Bottleneck-Stationary Compact Model Accelerator With Reduced Requirement on Memory Bandwidth for Edge Applications
IEEE Transactions on Circuits and Systems I: Regular Papers(2023)
摘要
State-of-the-art compact models such as MobileNets and EfficientNets are structured using a linear bottleneck and inverted residuals. Hardware architecture using a single dataflow strategy fails to balance the required memory bandwidth with the given computational resources. This work presents a heterogeneous dual-core accelerator that performs a block-wise pipelined process as a unit using a bottleneck-stationary (BS) dataflow. The BS greatly relieves the requirement on DRAM bandwidth and on-chip SRAM capacity. A look-behind-only attention is also proposed as a co-optimized algorithm. Compared to the state-of-the-art hardware scheme, the proposed accelerator demonstrates a reduction of 1.8-
$2.9\times $
in latency and 2.2-
$3\times $
in energy consumption, respectively.For verification, the accelerator with a 16-bit integer precision was implemented using 28nm CMOS process. Measurements show energy efficiencies of 0.5-to-3.75 TOPS/W in a supply voltage range of 0.55-to-1.15V.
更多查看译文
关键词
Co-optimization for compact models,DNN accelerator,dataflow,data reuse,edge devices,memory bandwidth
AI 理解论文
溯源树
样例
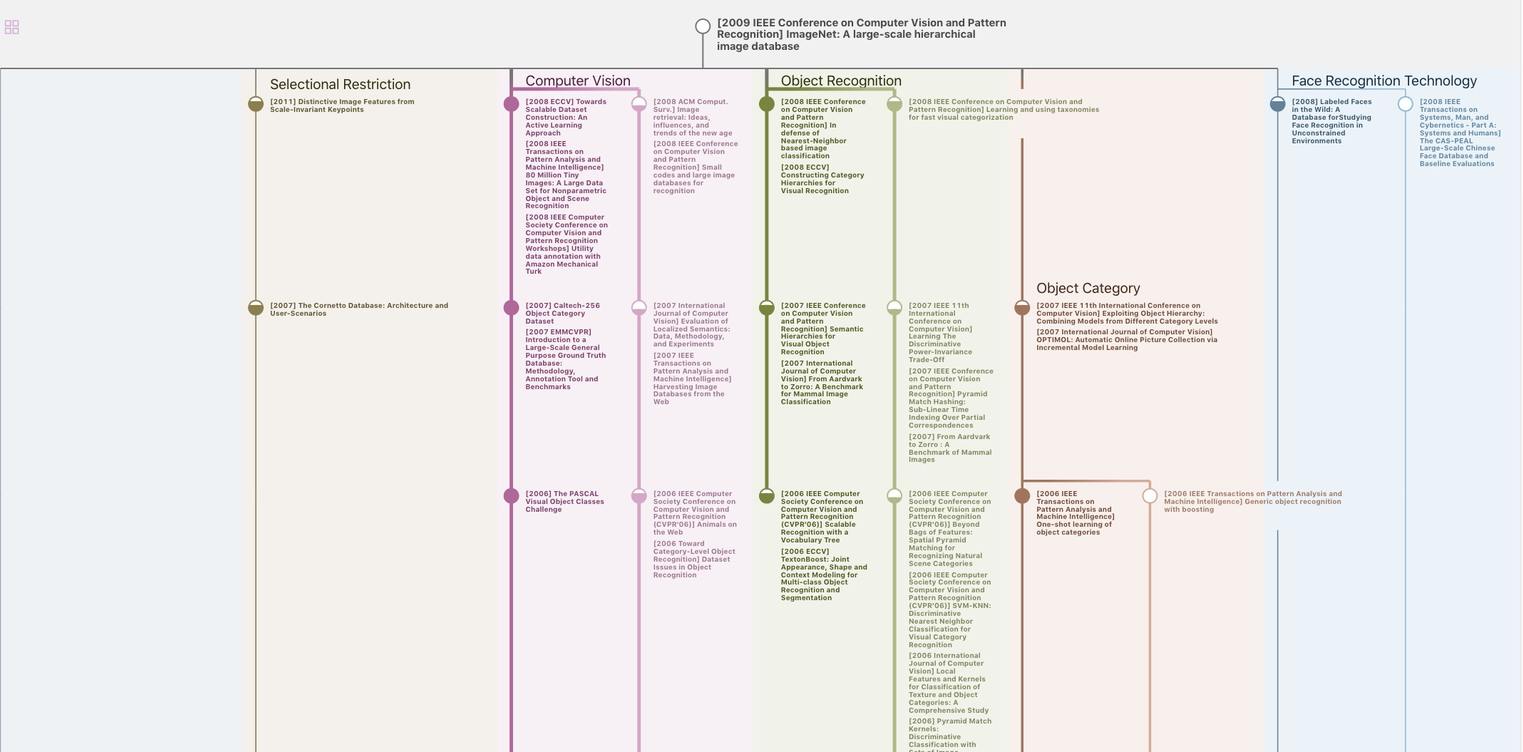
生成溯源树,研究论文发展脉络
Chat Paper
正在生成论文摘要