Learning for Optimization with Virtual Savant
Memoria Investigaciones en Ingeniería(2022)
摘要
Optimization problems arising in multiple fields of study demand efficient algorithms that can exploit modern parallel computing platforms. The remarkable development of machine learning offers an opportunity to incorporate learning into optimization algorithms to efficiently solve large and complex problems. This article explores Virtual Savant, a paradigm that combines machine learning and parallel computing to solve optimization problems. Virtual Savant is inspired in the Savant Syndrome, a mental condition where patients excel at a specific ability far above the average. In analogy to the Savant Syndrome, Virtual Savant extracts patterns from previously-solved instances to learn how to solve a given problem in a massively-parallel fashion. In this article, Virtual Savant is applied to three optimization problems related to software engineering, task scheduling, and public transportation. The efficacy of Virtual Savant is evaluated in different computing platforms and the experimental results are compared against exact and approximate solutions for both synthetic and realistic instances of the studied problems. Results show that Virtual Savant can find accurate solutions, effectively scale in the problem dimension, and take advantage of the availability of multiple computing resources.
更多查看译文
关键词
Machine learning,Optimization,Next release problem,Heterogeneous computing scheduling problem,Bus synchronization problem
AI 理解论文
溯源树
样例
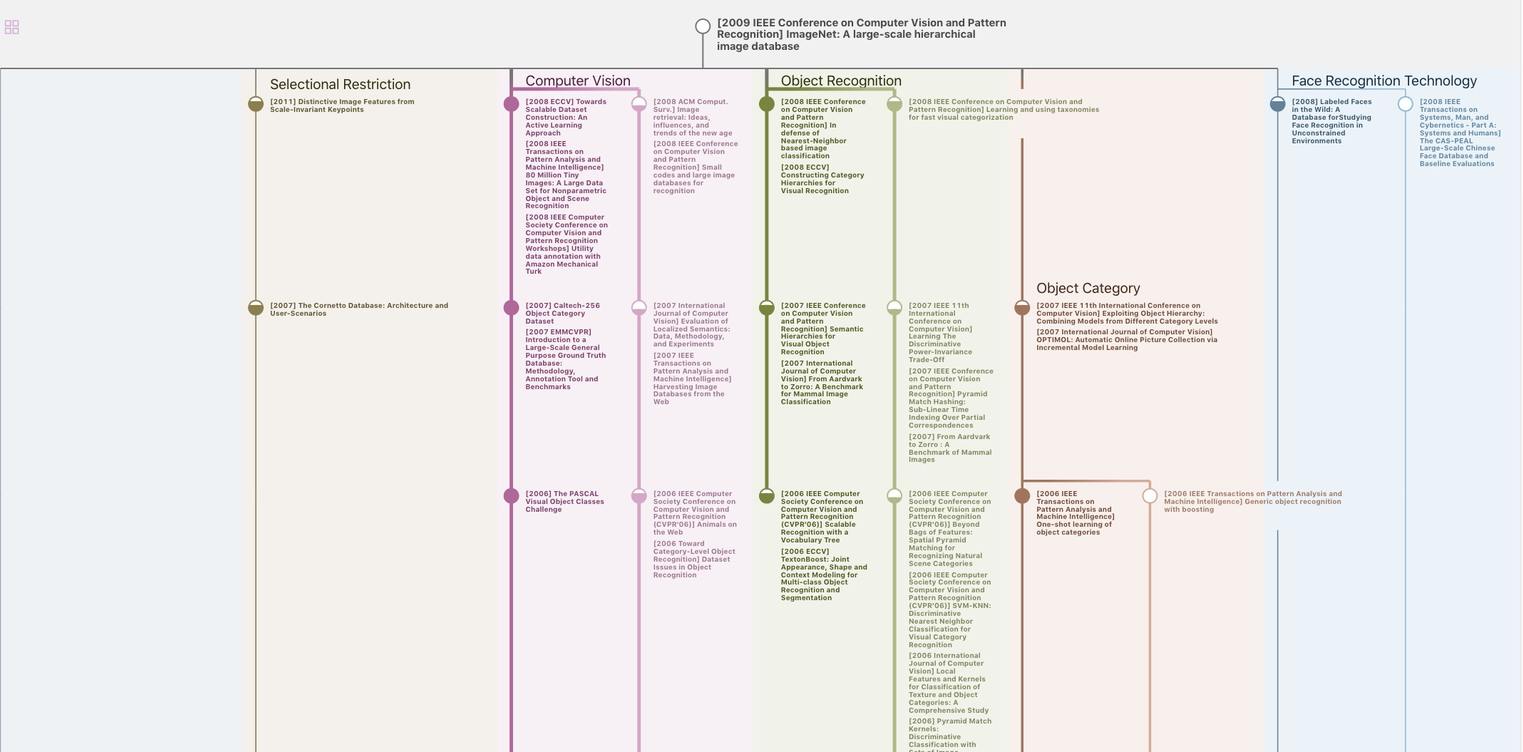
生成溯源树,研究论文发展脉络
Chat Paper
正在生成论文摘要