Combating medical noisy labels by disentangled distribution learning and consistency regularization
Future Generation Computer Systems(2023)
摘要
Machine learning, particularly the deep convolutional neural network (CNN), has significantly benefited computer-aided diagnosis technology to improve disease identification performance. Nevertheless, the shortage of training data with high-quality labels hinders training an effective and robust model. Medical image annotation is time-consuming and usually requires domain experts’ knowledge. Thus, most medical image datasets contain label noise including uncertainty and inconsistency. This paper proposes a hybrid hard-soft label learning mechanism and consistency regularization from two perspectives to enhance a single model’s disease detection capability instead of struggling with model ensembling. Specifically, a disentangled distribution learning integrates multiple reference models’ predictions and disentangles them into a majority confident label vector and a description degree score vector for co-training the single-target model, mitigating the negative influence caused by label noise. Furthermore, inter- and intra-instance consistency regularization aim to improve the target model’s robustness to give consistent predictions on images with similar lesion appearances. We conducted substantial experiments on the public chest X-ray and fundus image datasets as well as a computer vision benchmark, Clothing 1M, showing that our model surpasses the state-of-the-art methods with consistent improvements. The mean AUC on the CheXpert dataset is increased by 2.44%. The Q.W. Kappa on the KaggleDR+ and FGADR datasets, is increased by 5.65% and 4.66%, respectively. The classification accuracy on the Clothing 1M dataset is increased by 4.47%.
更多查看译文
关键词
Medical imaging,Label noise,Disentangled distribution learning,Consistency regularization
AI 理解论文
溯源树
样例
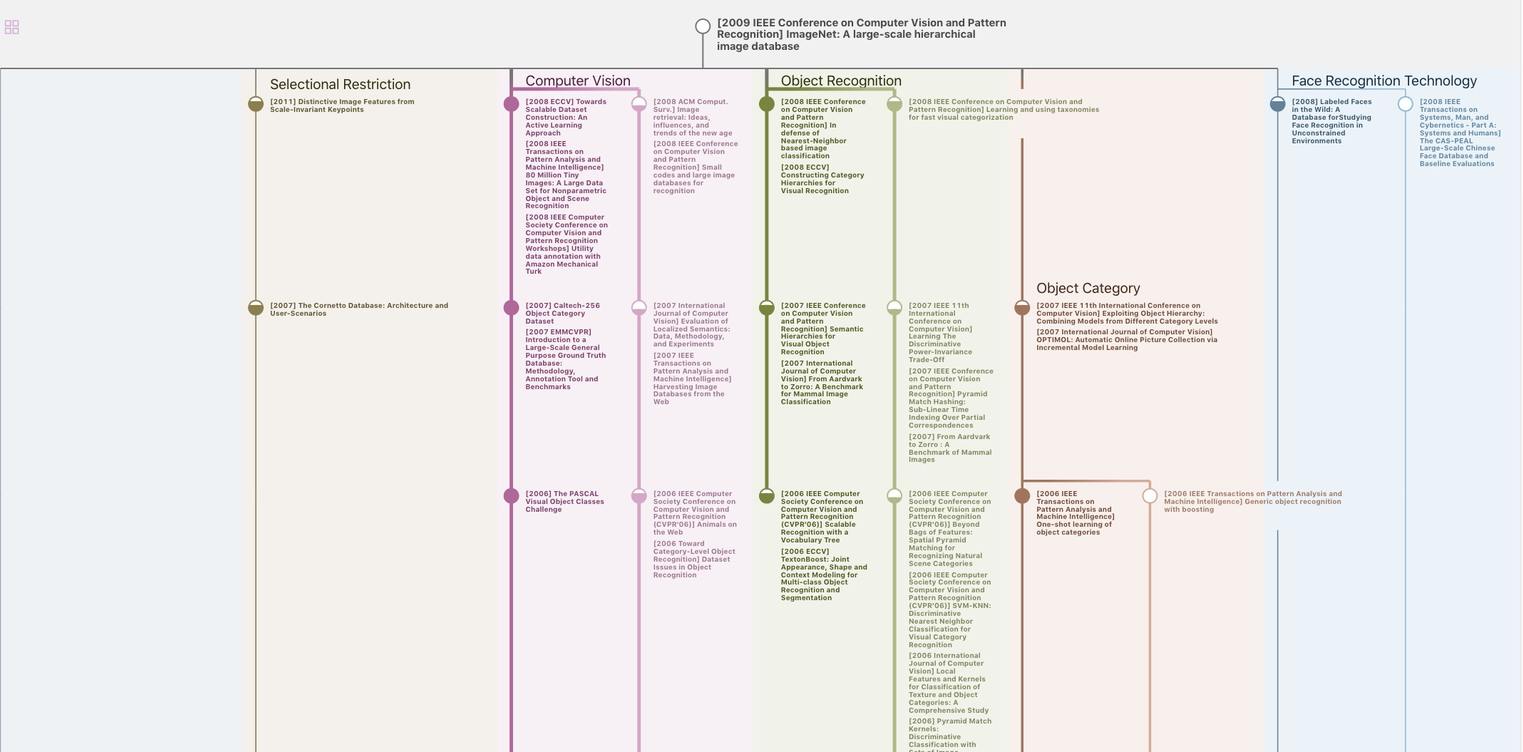
生成溯源树,研究论文发展脉络
Chat Paper
正在生成论文摘要