Twin prototype networks with noisy label self-correction for fault diagnosis of wind turbine gearboxes
MEASUREMENT SCIENCE AND TECHNOLOGY(2023)
摘要
Deep strong-supervised learning-based methods have been widely used and have made significant progress in intelligent fault diagnosis for wind turbine (WT) gearboxes. The superior performance of such methods relies on high-quality labels. However, correctly labeling the data is challenging because of the complexity of fault vibration signals and fault modes in real industrial scenarios, resulting in noisy labels in datasets, which significantly restricts the application of strong-supervised fault diagnosis models. In this study, a method based on twin prototype networks with noisy label self-correction was proposed to address fault diagnosis for WT gearboxes with noisy labels. This method introduced a collaborative learning architecture to improve the confirmation bias in the self-training of individual networks and to slow the speed of learning noisy-labeled samples. Simultaneously, the loss distribution of the samples from each network was modeled using the Gaussian mixture model to dynamically identify mislabeled samples in the training dataset. Finally, a collaborative relabeling prototype refinement module was designed to optimize the prototype learning process and enable self-correction of noisy labels. The experiments demonstrated the effectiveness and superiority of the proposed method.
更多查看译文
关键词
fault diagnosis,prototype network,Gaussian mixture model,noisy labels,collaborative learning
AI 理解论文
溯源树
样例
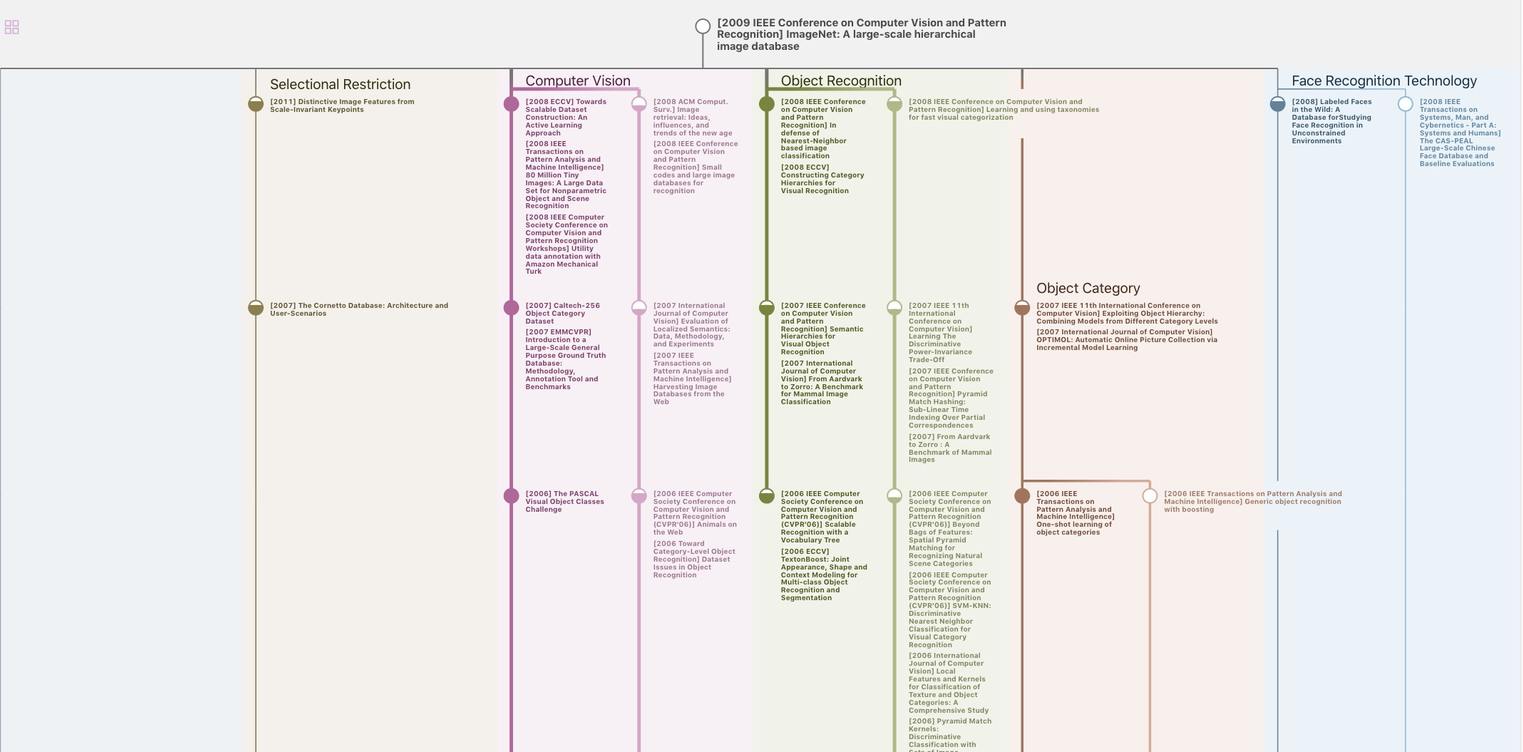
生成溯源树,研究论文发展脉络
Chat Paper
正在生成论文摘要