High-Throughput Fluorescent Screening and Machine Learning for Feature Selection of Electrocatalysts for the Alkaline Hydrogen Oxidation Reaction
ACS SUSTAINABLE CHEMISTRY & ENGINEERING(2022)
摘要
A parallel fluorescent screening method was used to evaluate active catalysts for the alkaline hydrogen oxidation reaction (HOR). A library of 1584 catalyst samples containing single element, binary, and ternary combinations was prepared in high-throughput fashion from 12 elements (Pt, Ag, Au, Co, Cu, Fe, Hg, Ni, Pb, Pd, Rh, and Sn) and was screened for their HOR onset potentials in an alkaline electrolyte. One of the most active alloys, Pt6Sn4, was tested in an alkaline polymer membrane fuel cell and produced a power density of 132 mW/(cm(2)center dot mg of Pt) compared with 103 mW/(cm(2)center dot mg of Pt) for a Pt/C reference catalyst. The compositions, morphologies, surface chemistries, and atomic structures of the catalysts were characterized to better understand the trends in their properties. The HOR onset potentials measured in the screening experiments were then used to create a database that was combined with elemental descriptors to train several machine learning models. The most accurate models were used to predict new alloy catalysts and rank the importance of each feature in the data set.
更多查看译文
关键词
Alloy electrocatalyst, Hydrogen oxidation, Alkaline fuel cell, High-throughput screening, Machine learning, Activity descriptor
AI 理解论文
溯源树
样例
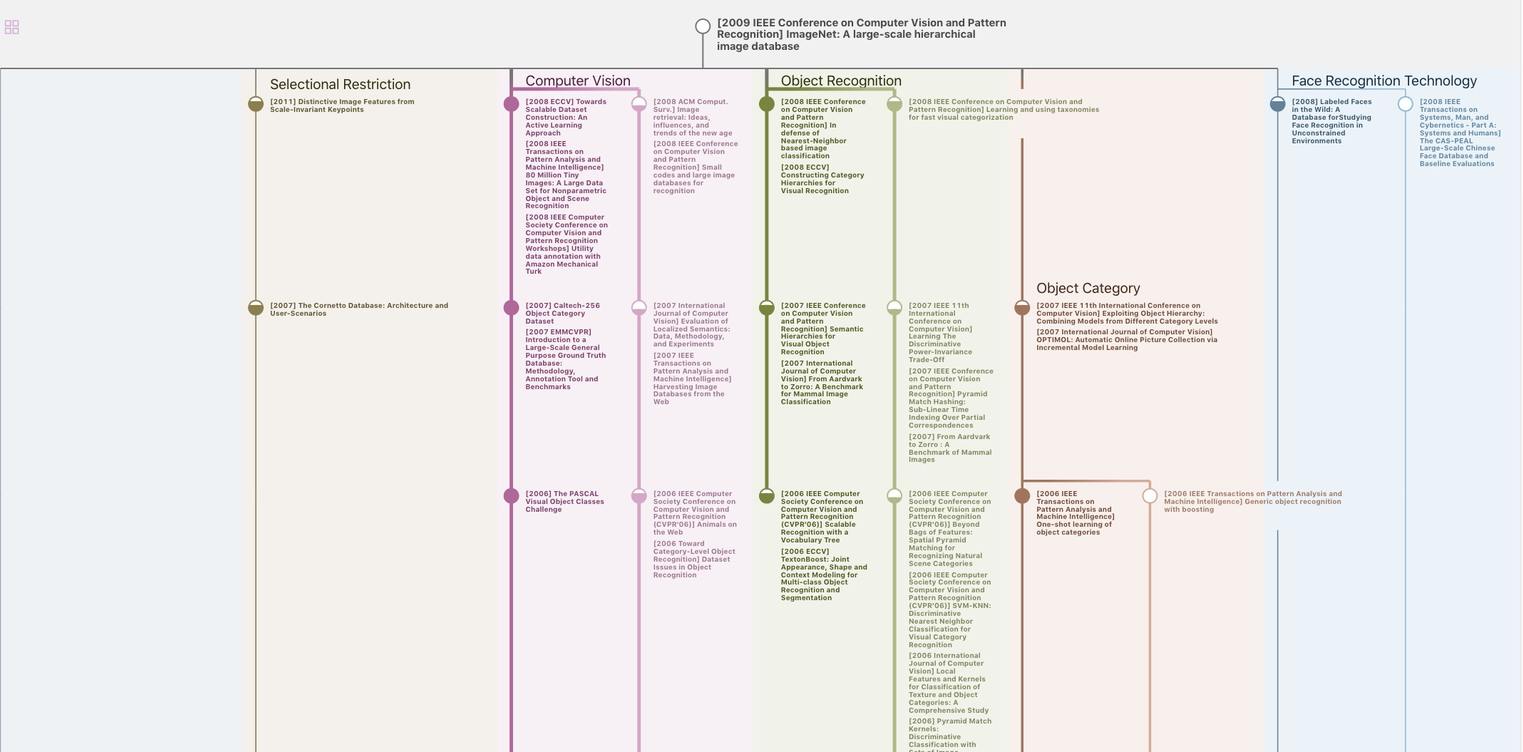
生成溯源树,研究论文发展脉络
Chat Paper
正在生成论文摘要