Noise-resistant multilabel fuzzy neighborhood rough sets for feature subset selection
Information Sciences(2023)
摘要
Feature selection attempts to capture the more discriminative features and plays a significant role in multilabel learning. As an efficient mathematical tool to handle incomplete and uncertain information, fuzzy rough set theory has been widely used in feature selection. However, there are two pivotal issues to be addressed when adopting fuzzy rough sets for multilabel data analysis. One is how to effectively characterize the discriminative ability of the features to multilabel samples. Another one is how to alleviate the effect of data noise on feature selection. For these reasons, this study mainly studies the noise-tolerant fuzzy neighborhood rough set model for multilabel learning and its feature selection strategy. Firstly, a parameterized hybrid fuzzy similarity relation is introduced to granulate multilabel data, and the parameterized fuzzy decision is extended to multilabel learning. Then, a noise-resistant multilabel fuzzy neighborhood rough set model using inclusion relation is constructed for describing the discriminative ability of the features to multilabel samples. Furthermore, a noise-resistant heuristic feature selection algorithm titled NRFSFN is designed. Besides, motivated by local sampling and ensemble strategy, an efficient extended version of NRFSFN is designed from the perspective of data distribution, named ENFSFN. Finally, a series of comparison experiments on sixteen multilabel datasets demonstrate the effectiveness of the proposed two algorithms.
更多查看译文
关键词
Fuzzy neighborhood rough sets,Multilabel feature selection,Noise-resistant,Ensemble strategy
AI 理解论文
溯源树
样例
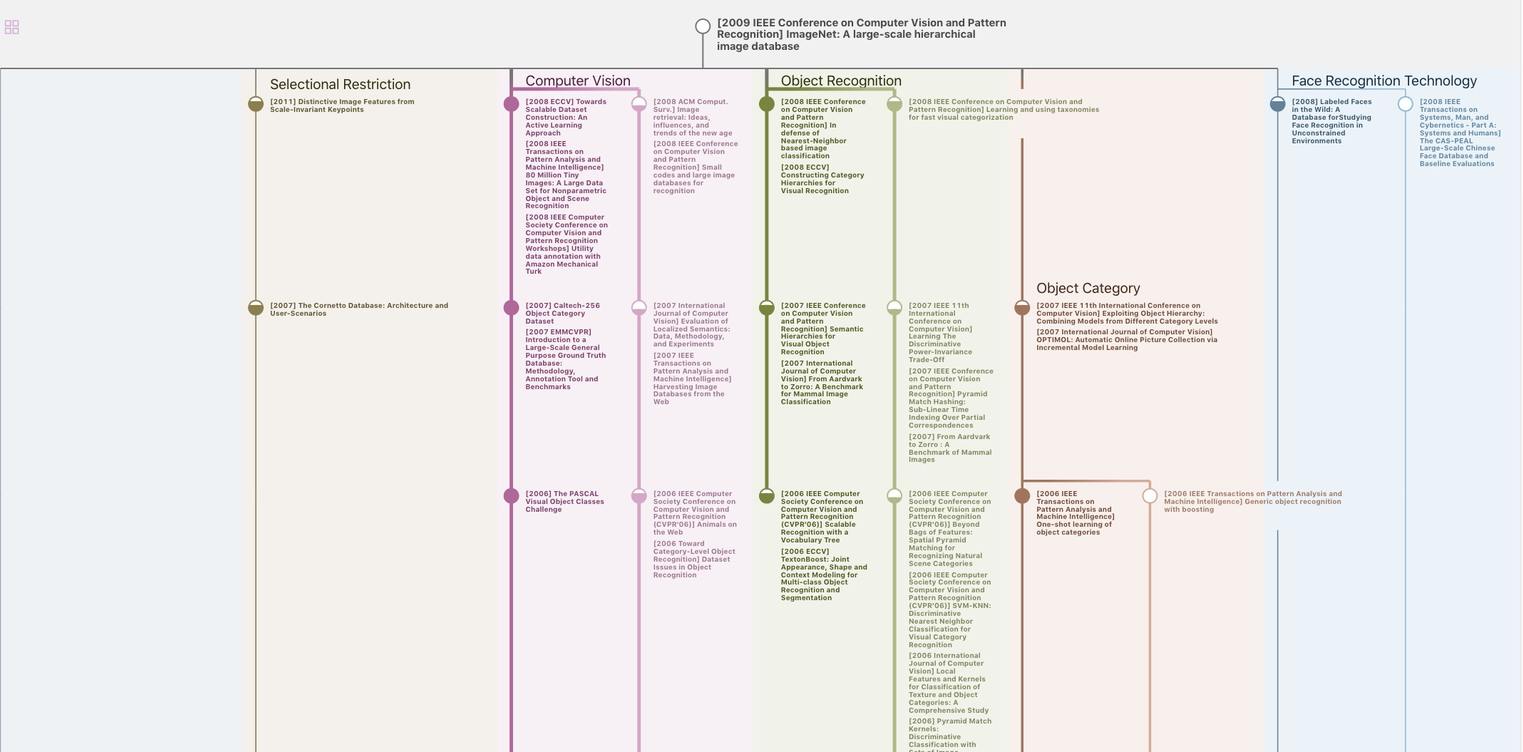
生成溯源树,研究论文发展脉络
Chat Paper
正在生成论文摘要