General Community Detection in Attributed Networks with Consistent-Module Constrained Nonnegative Matrix Factorization
WIRELESS COMMUNICATIONS & MOBILE COMPUTING(2022)
摘要
Nonnegative matrix factorization (NMF) model has been successfully applied to discover latent community structures due to its good performance and interpretability advantages in extracting hidden patterns. However, most previous studies explore only the structural information of the network while ignoring the rich attributes. Besides, they aim at detecting densely connected communities (also called community structures) and fail to identify general structures, such as bipartite structures and mixture structures. In this paper, we research on general structure discovery and propose a new method GCDNMF (General Community Detection based on Nonnegative Matrix Factorization), which integrates structural information and node attributes through consistency module constraint to capture the community interactions. It can discover the general community structures of nodes by iteratively updating the community-interaction matrix and the node-membership matrix. We also introduce matrix initialization based on centrality and dispersion of nodes for center selection to reduce the sensitivity of random initialization. Experimental results on real-world networks with a variety of characteristics validate the performance of our approach, especially on networks with general structures. In addition, the associated initialization evaluations demonstrate the effectiveness of our method in obtaining stable results.
更多查看译文
AI 理解论文
溯源树
样例
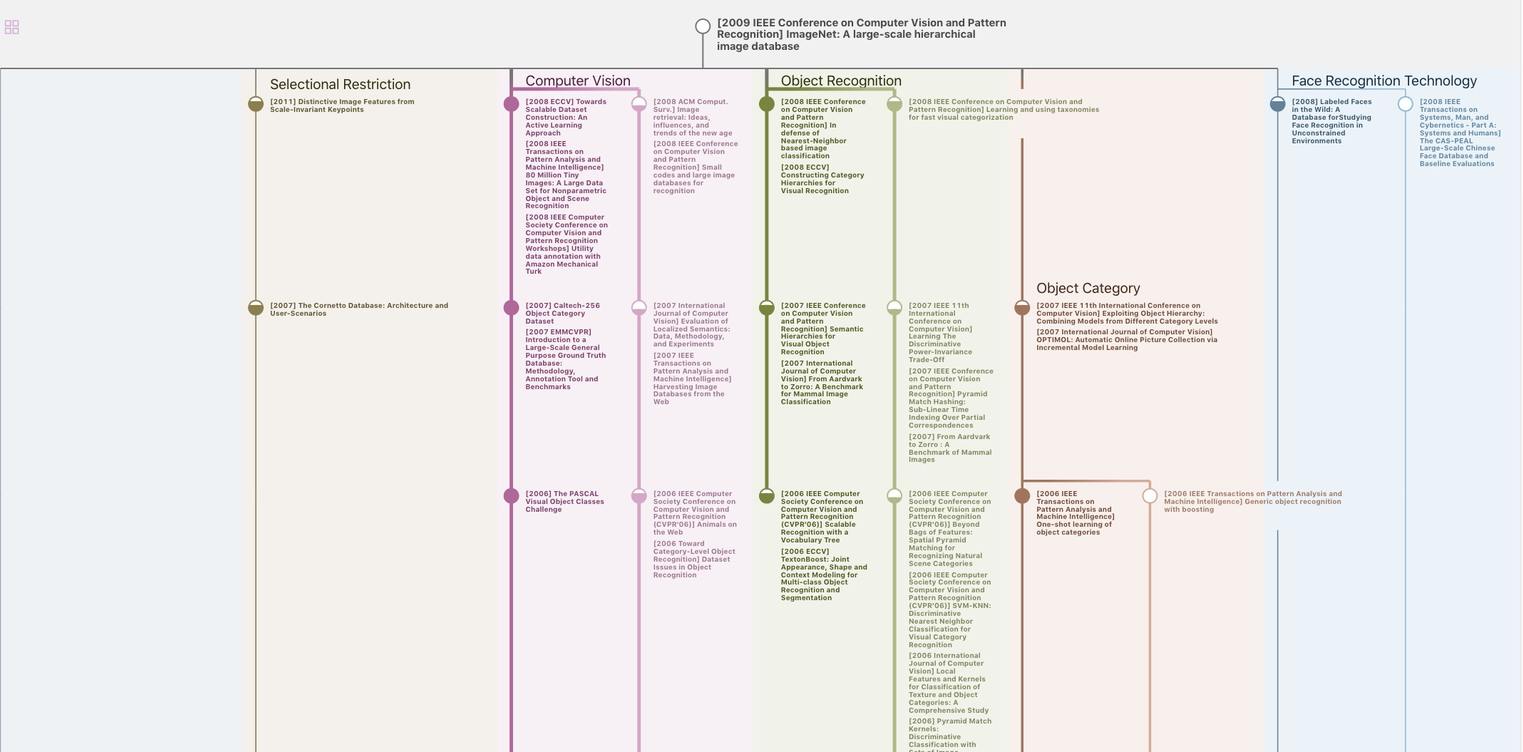
生成溯源树,研究论文发展脉络
Chat Paper
正在生成论文摘要