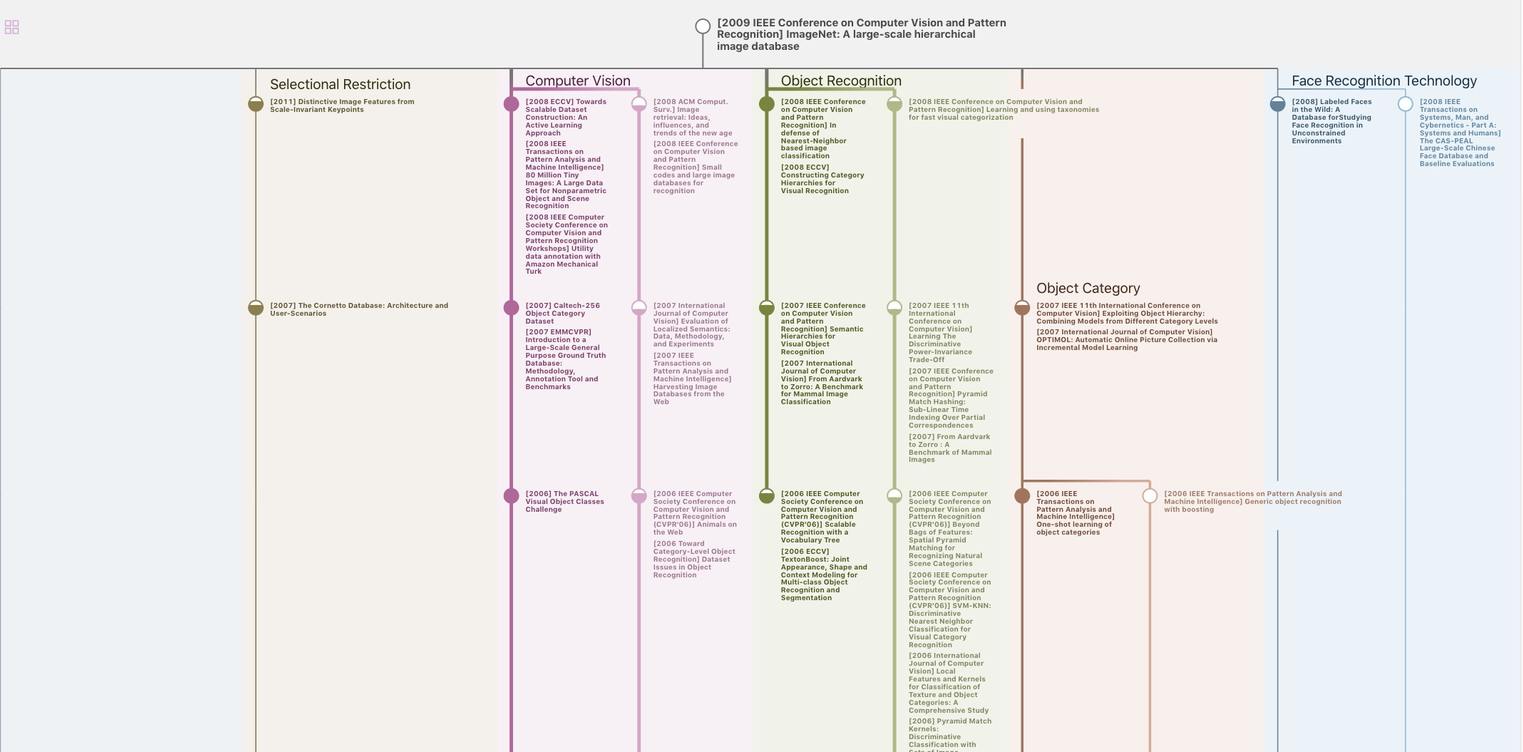
生成溯源树,研究论文发展脉络
Chat Paper
正在生成论文摘要