Structural Deterioration Knowledge Ontology towards Physics-Informed Machine Learning for Enhanced Bridge Deterioration Prediction
JOURNAL OF COMPUTING IN CIVIL ENGINEERING(2023)
摘要
The structural deterioration knowledge in existing mathematical physics models offers a unique opportunity to develop data-driven, physics-informed machine learning (ML) for enhanced bridge deterioration prediction. However, existing physics models typically represent deterioration knowledge using mathematical symbols and are limited in capturing the semantic meanings and relationships of the symbols, making the models not understandable and operable by machines. As a result, it is challenging to automatically instantiate mathematical physics models to deduce knowledge-driven deterioration facts to inform the learning. To address this challenge, this paper proposes a new structural deterioration knowledge ontology (DT-KL-Onto). The ontology represents structural deterioration knowledge in mathematical physics models as related to structure deficiency, deficiency property, and deficiency contributing factor in a formal, explicit, and semantic way to allow for automated model instantiation. To evaluate the performance of the ontology, the DT-KL-Onto was implemented in automatically instantiating structural deterioration-related mathematical physics models using bridge design, construction, and inspection data. The evaluation results show that the ontology was able to accurately instantiate the models in an automated way: the coefficient of determination and mean squared error between the deterioration facts deduced from the automated instantiation and those from the manual instantiation are 1.0000 and 0.0000, respectively. Two bridge deterioration prediction models were also developed to further demonstrate the benefits of using the deterioration knowledge captured by the ontology to inform the learning. Compared to the purely ML-based prediction model, the physics-informed ML-based prediction model improved the performance of predicting the condition ratings of concrete decks by 10.0%. This research contributes to the body of knowledge by offering an ontology that can semantically represent structural deterioration knowledge in mathematical physics models to enable automated model instantiation. This research thus has the potential to fully unleash the power of the large amount of bridge data and deterioration knowledge that exist to develop next-generation learning machines for data-driven, physics-informed ML-based deterioration prediction and maintenance decision making.
更多查看译文
关键词
Mathematical physics models, Ontology, Physics-informed machine learning (ML), Bridge deterioration
AI 理解论文
溯源树
样例
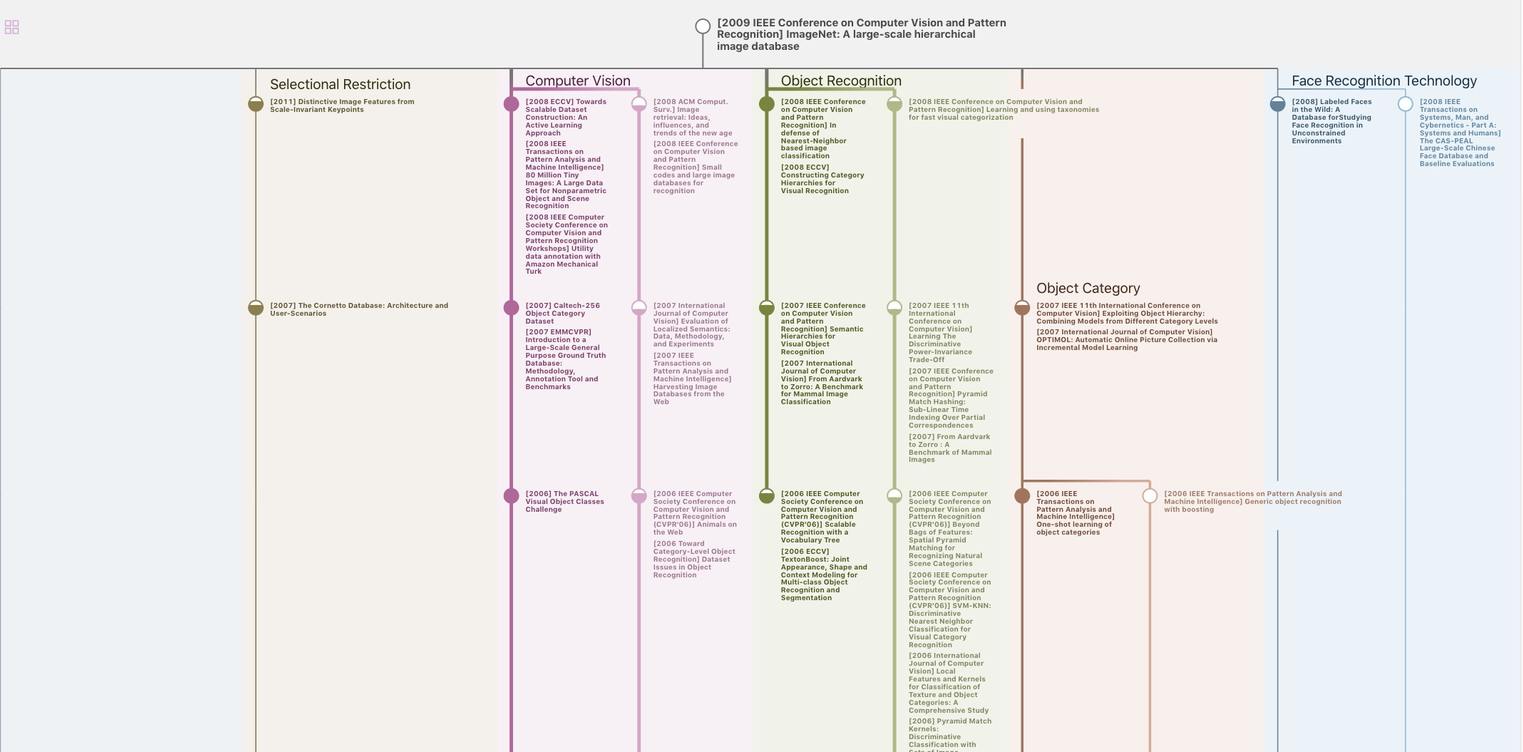
生成溯源树,研究论文发展脉络
Chat Paper
正在生成论文摘要