Enhancing differential evolution algorithm using leader-adjoint populations
Information Sciences(2023)
摘要
The performance of differential evolution (DE) significantly depends on the settings of mutation strategies and control parameters. Inappropriate settings may cause an imbalance between exploration and exploitation of the algorithm, thus resulting in two extremes: premature convergence and failure to converge. In this paper, we propose a Differential Evolution using Leader-Adjoint populations (LADE), which simultaneously integrates four mutation strategies to meet the needs of exploration and exploitation at different evolutionary stages. In LADE, the population in each generation is divided into leader population and adjoint population by using a leader-adjoint model. The leader population adopts two mutation strategies with strong exploration ability to maintain the diversity and avoid premature convergence, while the adjoint population uses the other two mutation strategies with strong exploitation ability to promote convergence and avoid stagnation. In addition, the interaction and collaboration between both populations are achieved through the sharing between their individuals, thus achieving a good trade-off between exploration and exploitation. The performance of LADE is evaluated on single-objective benchmark problems of the 2017 Institute of Electrical and Electronics Engineers Congress on Evolutionary Computation (IEEE CEC2017). Experimental results indicate that LADE shows competitive convergence performance, and outperforms various state-of-the-art DE variants and two well-known metaheuristics.
更多查看译文
关键词
Differential evolution,Leader-adjoint model,Population diversity,Mutation strategies,Convergence
AI 理解论文
溯源树
样例
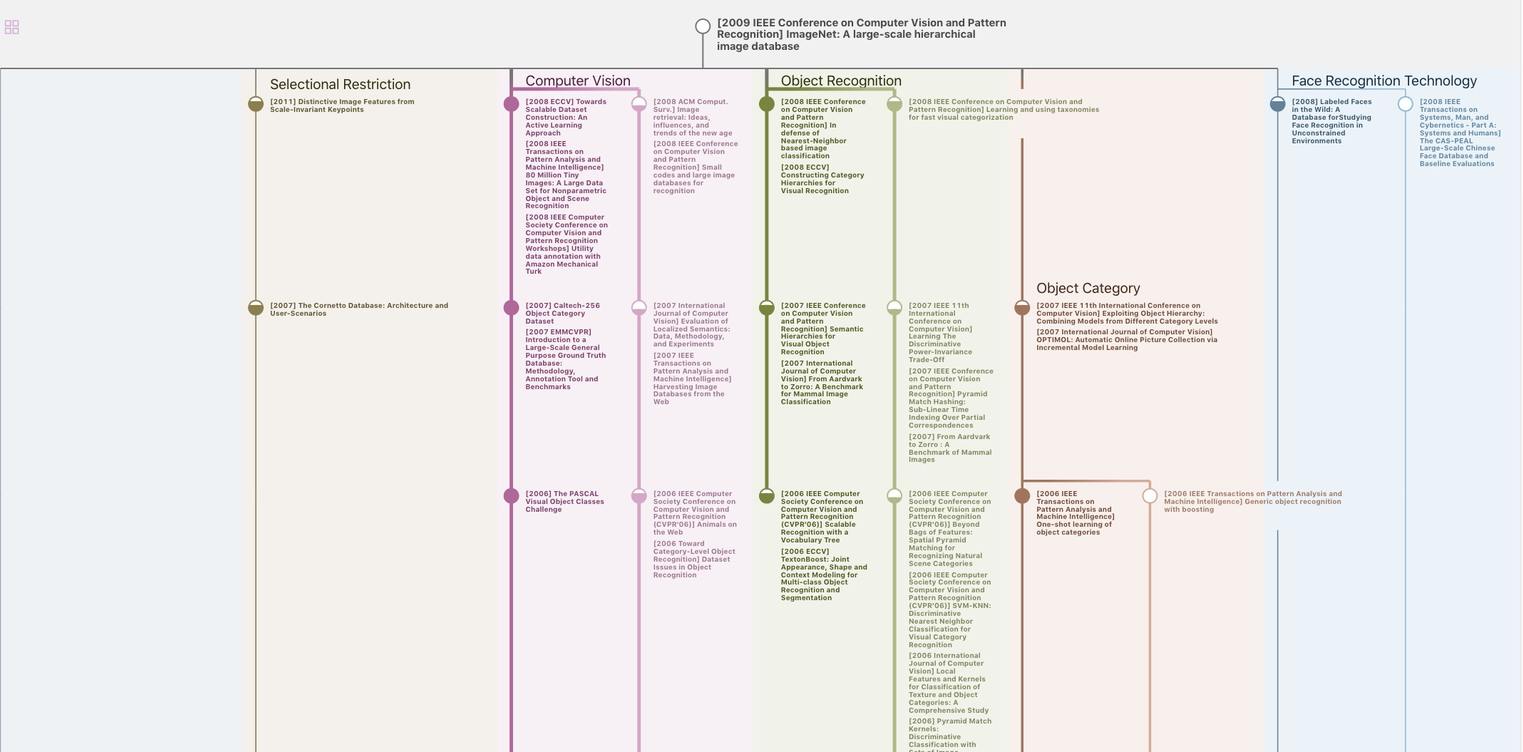
生成溯源树,研究论文发展脉络
Chat Paper
正在生成论文摘要