A strategy learning framework for particle swarm optimization algorithm
Information Sciences(2023)
摘要
Many variants with various strategies have been proposed to improve the efficiency of Particle Swarm Optimization (PSO) algorithm. These strategies are a precious resource waiting to be exploited. We conjecture that some new combinations of strategies selected from different PSO variants may better improve the performance of PSO. Inspired by this idea, this paper proposes a strategy learning framework to learn an optimal combination of strategies and thus derive a new PSO variant based on this combination. In this framework, a strategy pool with strategies selected from existing PSO variants is first constructed. Then, a training engine, implemented by an adaptive differential evolutionary algorithm, is employed to evaluate the performance of strategy combinations on training benchmark functions. Furthermore, a new PSO variant, named SLFPSO, is created based on the strategies learned from training results. This framework provides a novel method to design PSO variants by learning from existing algorithms through a learning mechanism. The performance and scalability of SLFPSO are compared with ten state-of-the-art PSO variants on 10/30/50/100-dimensional CEC2013/2014/2017 benchmark functions. The results verify that SLFPSO performs significantly better than the compared algorithms in most test scenarios.
更多查看译文
关键词
Particle swarm optimization,Strategy learning framework,Strategy pool,Training engine
AI 理解论文
溯源树
样例
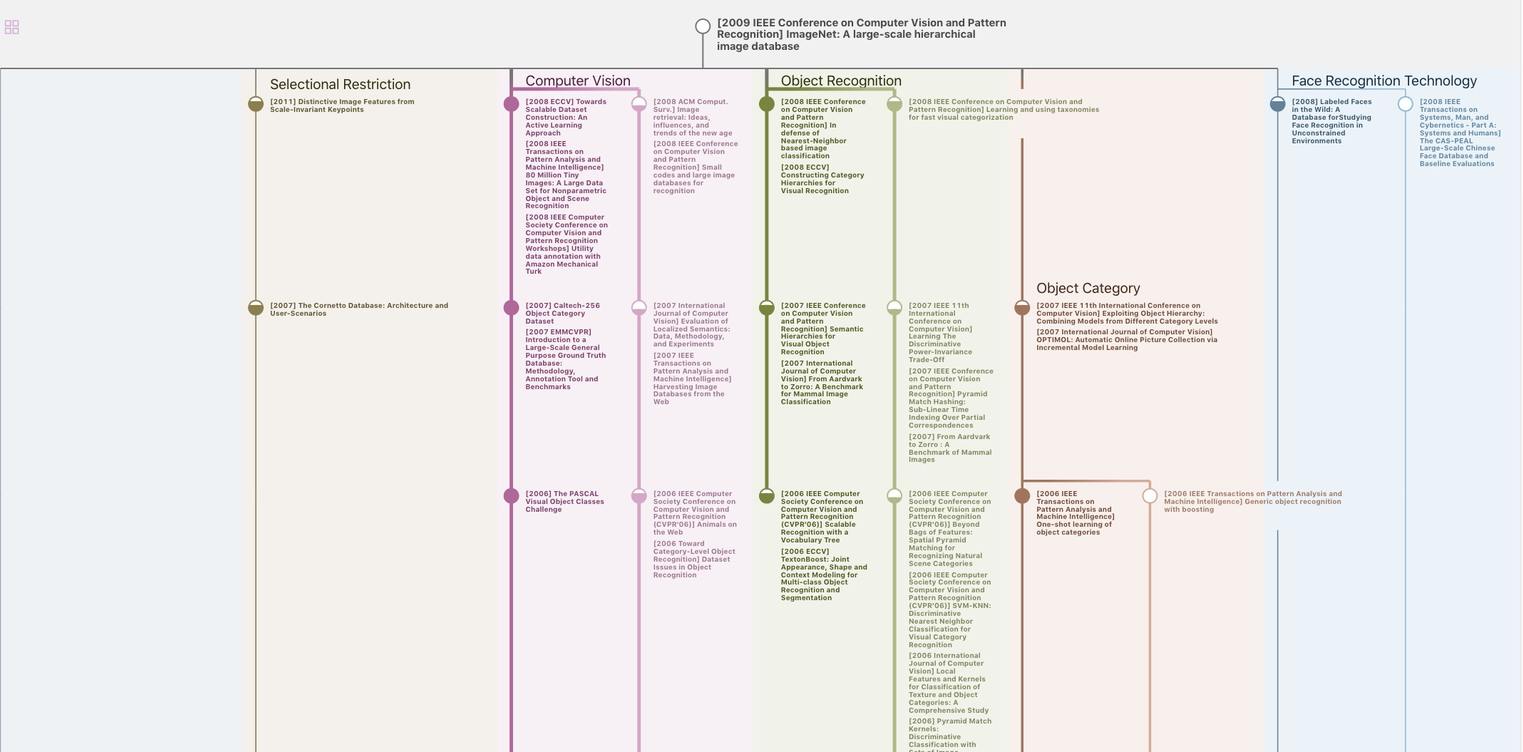
生成溯源树,研究论文发展脉络
Chat Paper
正在生成论文摘要