A full-level fused cross-task transfer learning method for building change detection using noise-robust pretrained networks on crowdsourced labels
Remote Sensing of Environment(2023)
摘要
Accurate building change detection is crucial for understanding urban development. Although fully supervised deep learning-based methods for building change detection have made progress, they tend to fuse temporal information only at a single level (e.g., input, feature, or decision levels) to mitigate the data distribution differences between time-series images, which is highly prone to introduce a large number of pseudo changes. Moreover, these methods rely on a large number of high-quality pixel-level change labels with high acquisition costs. In contrast, available crowdsourced building data are abundant but are less considered for change detection. For example, OpenStreetMap (OSM), Google Map, and Gaode Map provide lots of available building labels, yet they usually contain noise such as false alarms, omissions, and mismatches, limiting their wide application. In addition, when the building extraction task is transferred to the building change detection task, the temporal and regional differences between different images may lead to undesired pseudo changes. Given these issues, we propose a full-level fused cross-task transfer learning method for building change detection using only crowdsourced building labels and high-resolution satellite imagery. The method consists of three steps: 1) noise-robust building extraction network pretraining; 2) uncertainty-aware pseudo label generation; and 3) full-level fused building change detection. We created building extraction and building change detection datasets. The former (building extraction dataset) contains 30 scenes of ZY-3 images covering 27 major cities in China and crowdsourced building labels from Gaode Map for training, while the latter (building change dataset) contains bi-temporal ZY-3 images in Shanghai and Beijing for testing. The results show that the proposed method can identify changed buildings more effectively and better balance false alarms and omissions, compared to the existing state-of-the-art methods. Further analysis indicates that the inclusion of samples from multiple cities with various spatial heterogeneities is helpful to improve the performance. The experiments show that it is promising to apply the proposed method to situations where true labels are completely lacking or limited, thus alleviating the issue of high label acquisition cost. The source code will be available at https://github.com/lauraset/FFCTL.
更多查看译文
关键词
Building change detection,Transfer learning,Crowdsourced label,High-resolution remote sensing,Pseudo label
AI 理解论文
溯源树
样例
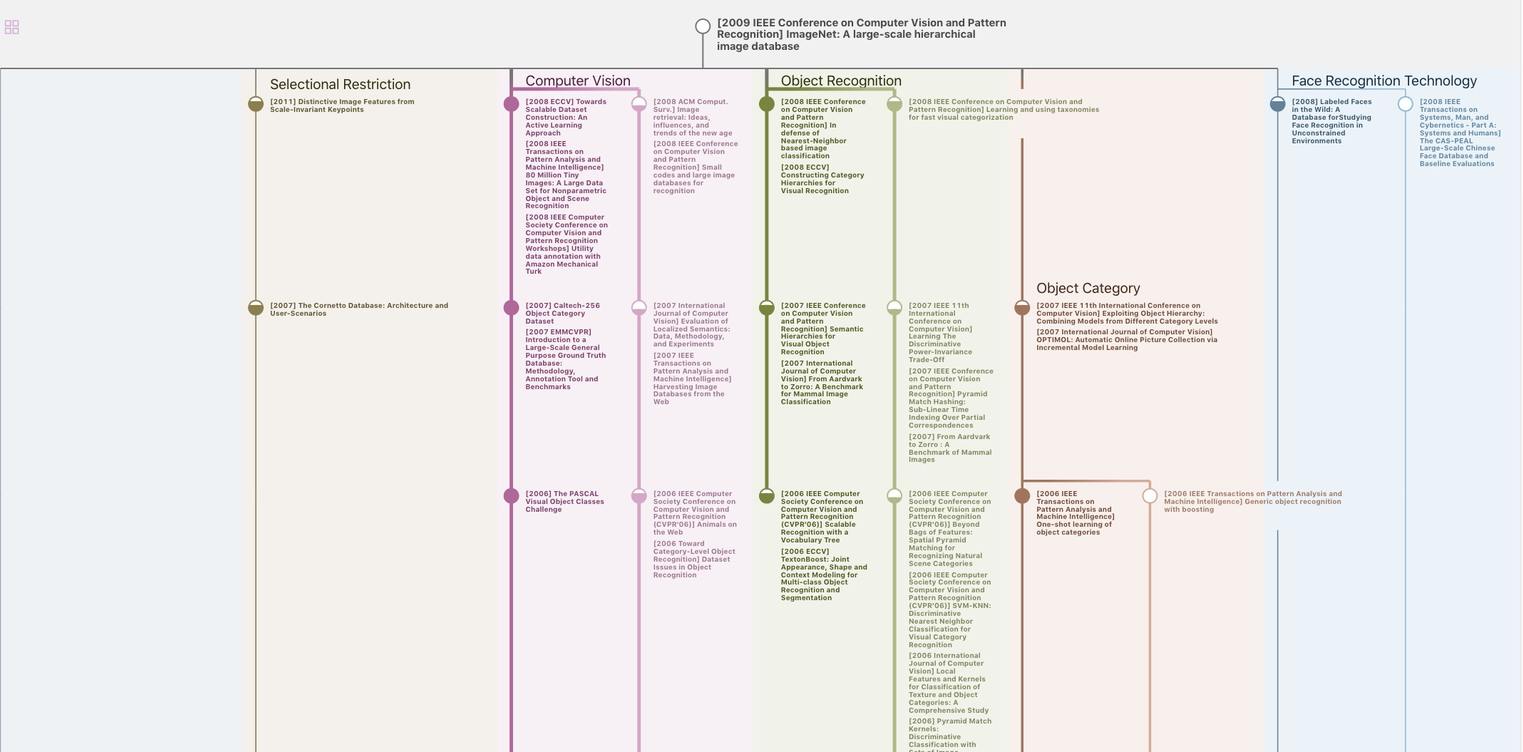
生成溯源树,研究论文发展脉络
Chat Paper
正在生成论文摘要