Map-enhanced generative adversarial trajectory prediction method for automated vehicles
Information Sciences(2023)
摘要
Trajectory prediction in dynamic and highly interactive scenarios is a critical method for achieving advanced autonomous driving. Maximizing the guidance and constraints pro-vided by high-definition (HD) maps can help improve prediction performance across the board. In this paper, we propose a map-enhanced generative adversarial network (ME -GAN) for vehicle trajectory prediction. The vehicle motion features, map constraints, traffic flow density, and vehicle interactions are comprehensively considered in the generator, and a graph query mechanism is proposed to realize the reuse of the global map. In the dis-criminator, in addition to considering the authenticity of a generated trajectory and whether it is consistent with the historical trajectory, additional map information is intro-duced to establish a matching model between the generated trajectory and the current map. Experiments based on the Argoverse and nuScenes dataset are subsequently per -formed. The experimental results show that our prediction method outperforms state-of -the-art prediction systems, namely, TNT, PRIME and P2T. The strong coupling of the HD map significantly improves the reasonableness of the predicted trajectory.CO 2022 Elsevier Inc. All rights reserved.
更多查看译文
关键词
Vehicle trajectory prediction,Generative adversarial network,HD map,Graph convolution,Map-enhanced
AI 理解论文
溯源树
样例
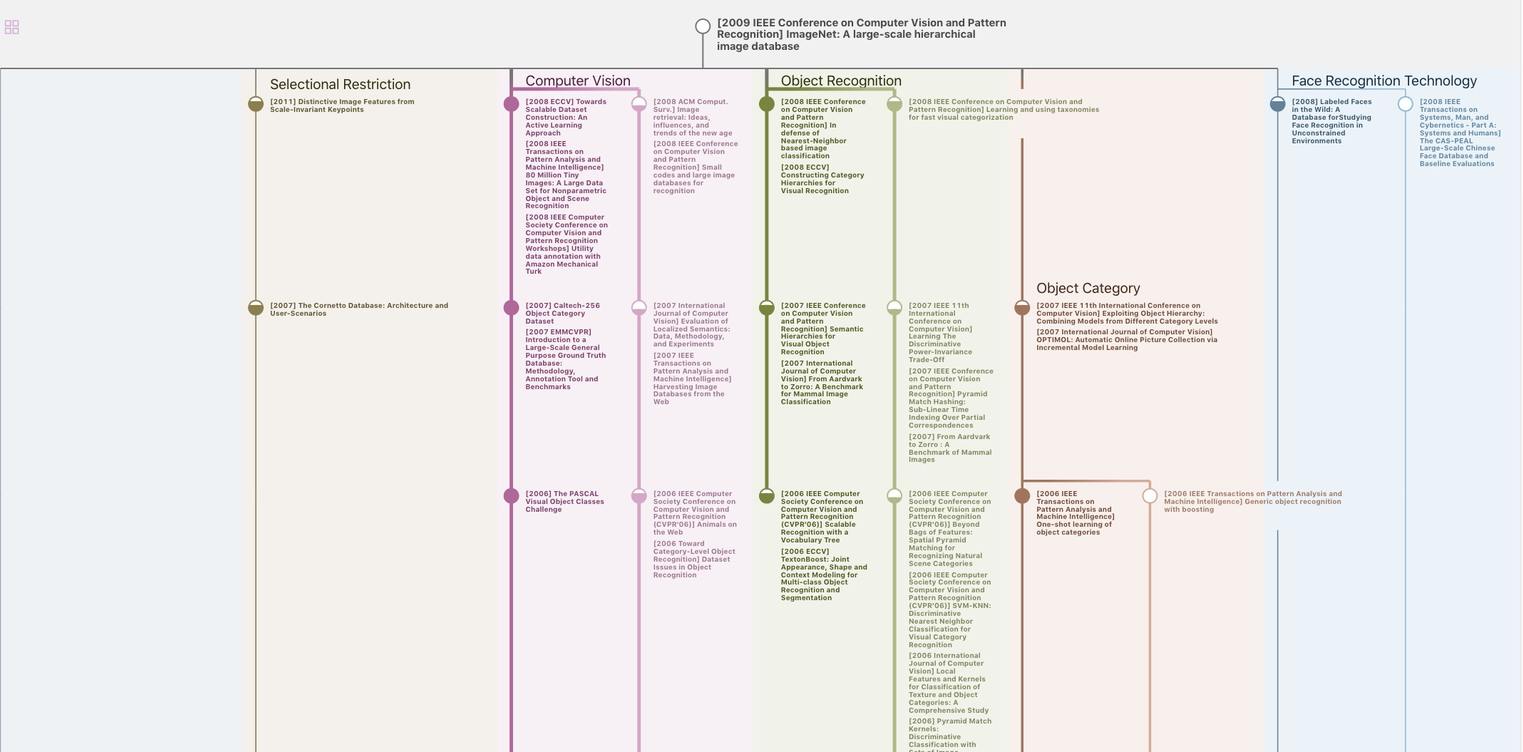
生成溯源树,研究论文发展脉络
Chat Paper
正在生成论文摘要