Low-carbon economic dispatch of the combined heat and power-virtual power plants: A improved deep reinforcement learning-based approach
IET RENEWABLE POWER GENERATION(2023)
摘要
To realize the national strategies for carbon emission reduction, electric power industries should undergo reforms to cope with the multiple challenges of decarbonization, marketization, and energy transition. How to design a dispatch strategy that considers both low-carbon demand and economic cost has become a major concern in integrated energy systems. To realize multi-energy complementation and low-carbonization, a scheduling framework of combined heat and power-virtual power plants (CHP-VPPs) considering carbon capture and power-to-gas is proposed. The stochastic dynamic optimization problem is modelled as a Markov decision process. An improved deep reinforcement learning-based algorithm named multi-level backtracking prioritized experience replay-twin delayed deep deterministic policy gradient (MBEPR-TD3) is employed to solve the low-carbon economic dispatch problem. The results show that: (1) The profits have been increased by 85.8% and carbon emissions have been reduced by 30.3% because of the addition of power-to-gas in CHP-VPP; (2) the revenues have been improved by 22.24% and carbon emissions have been decreased by 37.04% owing to the introduction of carbon capture and trading; (3) compared with results using DQN, DDPG, and TD3, the optimal dispatch strategy obtained by MBEPR-TD3 has higher reward returns and more stable convergence.
更多查看译文
关键词
artificial intelligence, cogeneration, energy conservation, energy management systems, intelligent control, power distribution planning, power system control, renewable energy sources
AI 理解论文
溯源树
样例
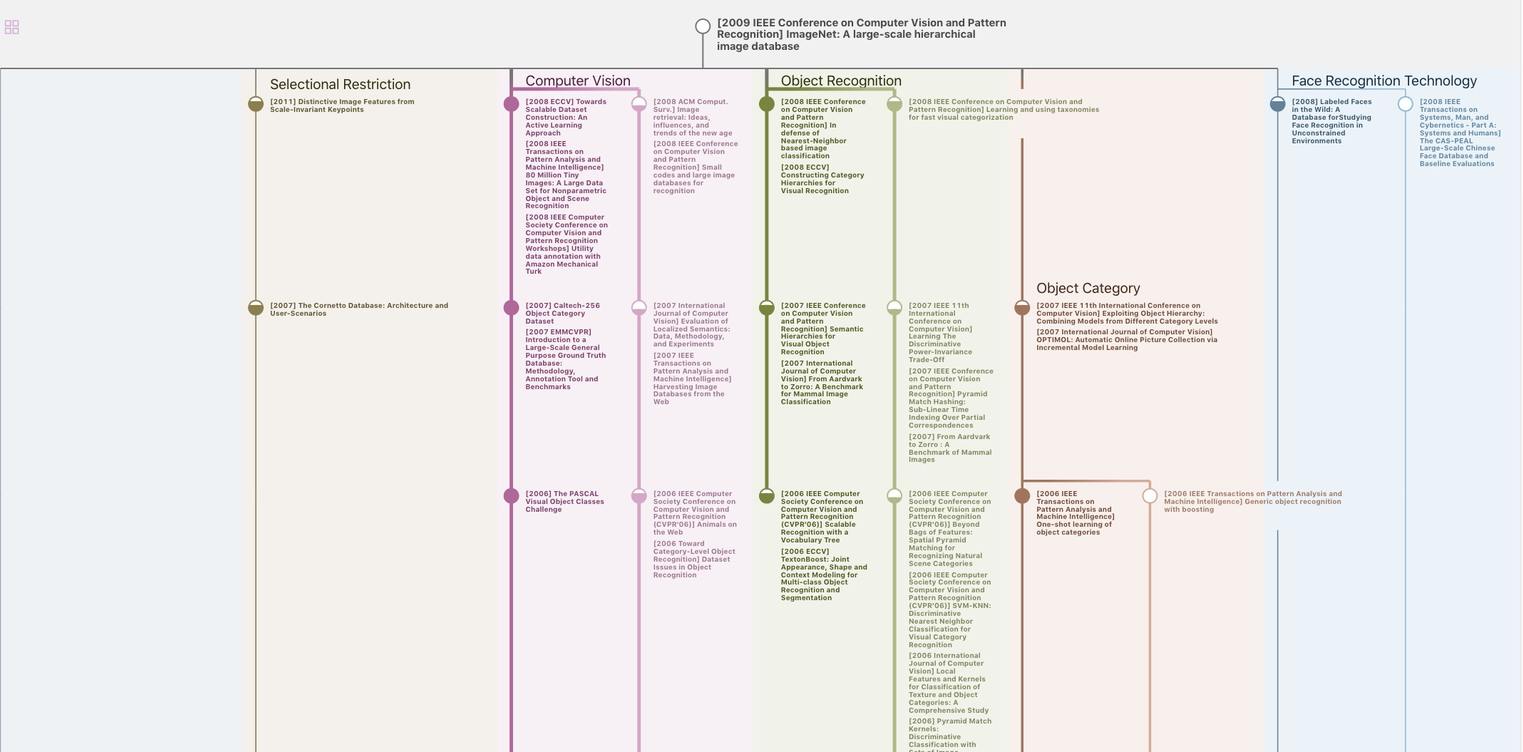
生成溯源树,研究论文发展脉络
Chat Paper
正在生成论文摘要