Importance of Spatial Autocorrelation in Machine Learning Modeling of Polymetallic Nodules, Model Uncertainty and Transferability at Local Scale
MINERALS(2021)
摘要
Machine learning spatial modeling is used for mapping the distribution of deep-sea polymetallic nodules (PMN). However, the presence and influence of spatial autocorrelation (SAC) have not been extensively studied. SAC can provide information regarding the variable selection before modeling, and it results in erroneous validation performance when ignored. ML models are also problematic when applied in areas far away from the initial training locations, especially if the (new) area to be predicted covers another feature space. Here, we study the spatial distribution of PMN in a geomorphologically heterogeneous area of the Peru Basin, where SAC of PMN exists. The local Moran's I analysis showed that there are areas with a significantly higher or lower number of PMN, associated with different backscatter values, aspect orientation, and seafloor geomorphological characteristics. A quantile regression forests (QRF) model is used using three cross-validation (CV) techniques (random-, spatial-, and cluster-blocking). We used the recently proposed "Area of Applicability" method to quantify the geographical areas where feature space extrapolation occurs. The results show that QRF predicts well in morphologically similar areas, with spatial block cross-validation being the least unbiased method. Conversely, random-CV overestimates the prediction performance. Under new conditions, the model transferability is reduced even on local scales, highlighting the need for spatial model-based dissimilarity analysis and transferability assessment in new areas.
更多查看译文
关键词
polymetallic nodules, spatial autocorrelation, cross-validation, model transferability
AI 理解论文
溯源树
样例
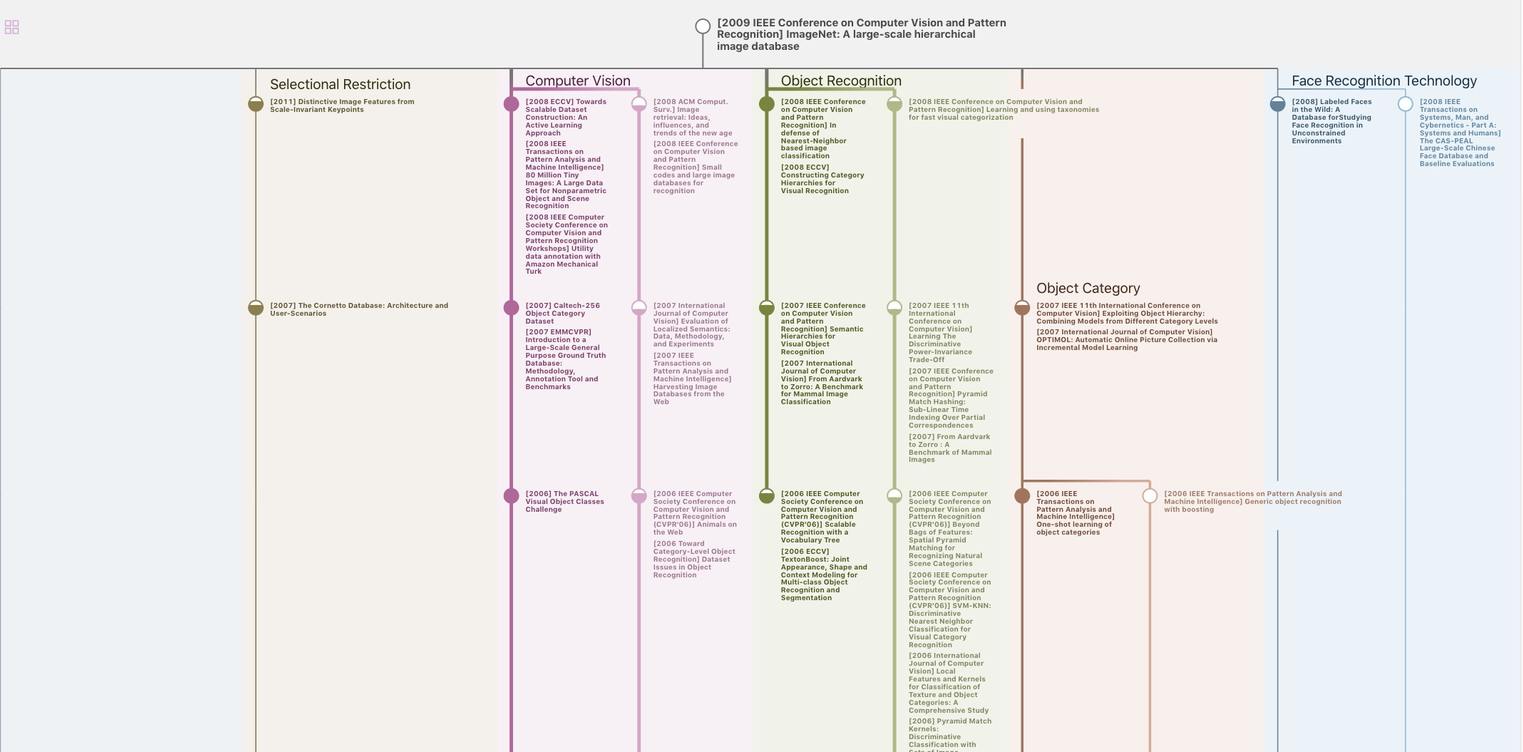
生成溯源树,研究论文发展脉络
Chat Paper
正在生成论文摘要