Dispersion-enhanced sequential batch sampling for adaptive contour estimation
QUALITY AND RELIABILITY ENGINEERING INTERNATIONAL(2024)
摘要
In computer simulation and optimal design, sequential batch sampling offers an appealing way to iteratively stipulate optimal sampling points based upon existing selections and efficiently construct surrogate modeling. Nonetheless, the issue of near duplicates poses tremendous quandary for sequential learning. It refers to the situation that selected critical points cluster together in each sampling batch, which are individually but not collectively informative towards the optimal design. Near duplicates severely diminish the computational efficiency as they barely contribute extra information towards update of the surrogate. To address this issue, we impose a dispersion criterion on concurrent selection of sampling points, which essentially forces a sparse distribution of critical points in each batch, and demonstrate the effectiveness of this approach in adaptive contour estimation. Specifically, we adopt Gaussian process surrogate to emulate the simulator, acquire variance reduction of the critical region from new sampling points as a dispersion criterion, and combine it with the modified expected improvement (EI) function for critical batch selection. The critical region here is the proximity of the contour of interest. This proposed approach is vindicated in numerical examples of a two-dimensional four-branch function, a four-dimensional function with a disjoint contour of interest and a time-delay dynamic system.
更多查看译文
关键词
Batch sampling,contour estimation,Gaussian process surrogate,sequential optimal design
AI 理解论文
溯源树
样例
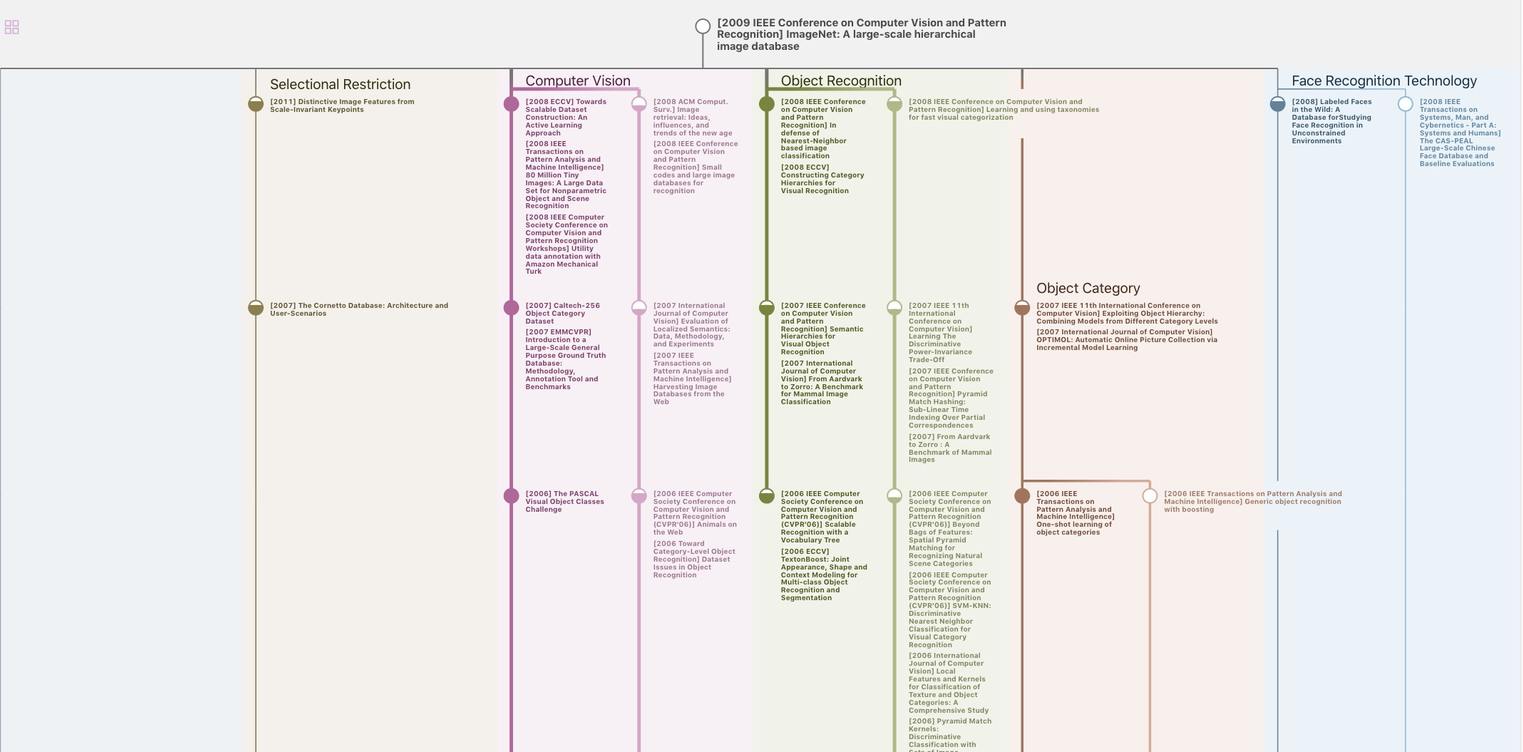
生成溯源树,研究论文发展脉络
Chat Paper
正在生成论文摘要