A variational local weighted deep sub-domain adaptation network for remaining useful life prediction facing cross-domain condition
Reliability Engineering & System Safety(2023)
摘要
Most supervised learning-based approaches follow the assumptions that offline data and online data must obey a similar distribution, which is difficult to satisfy in realistic remaining useful life (RUL) prediction. To solve the problem, domain adaptation (DA) learning-oriented transfer learning (TL) was proposed. Nevertheless, only adopting a conventional global DA approach may confuse the fine-grained features between subdomains represented by different degenerate stages. Consequently, a novel variational auto-encoder-long–short-term memory network-local weighted deep sub-domain adaptation network (VLSTM-LWSAN) is proposed for RUL prediction. Specifically, the input data are compressed into the interpretable latent space, from which the fine-grained features between subdomains are local alignment through local weighted deep sub-domain adaptation network. In this sense, the discrepancy between the unlabeled target domain and the source domain is decreased. The proposed VLSTM-LWSAN is verified by an aircraft turbofan engine dataset. The research results represent that the VLSTM-LWSAN outperforms some deep learning approaches without transfer learning and conventional transfer learning approaches.
更多查看译文
关键词
Remaining useful life,Transfer learning,Variational auto-encoder,Local weighted deep sub-domain adaptation,Prediction
AI 理解论文
溯源树
样例
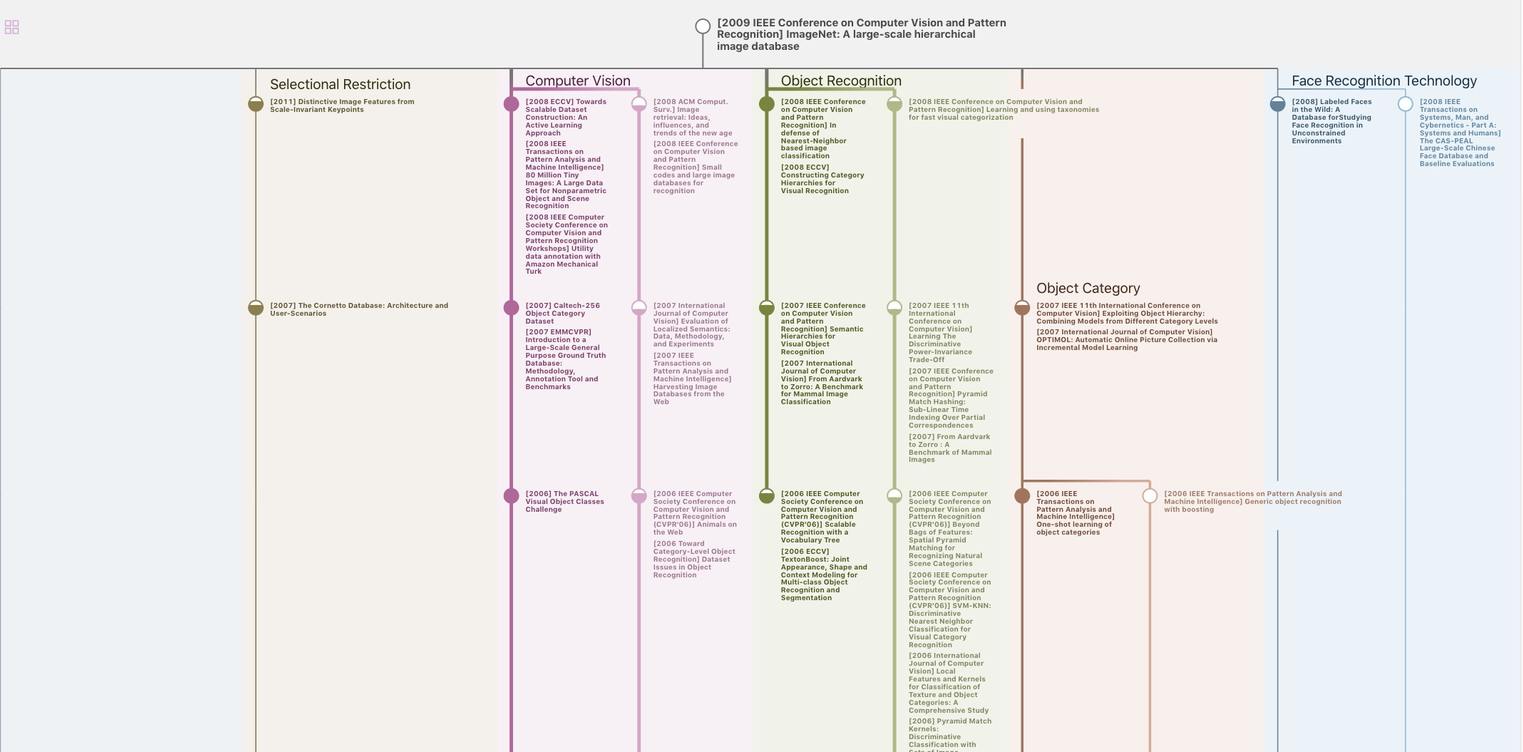
生成溯源树,研究论文发展脉络
Chat Paper
正在生成论文摘要