Dynamic Background Reconstruction via MAE for Infrared Small Target Detection
arXiv (Cornell University)(2023)
摘要
Infrared small target detection (ISTD) under complex backgrounds is a difficult problem, for the differences between targets and backgrounds are not easy to distinguish. Background reconstruction is one of the methods to deal with this problem. This paper proposes an ISTD method based on background reconstruction called Dynamic Background Reconstruction (DBR). DBR consists of three modules: a dynamic shift window module (DSW), a background reconstruction module (BR), and a detection head (DH). BR takes advantage of Vision Transformers in reconstructing missing patches and adopts a grid masking strategy with a masking ratio of 50\% to reconstruct clean backgrounds without targets. To avoid dividing one target into two neighboring patches, resulting in reconstructing failure, DSW is performed before input embedding. DSW calculates offsets, according to which infrared images dynamically shift. To reduce False Positive (FP) cases caused by regarding reconstruction errors as targets, DH utilizes a structure of densely connected Transformer to further improve the detection performance. Experimental results show that DBR achieves the best F1-score on the two ISTD datasets, MFIRST (64.10\%) and SIRST (75.01\%).
更多查看译文
关键词
dynamic background reconstruction,infrared small target
AI 理解论文
溯源树
样例
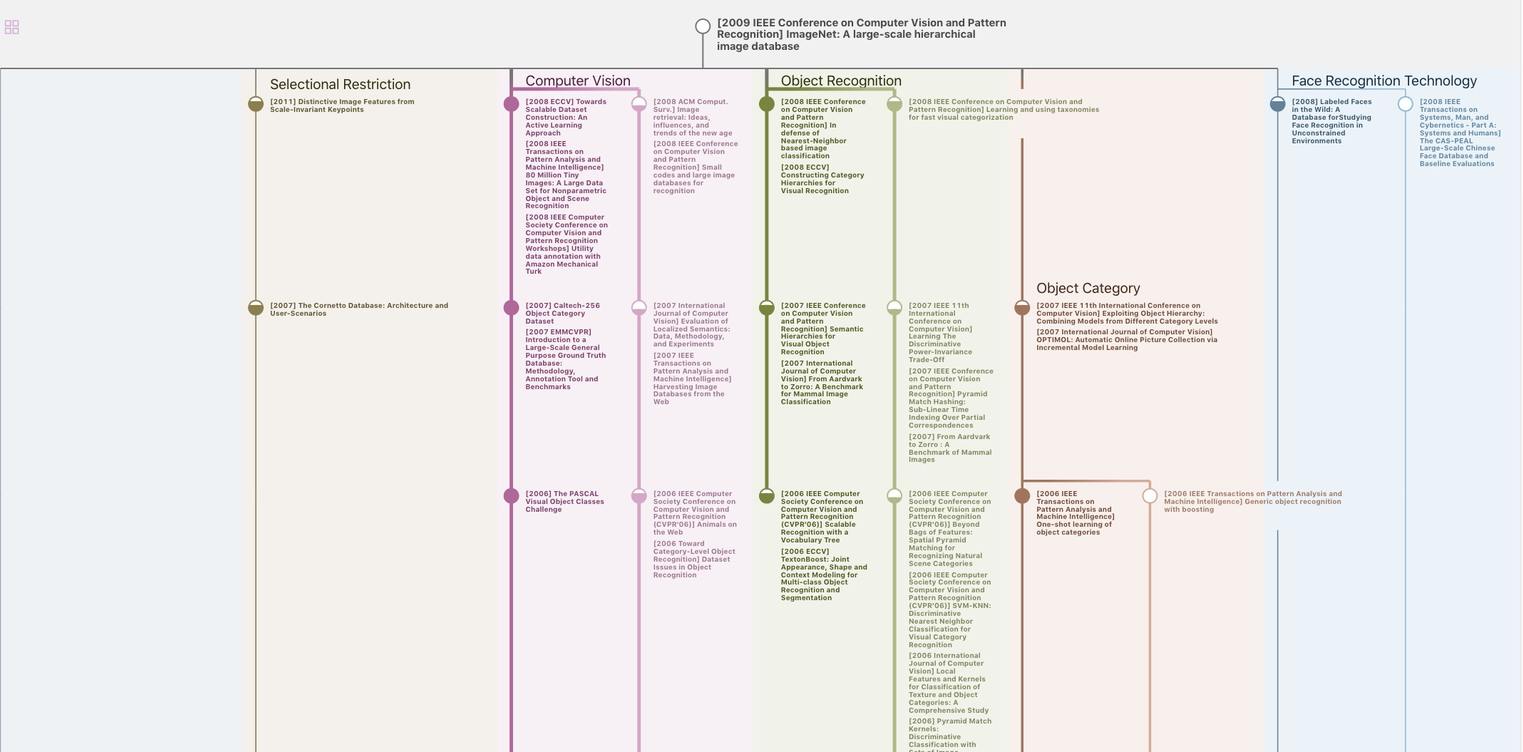
生成溯源树,研究论文发展脉络
Chat Paper
正在生成论文摘要