Few-mode fiber design for multiple-input-multiple-output-less mode division multiplexing by machine learning
JOURNAL OF THE OPTICAL SOCIETY OF AMERICA B-OPTICAL PHYSICS
摘要
Neural networks (NNs), random forests (RF), and extreme gradient boosting (XGBoost) are applied separately to inversely design the ring-core few-mode fiber (FMF) with a desired weakly coupled performance. We demonstrate the procedure of inverse designing of FMF via machine learning (ML) algorithms and evaluate the prediction accuracy of the above ML algorithms. Compared with RF and XGBoost, the NN performs the highest prediction accuracy. For the NN, RF, and XGBoost, the correlation coefficients of the mode effective index difference are 0.99993, 0.99857, and 0.99937, respectively. Subsequently, by utilizing the method of permuting feature importance ranking, we obtain the high-correlation fiber structural features with the mode effective index difference. Moreover, we analyze the effect of the minimum index difference between two adjacent modes (Delta n(eff.min)) on the structural parameters and get consistent feature attribution via permuting feature importance ranking above. Finally, we design a weakly coupled ring-core fiber that supports four modes (HE11, TE01, HE21, TM01) based on the NN algorithm, which could be successfully applied in vector mode division multiplexing. (c) 2022 Optica Publishing Group
更多查看译文
AI 理解论文
溯源树
样例
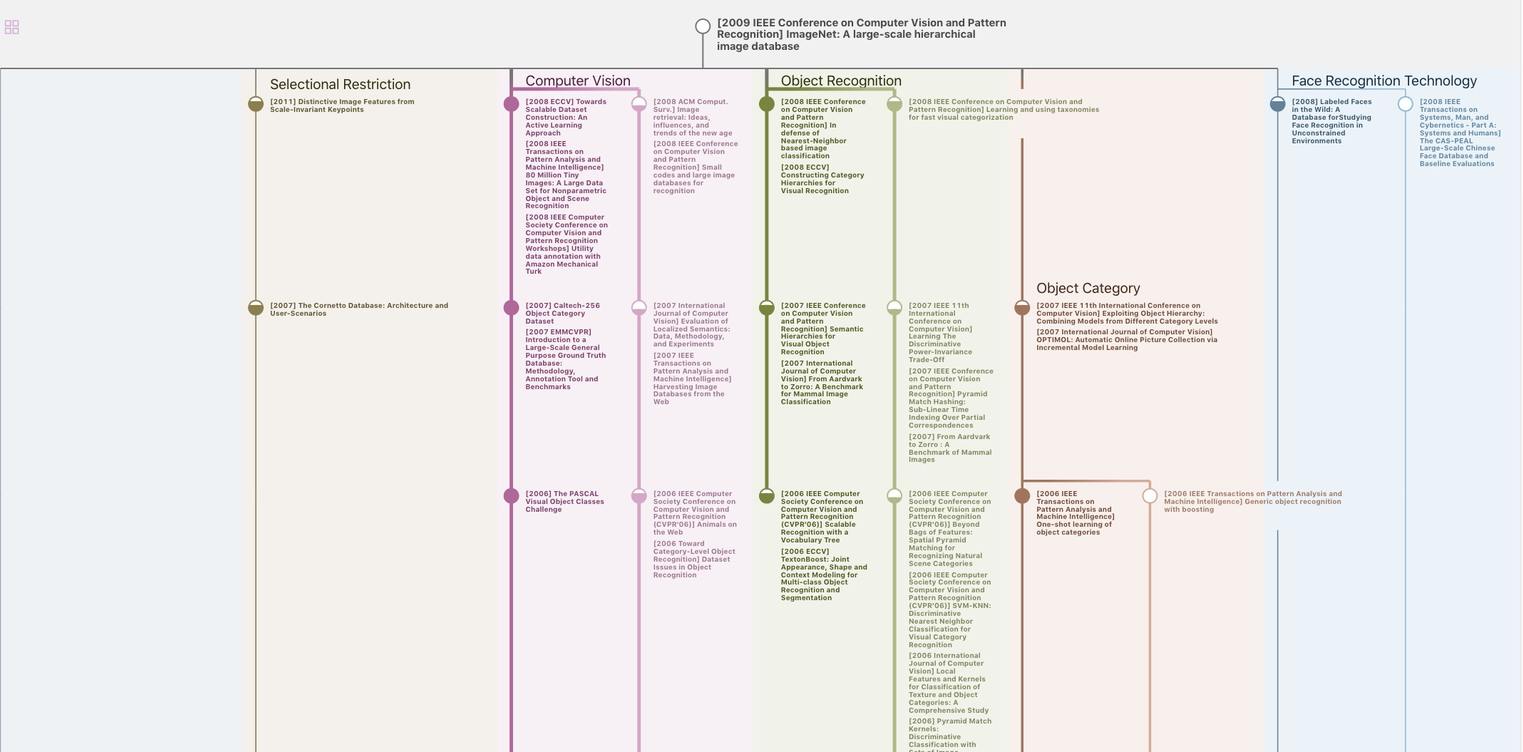
生成溯源树,研究论文发展脉络
Chat Paper
正在生成论文摘要