Accelerating the design of high-entropy alloys with high hardness by machine learning based on particle swarm optimization
INTERMETALLICS(2023)
摘要
Combining a machine-learning (ML) surrogate model with a particle swarm optimization (PSO) algorithm, we propose a design strategy to search for high-entropy alloys (HEAs) with high hardness in the Al-Co-Cr-Cu-Fe-Ni system. The relationship between various materials descriptors and a targeted property can be established by the ML models based on the data set, which contains the mole fraction of each element. In this study, we use random forest (RF) to rank the feature importance based on the materials descriptors. It shows that the valence-electron concentration (VEC) and atomic-size difference (delta) are the two most critical factors to the hardness. The random forest combined particle swarm optimization is proposed to design the HEAs with high hardness in a search space containing nearly 65,000 data for the Al-Co-Cr-Cu-Fe-Ni system. We present the top 10 HEAs with high hardness, which are greater than the existing alloys in this HEAs system.
更多查看译文
关键词
High-entropy alloy,Alloy design,Physical properties
AI 理解论文
溯源树
样例
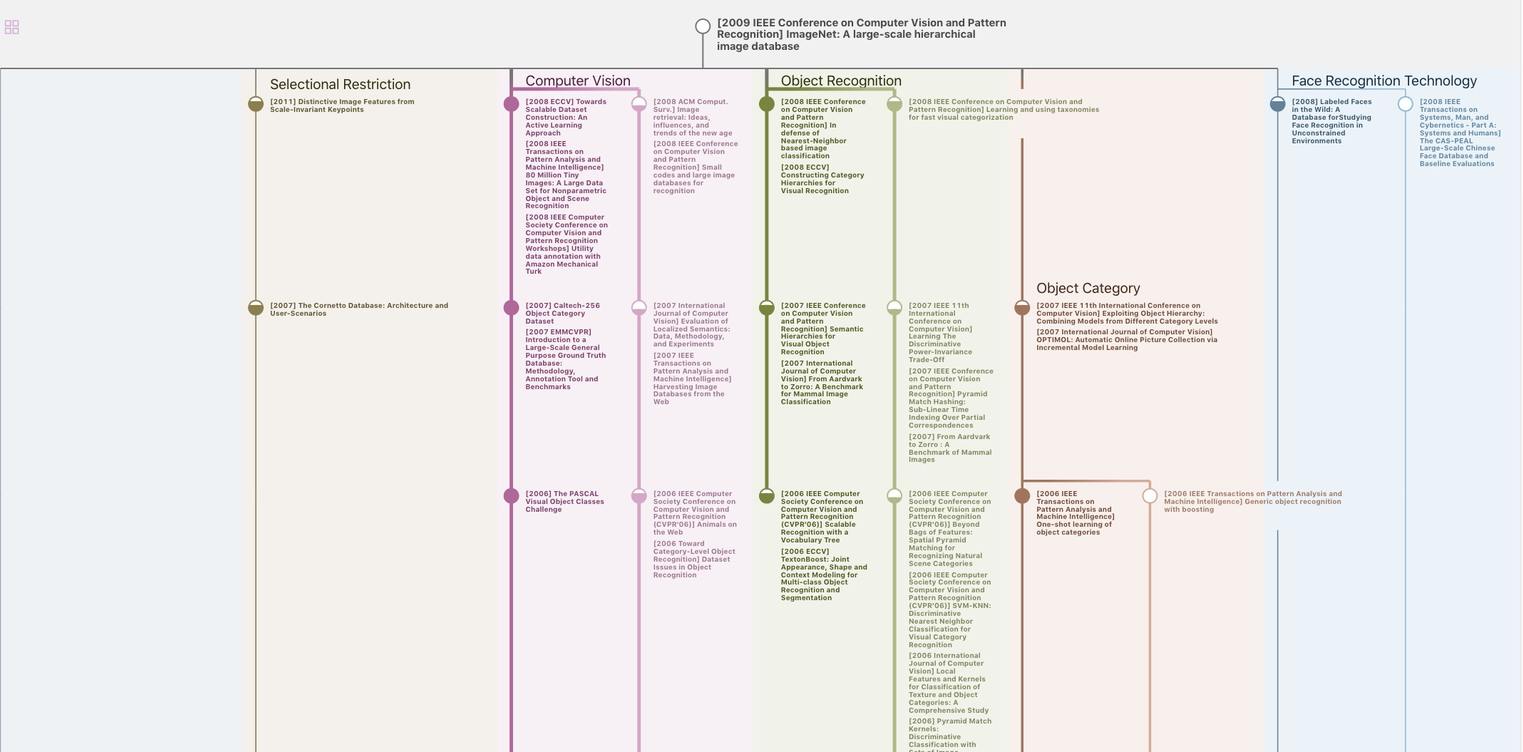
生成溯源树,研究论文发展脉络
Chat Paper
正在生成论文摘要