Weighted Random Forests for Evaluating Financial Credit Risk
Proceedings of Engineering and Technology Innovation(2019)
摘要
Credit evaluation of customers is a critical issue in financial organizations. Classification algorithms have been proposed for credit evaluation in recent years, and the class distribution in the financial data for those studies are not skewed. However, only a small proportion of customers will be the cases for bad credit. Financial records should be considered as an imbalanced data set for analyzing credit risk. Ensemble algorithms that make predictions by group decisions generally have relatively high accuracy than the ones inducing only one model from data. This study introduces a mechanism based on the weighted random forest to improve the prediction accuracy on the records with bad credit. This mechanism is tested on two financial data sets to demonstrate that it can achieve relatively high performance in evaluating credit risk and that the number of decision trees in a forest is not helpful. Critical attributes are also identified to provide practical meanings for credit risk analysis.
更多查看译文
关键词
credit risk analysis,decision tree,imbalanced data,random forest
AI 理解论文
溯源树
样例
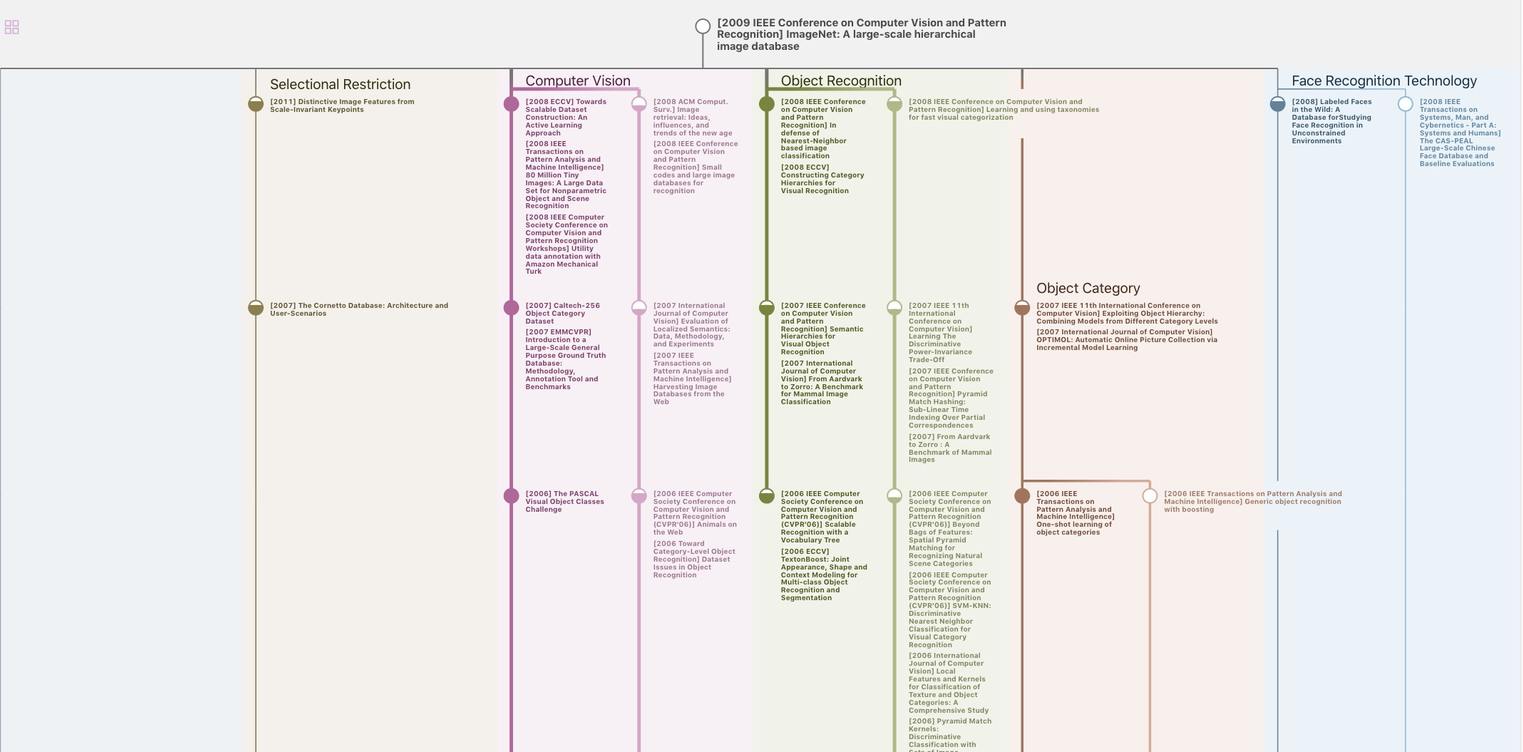
生成溯源树,研究论文发展脉络
Chat Paper
正在生成论文摘要