Multi-agent deep reinforcement learning for online request scheduling in edge cooperation networks
Future Generation Computer Systems(2023)
摘要
Edge computing as a complementary paradigm of cloud computing has gained more attention by providing mobile users with diversified services at the network edge. However, the increasingly complex mobile applications put a heavier load on edge networks. It is challenging to provide concurrency requests with high-quality service processing, especially when the edge networks are dynamically changing. To address the above issues, this paper investigates the online concurrent user requests scheduling optimization problem in edge cooperation networks. We model it as an online multi-stage decision-making problem, where requests are divided into a group of independent and logically related sub-tasks. We proposed a centralized training distributed execution based multi-agent deep reinforcement learning technique to realize the implicit cooperation scheduling decision-making policy learning among edge nodes. At the centralized training stage of the proposed mechanism, a value-decomposition-based policy learning technique is adopted to improve the long-term system performance, while at the distributed execution stage, only local environment status information is needed for each edge node to make the request scheduling decision. Extensive experiments are conducted, and simulation results demonstrate that the proposed mechanism outperforms other request scheduling mechanisms in reducing the long-term average system delay and energy consumption while improving the throughput rate of the system.
更多查看译文
关键词
Edge cooperation networks,Online request scheduling,Multi-agent system,Deep reinforcement learning,Value decomposition,Long-term performance
AI 理解论文
溯源树
样例
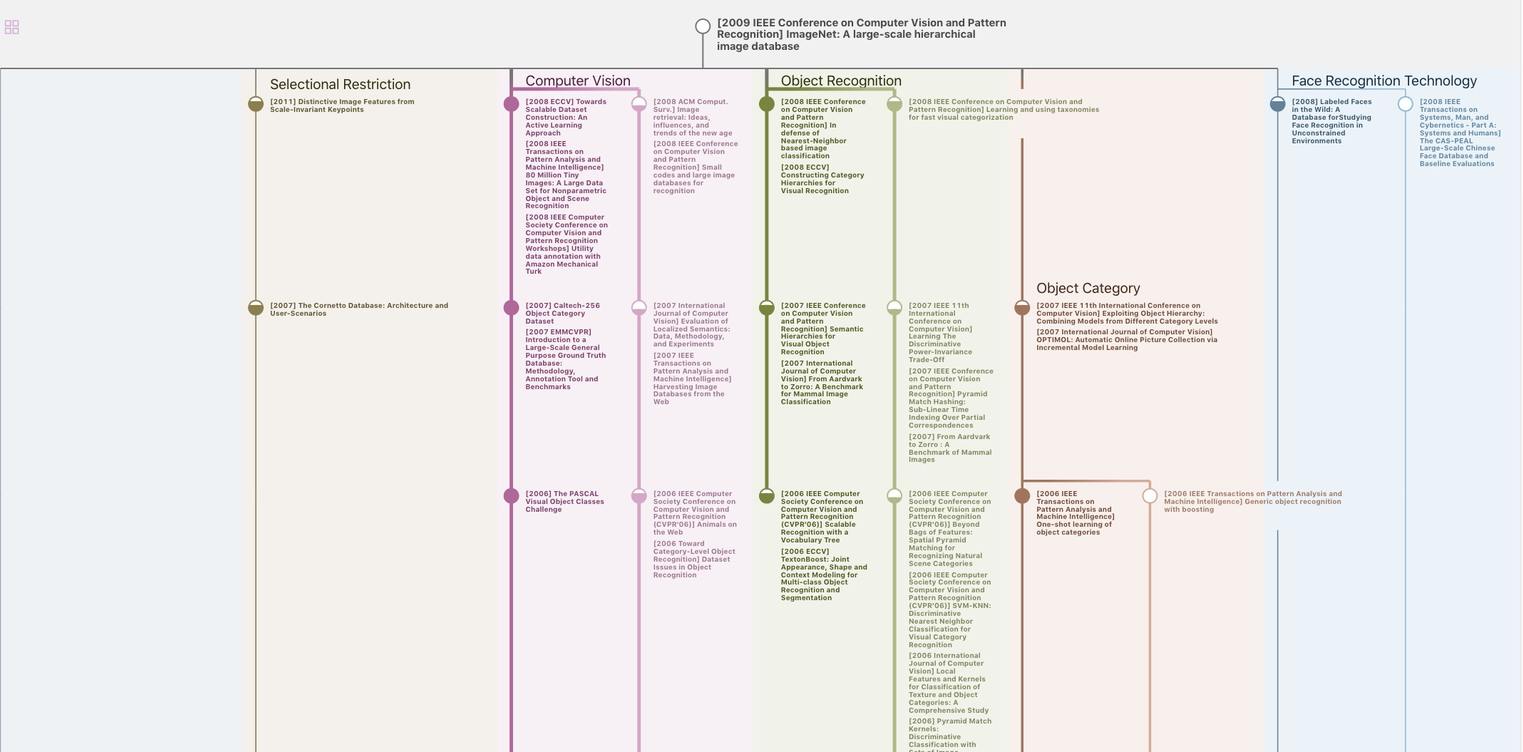
生成溯源树,研究论文发展脉络
Chat Paper
正在生成论文摘要