Shape-Former: Bridging CNN and Transformer via ShapeConv for multimodal image matching
Information Fusion(2023)
摘要
As with any data fusion task, the front-end of the pipeline for image fusion, aiming to collect multitudinous physical properties from multimodal images taken by different types of sensors, requires registering the overlapped content of two images via image matching. In other words, the accuracy of image matching will influence directly the subsequent fusion results. In this work, we propose a hybrid correspondence learning architecture, termed as Shape-Former, which is capable of solving matching problems such as multimodal, and multiview cases. Existing attempts have trouble capturing intricate feature interactions for seeking good correspondence, if the image pairs simultaneously suffer from geometric and radiation distortion. To address this, our key is to take advantage of convolutional neural network (CNN) and Transformer for enhancing structure consensus representation ability. Specifically, we introduce a novel ShapeConv so that CNN and Transformer can be generalized to sparse matches learning. Furthermore, we provide a robust soft estimation of outliers mechanism for filtering the response of outliers before capturing shape features. Finally, we also propose coupling multiple consensus representations to further solve the context conflict problems such as local ambiguity. Experiments with variety of datasets reveal that our Shape-Former outperforms state-of-the-art on multimodal image matching, and shows promising generalization ability to different types of image deformations.
更多查看译文
关键词
Feature matching,Deep learning,Shape-Former,Multimodal image matching,Registration and fusion
AI 理解论文
溯源树
样例
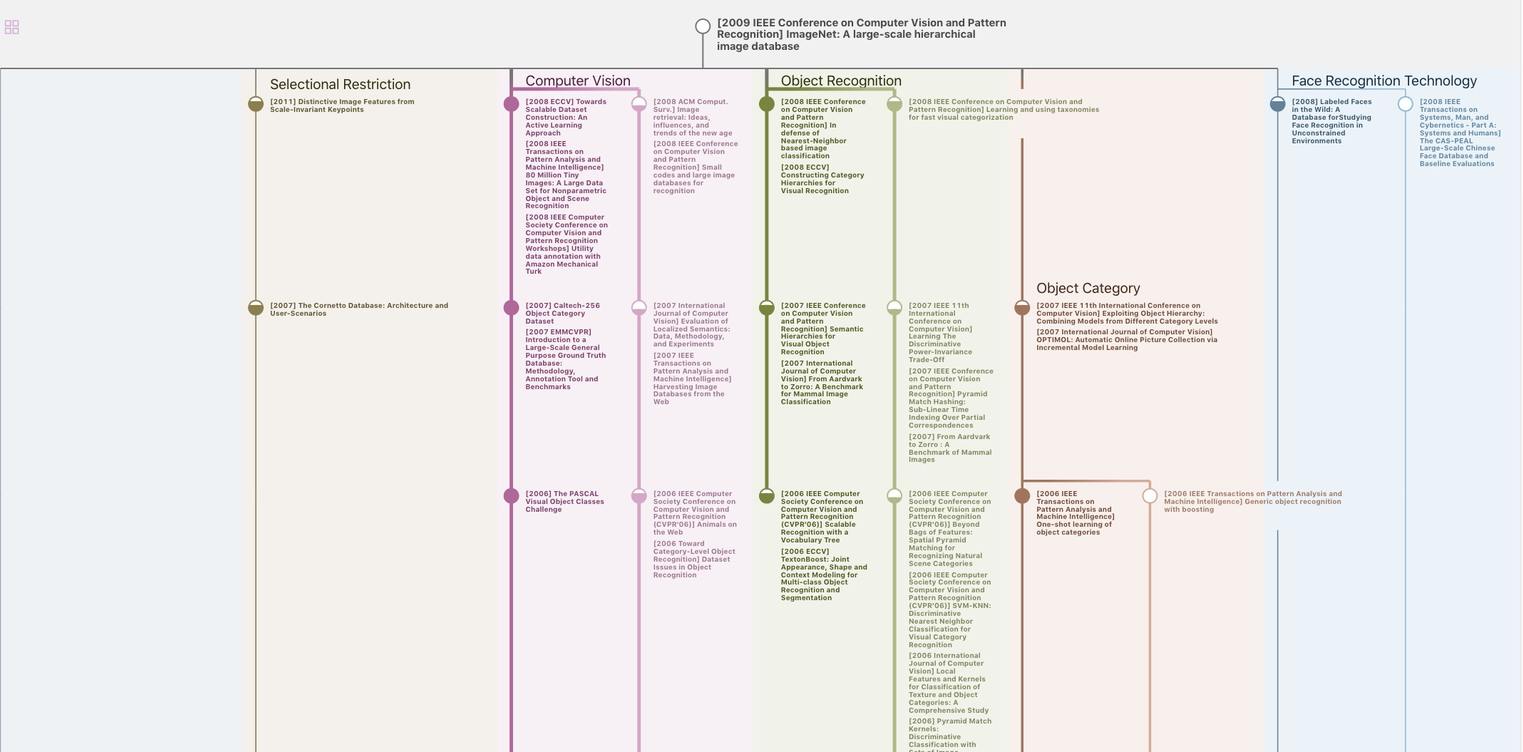
生成溯源树,研究论文发展脉络
Chat Paper
正在生成论文摘要