Learning neighborhood-based embedding sequence for link prediction in temporal knowledge graphs
JOURNAL OF INTELLIGENT & FUZZY SYSTEMS(2022)
摘要
Many real-world knowledge graphs are complex and keep evolving over time. Inferring missing facts in temporal knowledge graphs is a fundamental and challenging task. Previous studies focus on link prediction in static knowledge graphs which hardly extracts the temporal features effectively. In this paper, we propose a novel deep learning model, namely KBGAT-BiLSTM, which is capable of solving long-term predict problems and is suitable for temporal knowledge graph with complex structures. First, we adapt the Graph Attention Network (GAT) to learn the structural features of knowledge graph. Then we utilize the Bidirectional Long Short-Term Memory Networks (BiLSTM) to learn the temporal features and obtain the low-dimensional embeddings of entities and relations. Finally, we employ a scoring function for link prediction in temporal knowledge graphs. Through extensive experiments on YAGO, WIKI, and ICEWS18 datasets, we demonstrate the effectiveness of our model, compare the performance of our model with several different state-of-the-art methods and further analyze the properties of the proposed method.
更多查看译文
关键词
Knowledge graph, link prediction, graph attention network, temporal
AI 理解论文
溯源树
样例
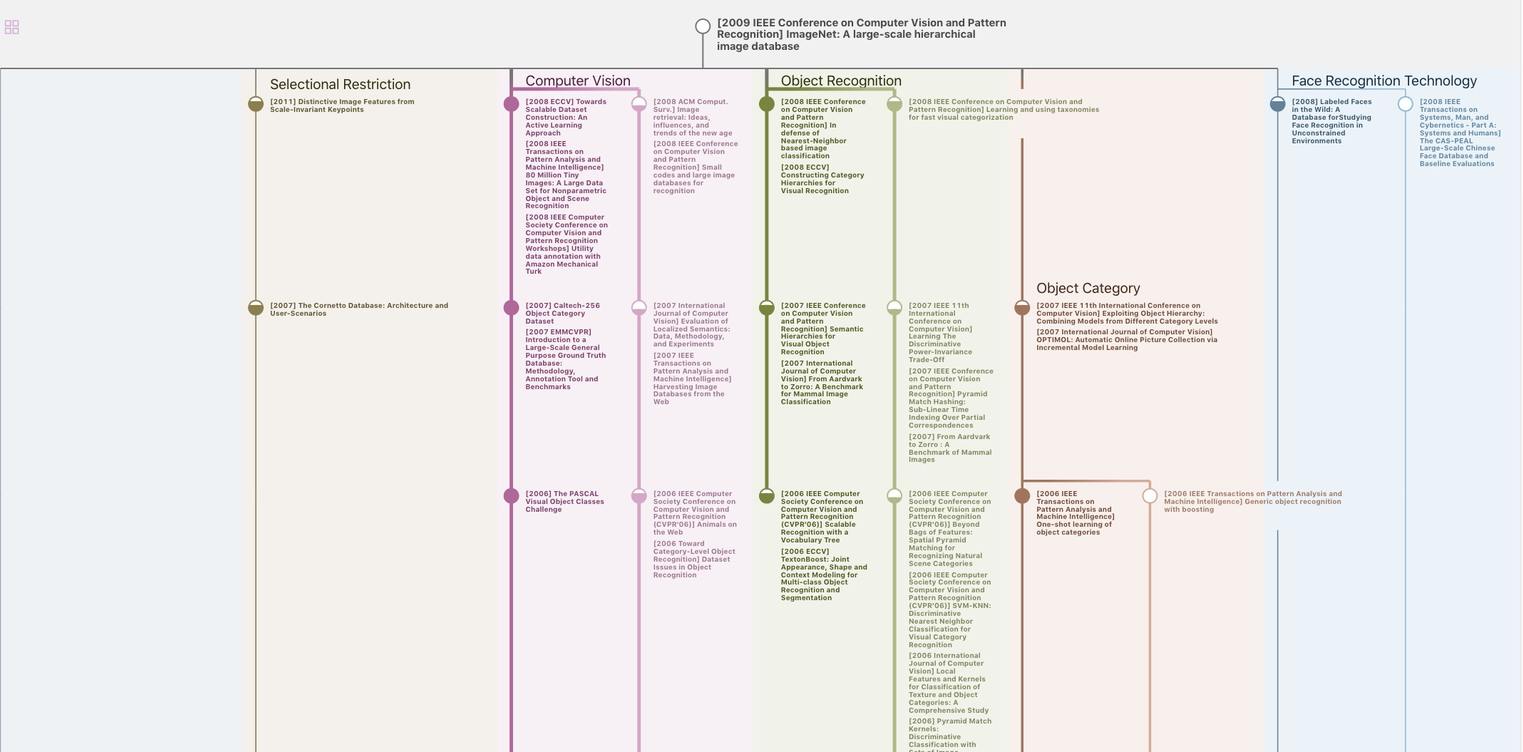
生成溯源树,研究论文发展脉络
Chat Paper
正在生成论文摘要