Secure Smart Communication Efficiency in Federated Learning: Achievements and Challenges
Applied Sciences(2022)
摘要
Federated learning (FL) is known to perform machine learning tasks in a distributed manner. Over the years, this has become an emerging technology, especially with various data protection and privacy policies being imposed. FL allows for performing machine learning tasks while adhering to these challenges. As with the emergence of any new technology, there will be challenges and benefits. A challenge that exists in FL is the communication costs: as FL takes place in a distributed environment where devices connected over the network have to constantly share their updates, this can create a communication bottleneck. This paper presents the state-of-the-art of the conducted works on communication constraints of FL while maintaining the secure and smart properties that federated learning is known for. Overall, current challenges and possible methods for enhancing the FL models’ efficiency with a perspective on communication are discussed. This paper aims to bridge the gap in all conducted review papers by solely focusing on communication aspects in FL environments.
更多查看译文
关键词
security,privacy,smart environments,federated learning,communication,machine learning
AI 理解论文
溯源树
样例
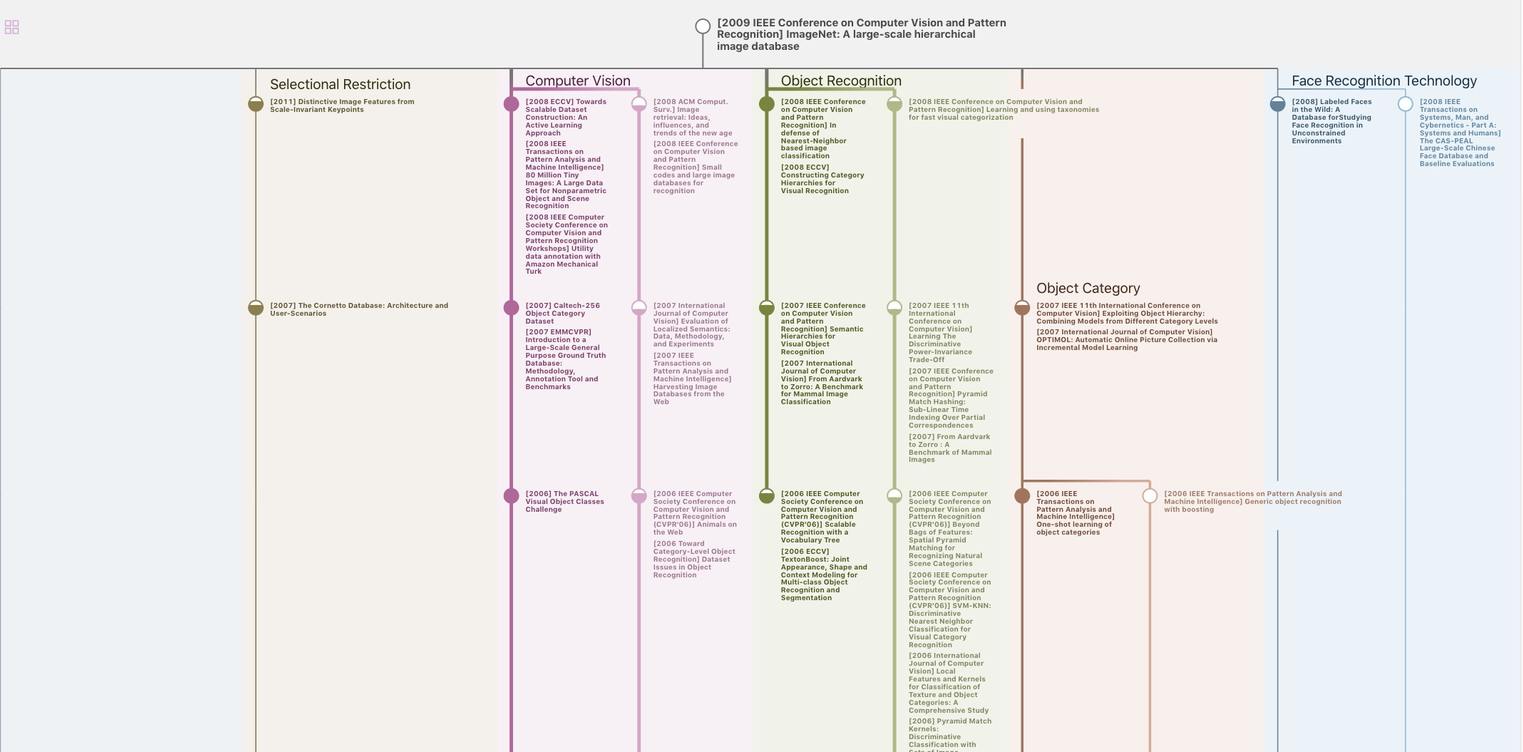
生成溯源树,研究论文发展脉络
Chat Paper
正在生成论文摘要