Improving Occupant Thermal Comfort through Personalized Space Recommendation
JOURNAL OF COMPUTING IN CIVIL ENGINEERING(2023)
摘要
Thermal comfort significantly affects occupants' satisfaction, well-being, and productivity in built environments. To improve thermal comfort, existing literature has investigated human-in-the-loop HVAC control and personal comfort systems (PCS) to adjust the macro- and microenvironments surrounding occupants to meet their preferences. However, these methods have limitations, including the inability to satisfy all occupants, energy waste due to undesired fluctuations in HVAC settings, uncertainty in energy savings, high upfront costs, lack of scalability, to name a few. In contrast, this study proposed a SpaceMatch framework by reimagining occupants as mobile agents who are willing to move to spaces where the conditions best match their personal preferences and needs. This framework leverages personal comfort models developed for each occupant using machine learning and natural spatial-temporal temperature variations in buildings to make space recommendations. An experiment with 12 subjects was conducted in a testbed building from October to November 2021 at Clemson University to validate the proposed framework. The results showed that subjects' thermal comfort increased at least by 18.8% with the help of space recommendations compared to the baseline, without incurring additional energy use. This framework has great potential in many built environments where flexible workplace strategies are employed, such as open-plan offices and libraries, especially in the postpandemic era when people's working habits have significantly changed because of remote work, job autonomy, and flexible scheduling.
更多查看译文
关键词
Thermal comfort, Personal comfort model, Activity-based workspace, Space match, Machine learning
AI 理解论文
溯源树
样例
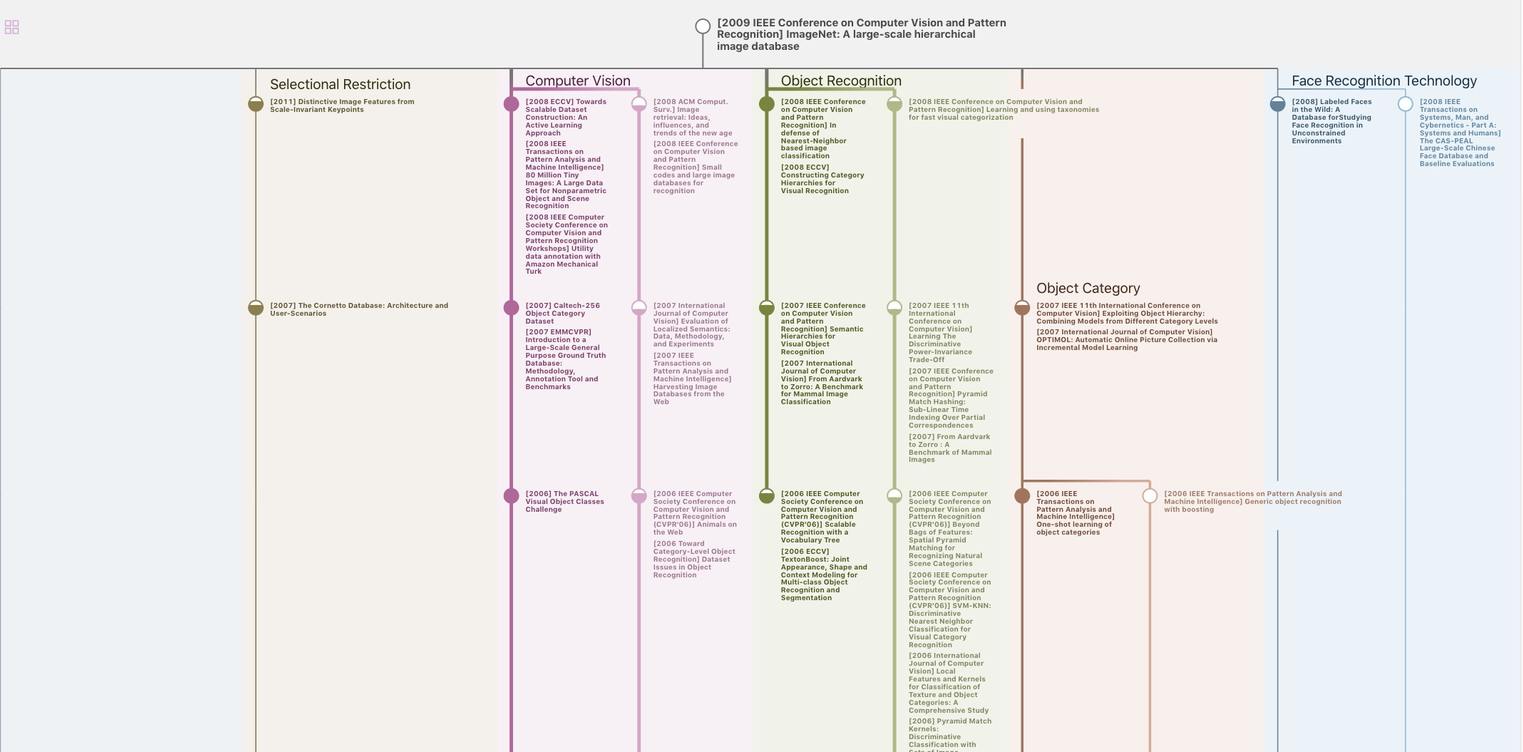
生成溯源树,研究论文发展脉络
Chat Paper
正在生成论文摘要