Principal Component Analysis and Related Methods for Investigating the Dynamics of Biological Macromolecules
J(2022)
摘要
Principal component analysis (PCA) is used to reduce the dimensionalities of high-dimensional datasets in a variety of research areas. For example, biological macromolecules, such as proteins, exhibit many degrees of freedom, allowing them to adopt intricate structures and exhibit complex functions by undergoing large conformational changes. Therefore, molecular simulations of and experiments on proteins generate a large number of structure variations in high-dimensional space. PCA and many PCA-related methods have been developed to extract key features from such structural data, and these approaches have been widely applied for over 30 years to elucidate macromolecular dynamics. This review mainly focuses on the methodological aspects of PCA and related methods and their applications for investigating protein dynamics.
更多查看译文
关键词
principal component analysis,collective variables,molecular dynamics,energy landscape,solvent effects,linear response theory
AI 理解论文
溯源树
样例
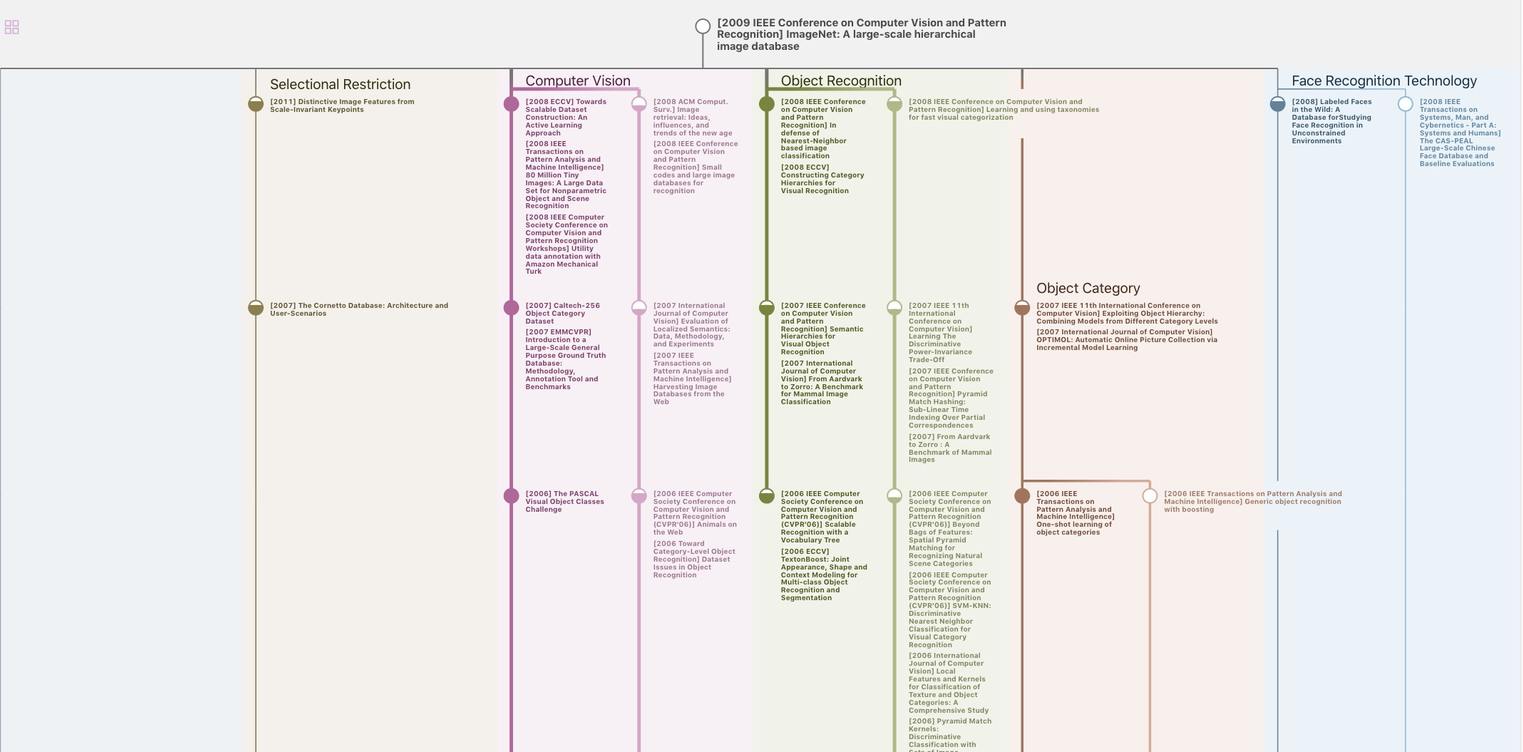
生成溯源树,研究论文发展脉络
Chat Paper
正在生成论文摘要