Learning attentive attribute-aware node embeddings in dynamic environments
International Journal of Data Science and Analytics(2024)
摘要
Learning node embeddings is fundamental for numerous applications, such as link prediction and node classification. Node embeddings seek to learn a low-dimensional representation for each node in the graph. Many existing node representation learning methods for dynamic attributed graphs focus on preserving the temporal proximity of the nodes with relatively shallow models. However, real-life graphs are complex and usually exhibit evolutionary patterns of node attributes and graph structure. Therefore, the current state-of-the-art models fail to capture the information in the dynamic attributed graphs and settle for sub-optimal results. In this paper, we propose a novel model for embedding nodes in dynamic attributed graphs that captures the full extent of all relevant node information and the graph interrelations as well as graph evolutionary patterns. During model training, attribute-aware node embedding is learned using both graph and node properties in a dynamic context. Experiments demonstrate that our proposed method is superior to the state-of-the-art models in link prediction tasks. In addition, it introduces a novel way of learning richer representations by fully exploiting node attributes, graph structure, and evolutionary patterns in dynamic attributed graphs.
更多查看译文
关键词
Attributed network,Dynamic network,Graph embedding,Unsupervised feature extraction,Self-attention
AI 理解论文
溯源树
样例
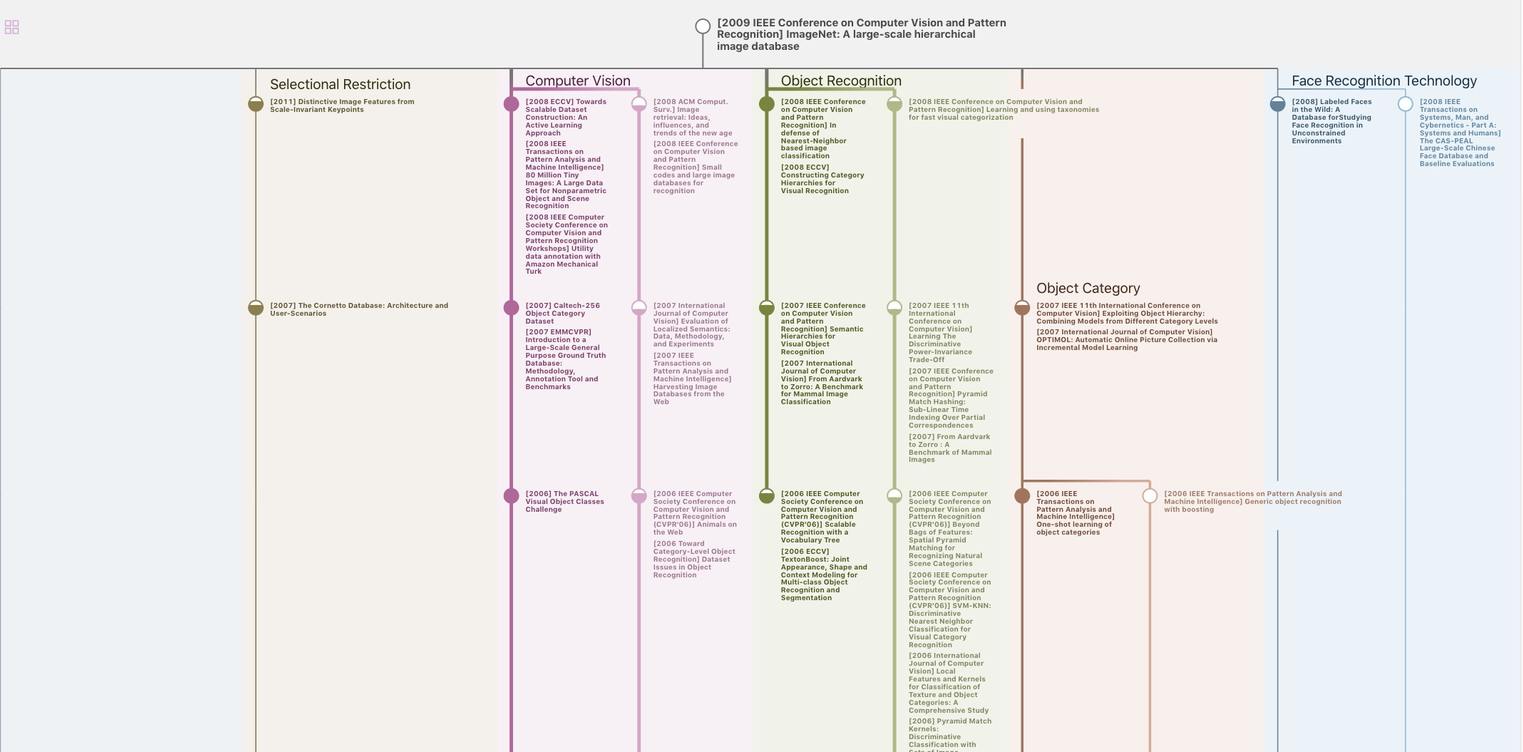
生成溯源树,研究论文发展脉络
Chat Paper
正在生成论文摘要