Using Under-Trained Deep Ensembles to Learn Under Extreme Label Noise: A Case Study for Sleep Apnea Detection
IEEE Access(2021)
摘要
Improper or erroneous labelling can pose a hindrance to reliable generalization for supervised learning. This can have negative consequences, especially for critical fields such as healthcare. We propose an effective new approach for learning under extreme label noise for medical applications like sleep apnea, that is based on under-trained deep ensembles. Each ensemble member is trained with a subset of the training data, to acquire a general overview of the decision boundary separation, without focusing on potentially erroneous details. The accumulated knowledge of the ensemble is combined to form new labels, that determine a better class separation than the original labels. A new model is trained with these labels to generalize reliably despite the label noise. We evaluate our approach on the tasks of sleep apnea detection and sleep apnea severity classification, and observe performance improvement in kappa from 0.02 up-to 0.55.
更多查看译文
关键词
Biomedical informatics,supervised learning,sleep apnea,machine learning,label noise
AI 理解论文
溯源树
样例
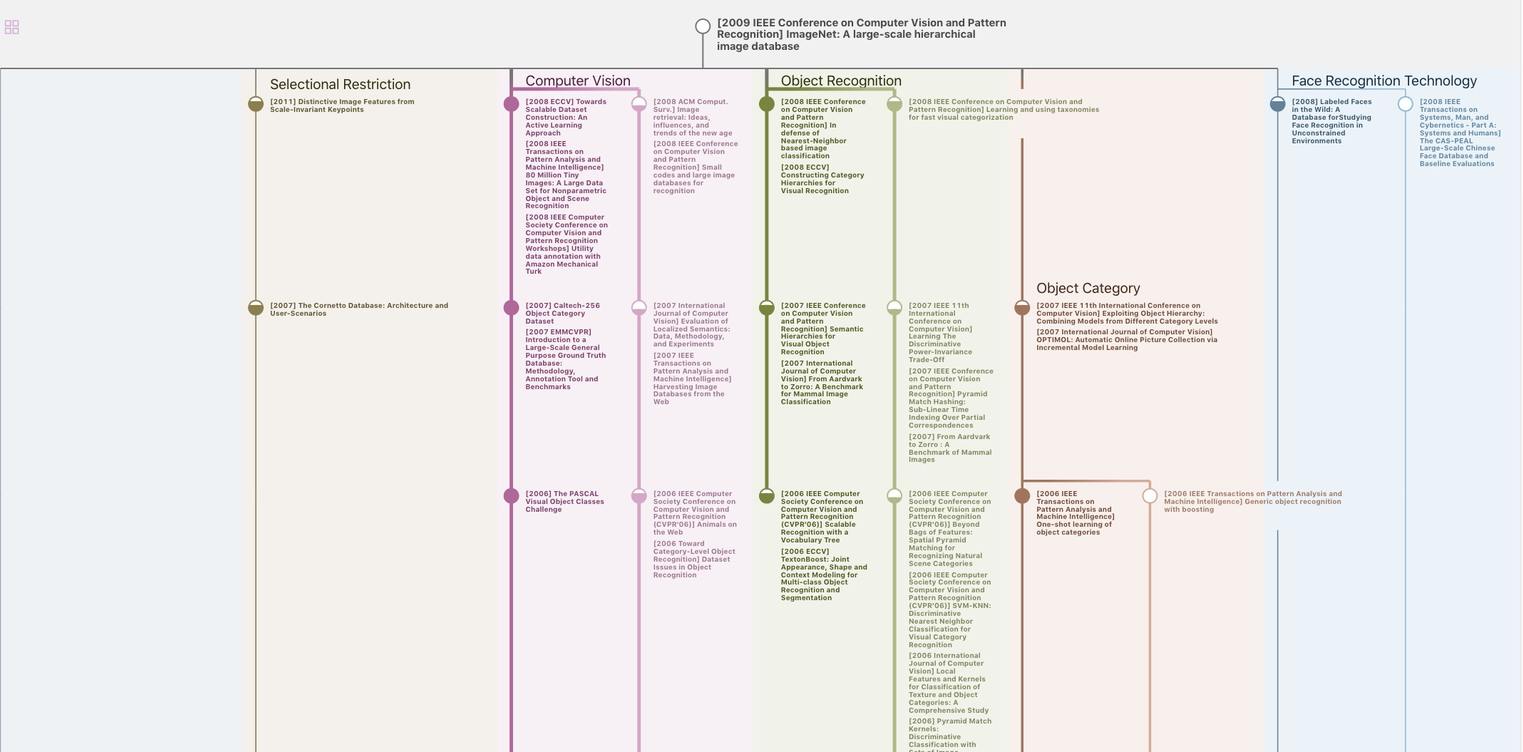
生成溯源树,研究论文发展脉络
Chat Paper
正在生成论文摘要