Dynamic traffic correlations based spatio-temporal graph convolutional network for urban traffic prediction
Information Sciences(2023)
摘要
Accurate urban traffic prediction is a critical issue in Intelligent Transportation Systems (ITS). It is challenging since urban traffic usually indicates high dynamic spatio-temporal correlations, leading to uncertainty and complexity of traffic status. Since the transporta-tion network is a graph structure practically, existing works have applied Graph Convolutional Network (GCN) on urban traffic prediction with a pre-defined adjacency matrix based on node distance or connectivity. However, in many urban traffic scenarios, spatio-temporal dependencies among traffic data usually change over time, so using a fixed adjacency matrix cannot describe the dynamic dependencies. To track the dynamic spatio-temporal dependencies among traffic data, we propose a novel deep learning framework, Dynamic Traffic Correlation-based Spatio-Temporal Graph Convolutional network (DTC-STGCN), to forecast traffic flow and speed accurately. DTC-STGCN extracts a dynamic adjacency matrix from different traffic characters to describe dynamic spatio-temporal cor-relations. Moreover, an attention and dynamic adjacency matrix-based GCNs framework is proposed to capture urban traffic dynamic spatial features, while a long-short-term mem-ory network (LSTM) is used to capture urban traffic temporal features, respectively. Finally, we feed the spatio-temporal features generated by GCN and LSTM, with real road segments into a hybrid graph convolution framework to simultaneously model the dynamic spatial and temporal dependencies for traffic predictions. The experiments on two real-world datasets demonstrate that the proposed DTC-STGCN model consistently outperforms the state-of-the-art traffic prediction baselines on MAE and RMSE over 10%, and achieve a stable performance for two specific tasks (long-term traffic prediction and peak time pre-diction). And ablation study validates the effectiveness of dynamic adjacency matrix, atten-tion mechanism, respectively. (c) 2022 Elsevier Inc. All rights reserved.
更多查看译文
关键词
Dynamic traffic correlations,Urban traffic prediction,Graph convolutional network,Long short term memory network,Attention networks
AI 理解论文
溯源树
样例
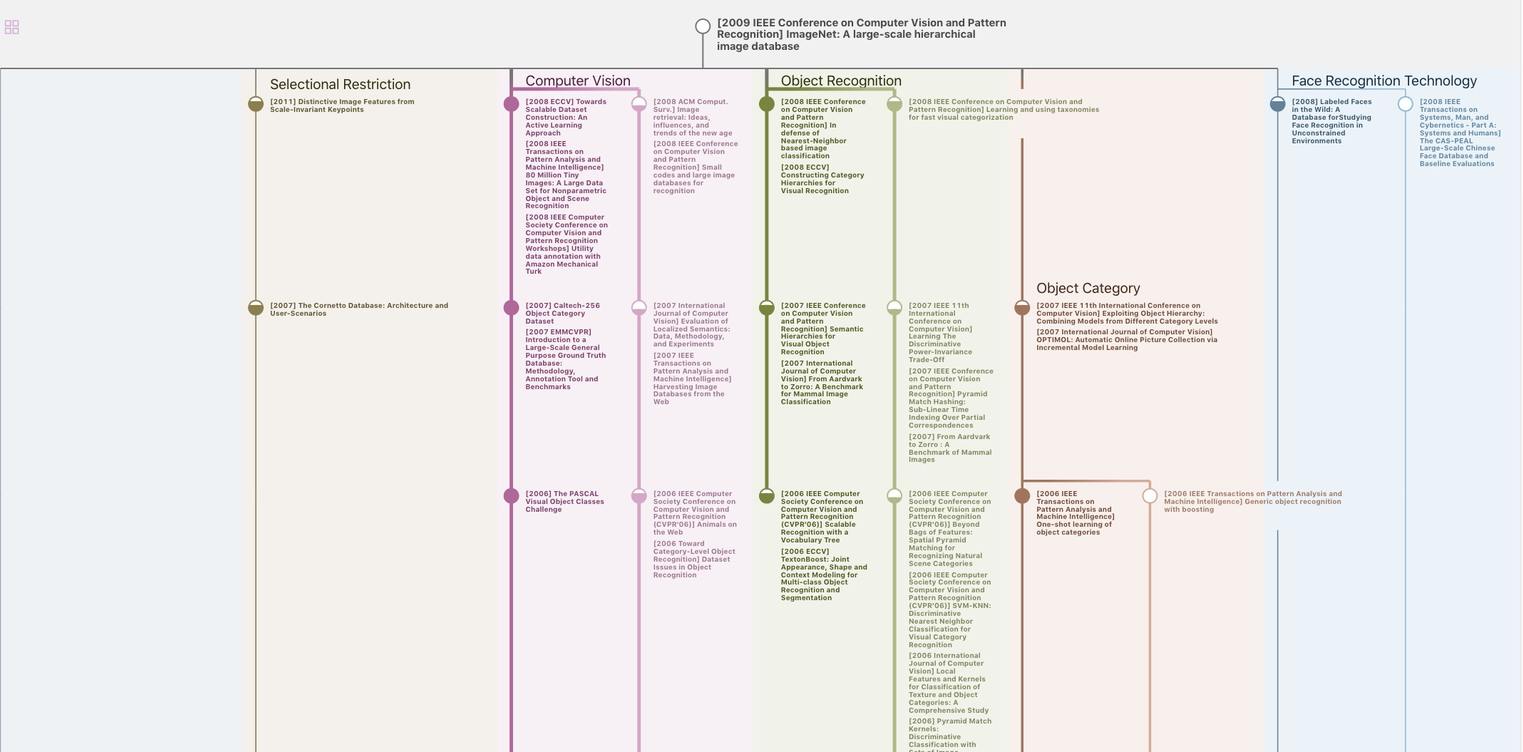
生成溯源树,研究论文发展脉络
Chat Paper
正在生成论文摘要