Transthyretin amyloid cardiomyopathy among patients hospitalized for heart failure and performance of an adapted wild-type ATTR-CM machine learning model: Findings from GWTG-HF
CIRCULATION(2023)
摘要
Background An 11-factor random forest model has been developed among ambulatory heart failure (HF) patients for identifying potential wild-type amyloidogenic TTR cardiomyopathy (wtATTR-CM). The model has not been evaluated in a large sample of patients hospitalized for HF. Methods This study included Medicare beneficiaries aged & GE;65 years hospitalized for HF in the Get With The GuidelinesHF & REG; Registry from 2008-2019. Patients with and without a diagnosis of ATTR-CM were compared, as defined by inpatient and outpatient claims data within 6 months pre-or post-index hospitalization. Within a cohort matched 1:1 by age and sex, univariable logistic regression was used to evaluate relationships between ATTR-CM and each of the 11 factors of the established model. Discrimination and calibration of the 11-factor model were assessed. Results Among 205,545 patients (median age 81 years) hospitalized for HF across 608 hospitals, 627 patients (0.31%) had a diagnosis code for ATTR-CM. Univariable analysis within the 1:1 matched cohort of each of the 11-factors in the ATTRCM model found pericardial effusion, carpal tunnel syndrome, lumbar spinal stenosis, and elevated serum enzymes (e.g., troponin elevation) to be strongly associated with ATTR-CM. The 11-factor model showed modest discrimination (c-statistic 0.65) and good calibration within the matched cohort. Conclusions Among US patients hospitalized for HF, the number of patients with ATTR-CM defined by diagnosis codes on an inpatient/outpatient claim within 6 months of admission was low. Most factors within the prior 11-factor model were associated with greater odds of ATTR-CM diagnosis. In this population, the ATTR-CM model demonstrated modest discrimination.
更多查看译文
关键词
heart failure,machine learning,wild-type
AI 理解论文
溯源树
样例
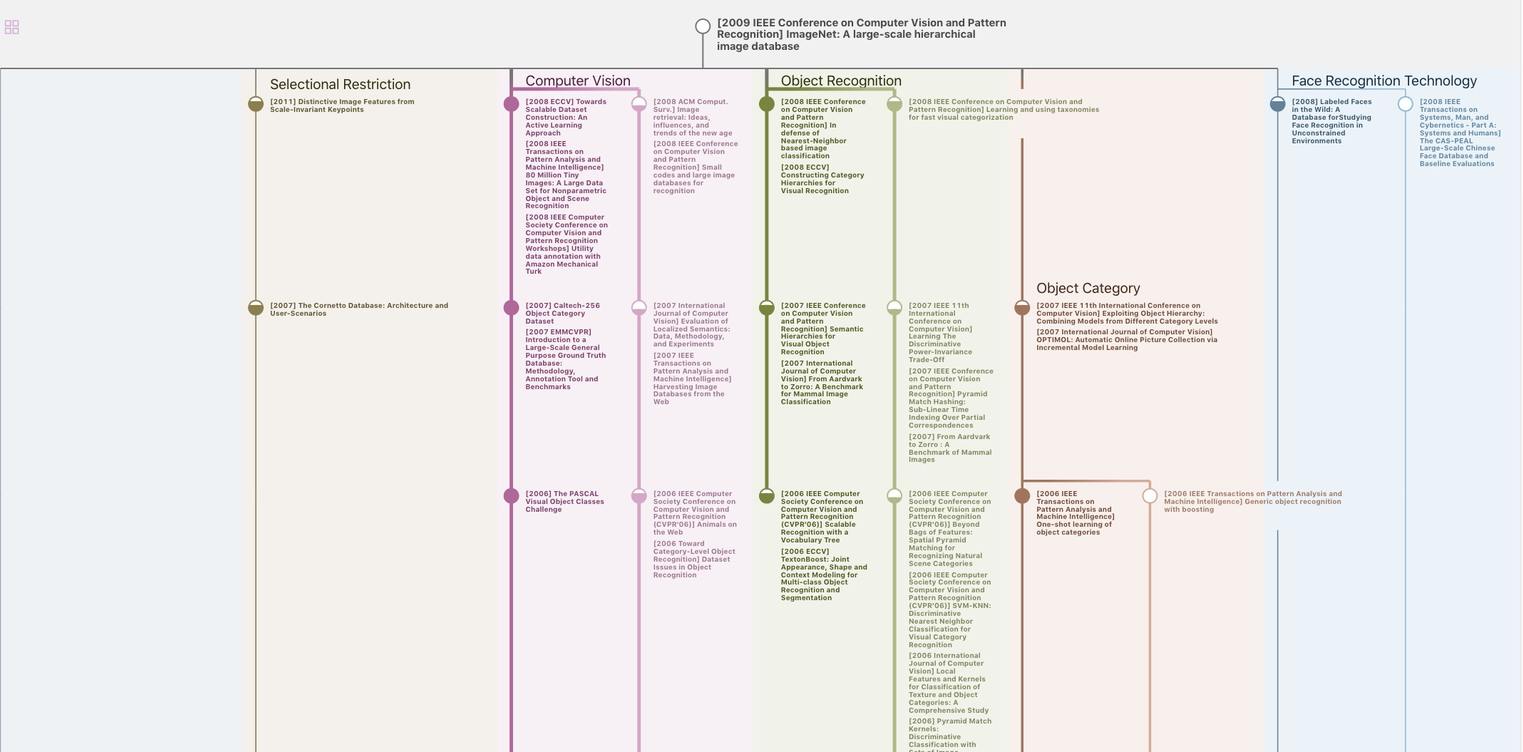
生成溯源树,研究论文发展脉络
Chat Paper
正在生成论文摘要