Ensembled masked graph autoencoders for link anomaly detection in a road network considering spatiotemporal features
Information Sciences(2023)
摘要
Road anomaly detection aims to find a small group of roads that are exceptional with respect to the rest of the physical links in a transportation network, posing great challenges for spatial data mining and urban infrastructure management applications. Road links involve complex features from both spatial and temporal perspectives, but existing detec-tion methods mostly rely on the topological features of road networks because they face difficulty in capturing the spatial and temporal characteristics of road networks. To over-come this difficulty, we constructed an ensembled autoencoder framework for road anom-aly detection by combining spatiotemporal graph learning and graph masking methods, and our main focus was to effectively detect the topological and attribute anomalies mapped by spatiotemporal features in a road network based on traffic flow data. To model local contexts, we performed random masking on the input graph to obtain heterogeneous masked subgraphs. On this basis, a spatiotemporal graph learning model was used to extract topological and traffic flow features from masked subgraphs. Then, we carried out feature reconstructions for each subgraph and calculated the anomaly scores for every node in the graph. Finally, an ensemble model was designed to integrate the anomaly detection results from multiple autoencoders that were trained on the subgraphs to improve feature learning. Our experimental results show that the proposed method is superior to traditional topological analysis methods, machine learning methods (DBScan, isolation forest, LOF, and one-class SVM), and recent deep learning methods (GAE and VGAE).(c) 2022 Elsevier Inc. All rights reserved.
更多查看译文
关键词
Anomaly detection,Autoencoder,Deep learning,Road network
AI 理解论文
溯源树
样例
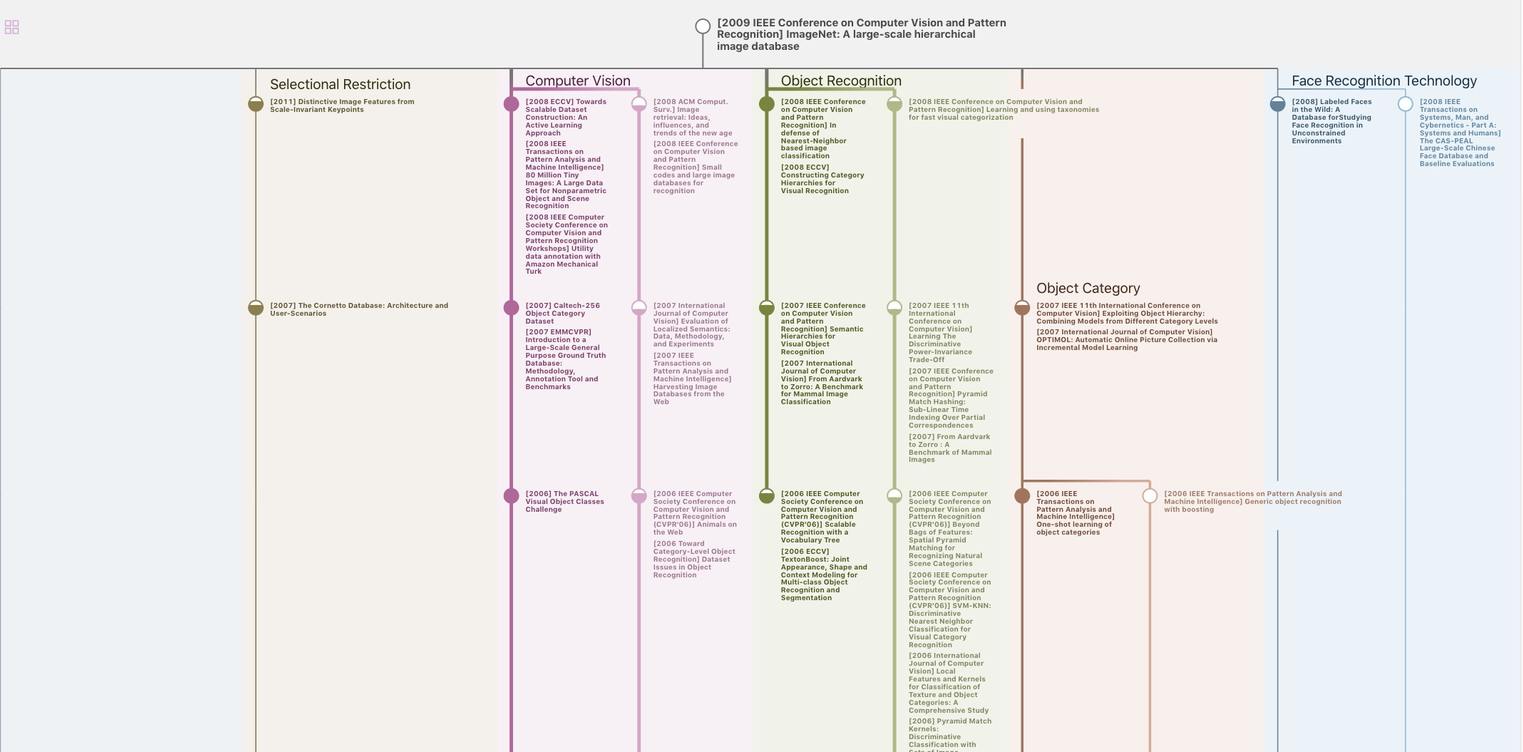
生成溯源树,研究论文发展脉络
Chat Paper
正在生成论文摘要