Development of Deep Residual Neural Networks for Gear Pitting Fault Diagnosis Using Bayesian Optimization
IEEE TRANSACTIONS ON INSTRUMENTATION AND MEASUREMENT(2022)
摘要
In recent years, the application of deep neural networks containing directed acyclic graph (DAG) architectures in mechanical fault diagnosis has achieved remarkable results. In order to improve the fault diagnosis ability of the networks, researchers have been working on developing new network architectures and optimizing the training process. However, this approach requires sufficient time and empirical knowledge to try a potential optimal framework. Furthermore, it is time-consuming and laborious to retune the network architecture and hyperparameter values when faced with different operating conditions or diagnostic tasks. To avoid these drawbacks, this article proposes an automated network architecture search (NAS) method and performs hyperparameter optimization. The adjacency matrix is used to define the architecture search space, the Bayesian optimization is used as the architecture search strategy, and a network test error is used for architecture evaluation. Seven types of convolutional layers and pooling layers are used as basic components to build fault diagnosis models. The gear pitting fault experiment, including seven gear pitting types, was established and used to validate the diagnostic model. The experimental results show that the diagnostic results of the network model automatically constructed by the proposed method are better than those of the general network model. It can be concluded that the proposed method can indeed replace the manual construction of an effective and practical gear pitting fault diagnosis model.
更多查看译文
关键词
Bayesian optimization, directed acyclic graph (DAG) network, gear pitting faults, hyperparameter optimization, network architecture search (NAS)
AI 理解论文
溯源树
样例
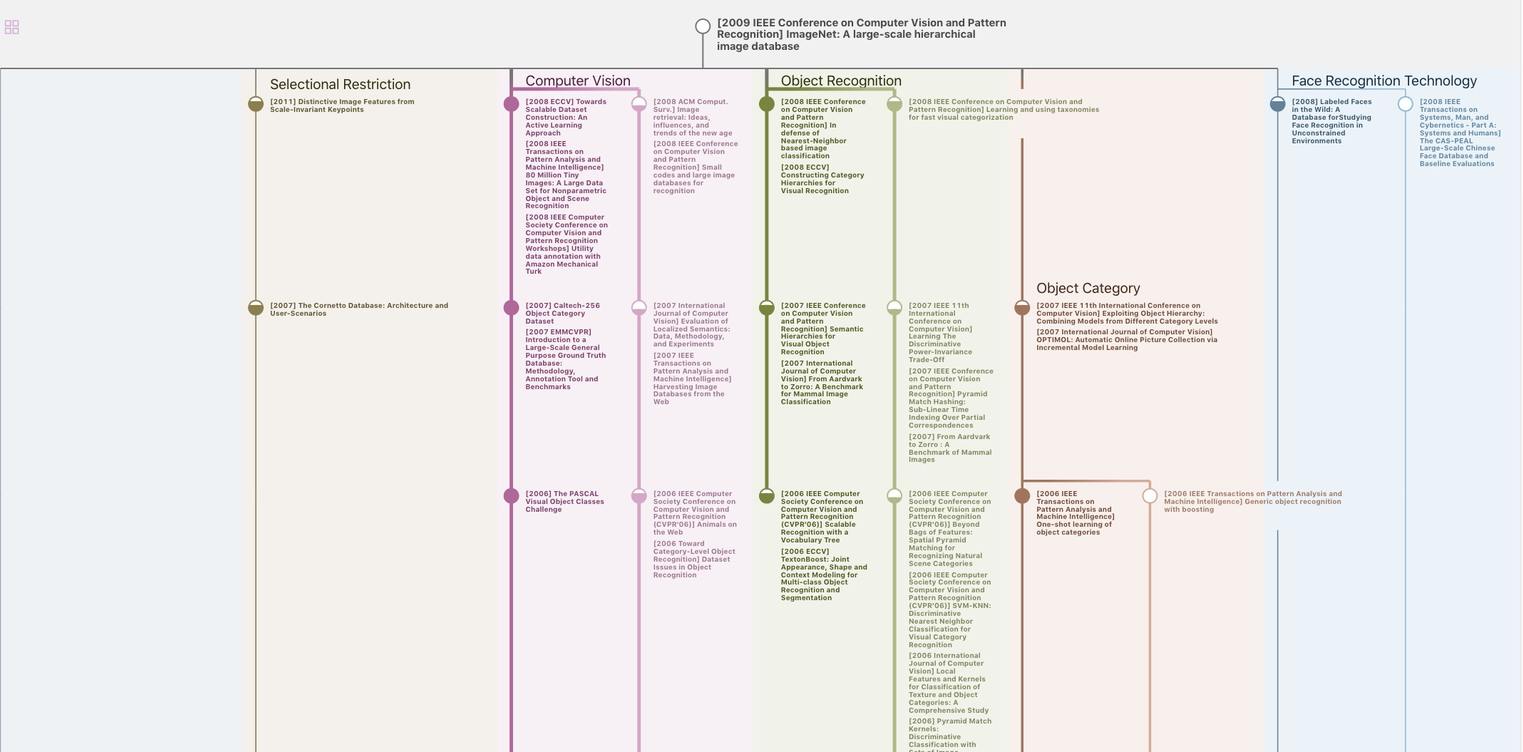
生成溯源树,研究论文发展脉络
Chat Paper
正在生成论文摘要