Interpreting the prediction results of the tree-based gradient boosting models for financial distress prediction with an explainable machine learning approach
JOURNAL OF FORECASTING(2022)
摘要
Financial distress prediction is a major issue in the burgeoning fintech field. Given the importance of the reliability of the prediction results, there is an urgent need for the explanatory ability of the financial distress prediction model. From the modeling and explanation point of view, this study employs four prevailing tree-based gradient boosting models, namely, gradient boosting decision tree, extreme gradient boosting, light gradient boosting machine, and categorical boosting, to build financial distress prediction models by using financial data of listed companies in China from 1998 to 2014 and five different prediction time spans. We observe that tree-based gradient boosting models have better prediction performance than other prediction models. To explore the reasons for the prediction results, we deploy TreeSHAP. Then, we use Shapley regression to examine the statistically significant relationships between financial indicators and financial distress. We discover that financial indicators, such as net asset value per share and ratio of operating profits to current liabilities, are significantly related to financial distress. There is usually a nonlinear relationship between the financial predictors and prediction target. Thus, this study provides an effective method for financial distress prediction and an explanation of the results for listed companies in China.
更多查看译文
关键词
data mining, explainable machine learning, financial distress prediction, tree-based gradient boosting models
AI 理解论文
溯源树
样例
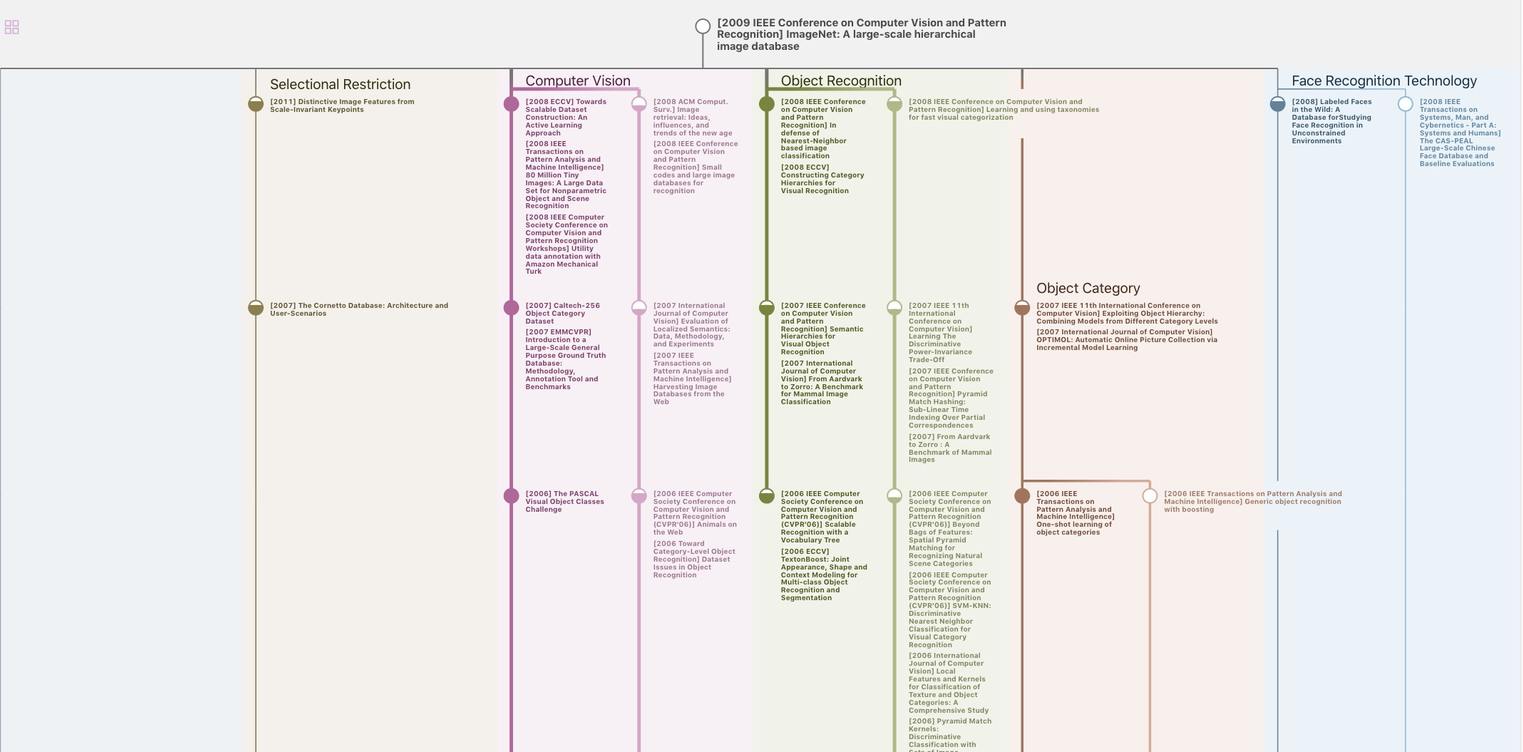
生成溯源树,研究论文发展脉络
Chat Paper
正在生成论文摘要